Deep Hashing With Weighted Spatial Importance
IEEE TRANSACTIONS ON MULTIMEDIA(2021)
摘要
Hashing method has been widely used in big data retrieval because of its low computational complexity. Most of existing hashing methods learn the final hash code from the semantic information of the whole image. However, different spatial regions of an image have different influences during the hash learning. To tackle this issue, we propose a new deep hashing with weighted spatial importance (DWSH) in this paper. Specifically, the proposed DWSH first utilizes a spatial attention model to learn the importance of different spatial regions in the original image, and then assigns different weights to these spatial regions according to their importance. The final hash codes are learned based on the weighted spatial information. In addition, two strategies are designed to utilize the spatial importance, including discrete weight strategy and continuous weight strategy, which weight the spatial information with discrete and continuous values, respectively. The results of extensive experiments conducted on three benchmark datasets show that the proposed DWSH method is superior to the state-of-the-art hashing method based on different evaluation protocols.
更多查看译文
关键词
Semantics, Hash functions, Feature extraction, Binary codes, Distributed databases, Deep learning, Data models, Hash, spatial importance, weight
AI 理解论文
溯源树
样例
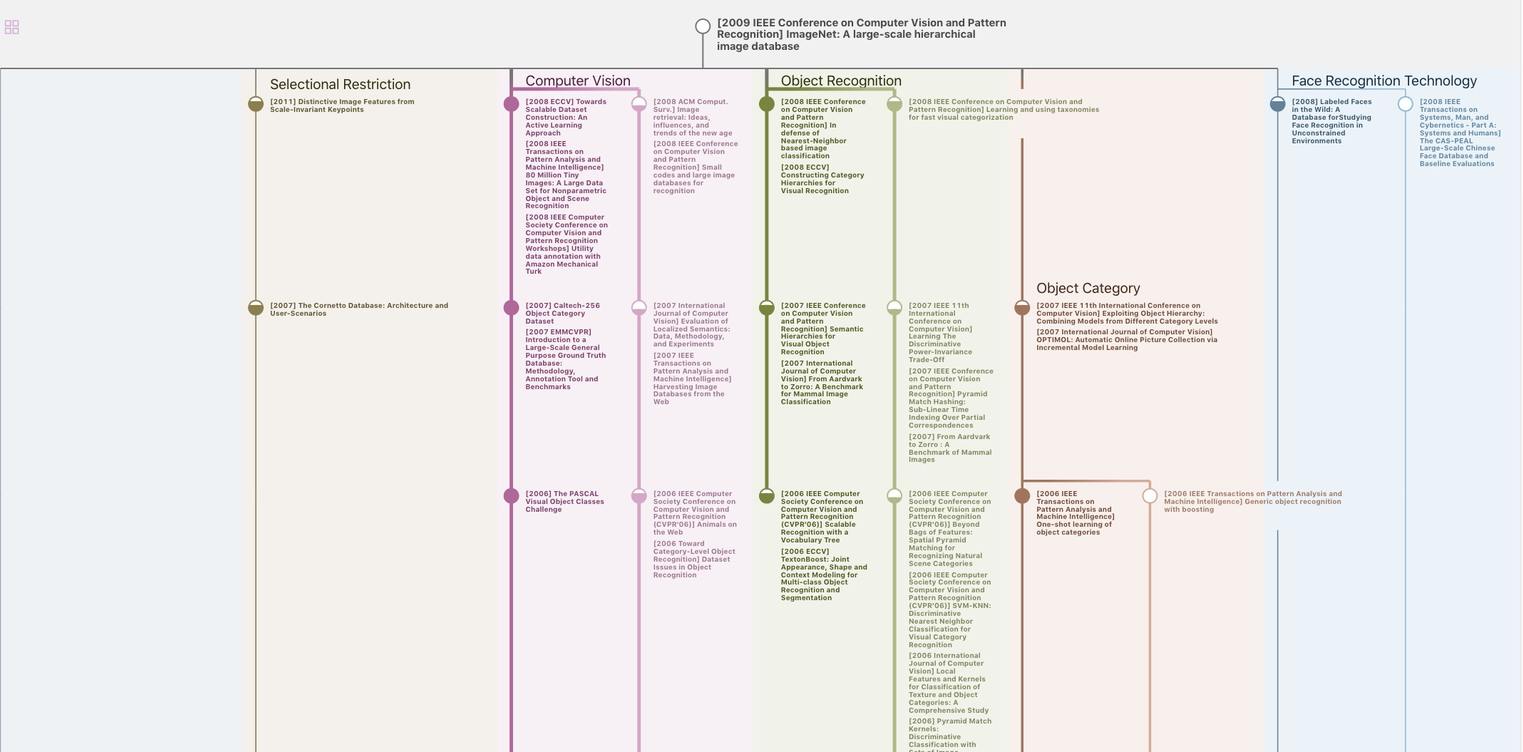
生成溯源树,研究论文发展脉络
Chat Paper
正在生成论文摘要