Enhanced-Pca Based Dimensionality Reduction And Feature Selection For Real-Time Network Threat Detection
ENGINEERING TECHNOLOGY & APPLIED SCIENCE RESEARCH(2020)
摘要
With the rise of the data amount being collected and exchanged over networks, the threat of cyber-attacks has also increased significantly. Timely and accurate detection of any intrusion activity in networks has become a crucial task. While manual moderation and programmed logic have been used for this purpose, the use of machine learning algorithms for superior pattern mapping is desired. The system logs in a network tend to include many parameters, and not all of them provide indications of an impending network threat. The selection of the right features is thus important for achieving better results. There is a need for accurate mapping of high dimension features to low dimension intermediate representations while retaining crucial information. In this paper, an approach for feature reduction and selection when working on network threat detection is proposed. This approach modifies the traditional Principal Component Analysis (PCA) algorithm by working on its shortcomings and by minimizing false detection rates. Specifically, work has been done upon the calculation of symmetric uncertainty and subsequent sorting of features. The performance of the proposed approach is evaluated on four standard-sized datasets that are collected using the Microsoft SYSMON real-time log collection tool. The proposed method is found to be better than the standard PCA and FAST methods for data reduction. The proposed approach makes a strong case as a dimensionality reduction and feature selection technique for reducing time complexity and minimizing false detection rates when operating on real-time data.
更多查看译文
关键词
principal component analysis, fast clustering, dimensionality reduction, machine learning, network security
AI 理解论文
溯源树
样例
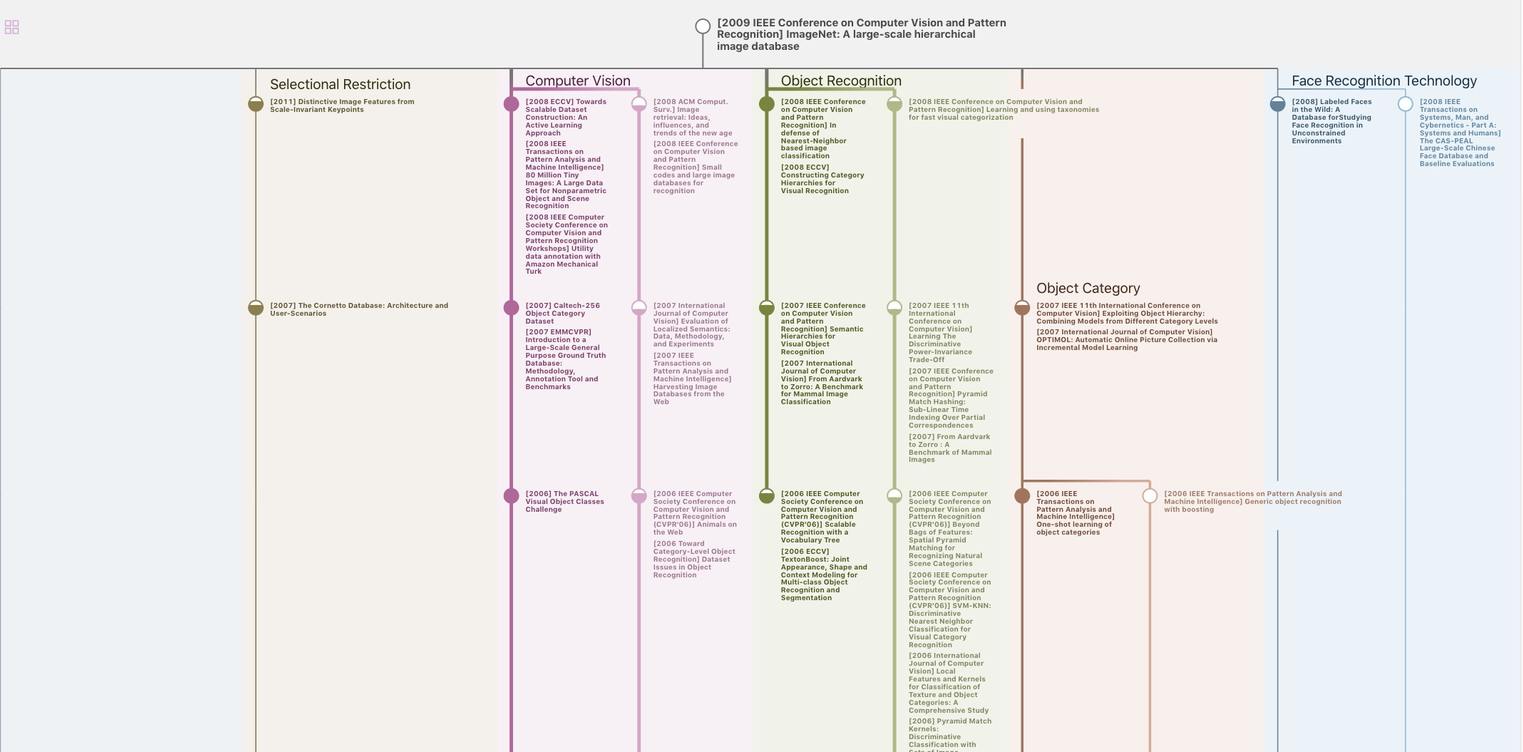
生成溯源树,研究论文发展脉络
Chat Paper
正在生成论文摘要