An Insight Into The Gibbs Sampler: Keep The Samples Or Drop Them?
IEEE SIGNAL PROCESSING LETTERS(2020)
摘要
In this letter, we propose an insight into Markov Chain Monte Carlo (MCMC) algorithms and more precisely the Gibbs sampler. From a didactic toy model, based on a normal bivariate distribution, a non-asymptotic analysis is derived and estimators are fully characterized. It provides a worthwhile and non-empirical understanding of the Gibbs sampler behaviour. Issues are investigated, such as the influence of the "burn-in" phase, useful in practice. Especially, the trade-off between discarding samples and integrating them into estimators is studied. On the whole, it leads to an analytical awareness of MCMC sampler.
更多查看译文
关键词
Bayesian statistics, burn-in, gibbs, MCMC
AI 理解论文
溯源树
样例
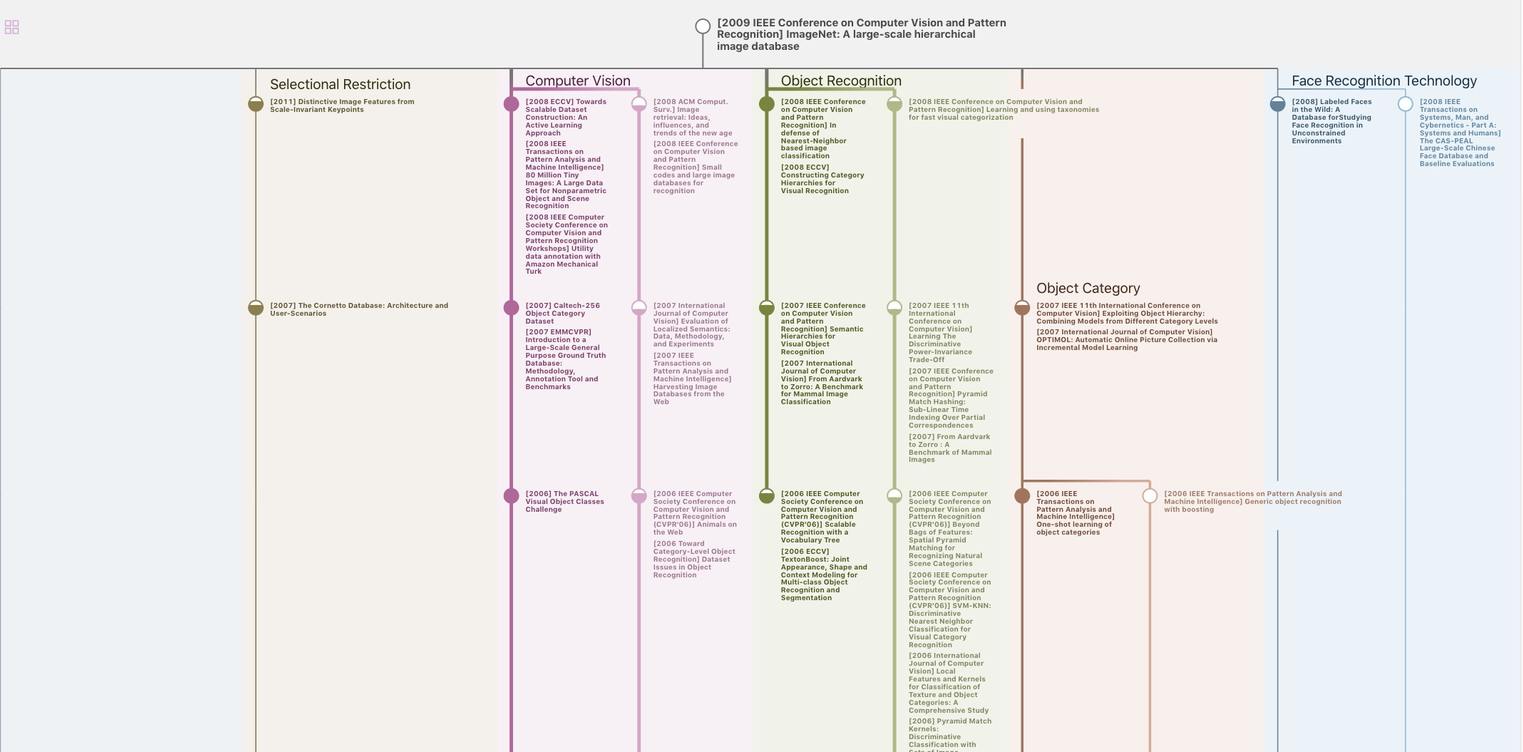
生成溯源树,研究论文发展脉络
Chat Paper
正在生成论文摘要