Low-rank adaptive graph embedding for unsupervised feature extraction
Pattern Recognition(2021)
摘要
•We propose a novel unsupervised feature extraction method named LRAGE, which can learn informative projection and a meaningful adaptive graph with probabilistic weight jointly based on reconstruction error minimization.•We impose a low- rank constraint on the model to learn more informative projection. The potential properties of LRAGE is well revealed by comparing it with several similar models on both synthetic and real-world data sets.•An iterative algorithm is elaborately designed to solve the resulting optimization problem. The convergence of the proposed algorithm is proved and the corresponding computational complexity analysis is also presented. Extensive experiments indicate the promising performance of the proposed LRAGE, especially on the corrupted data sets.•Extensive experiments indicate the promising performance of the proposed LRAGE, especially on the corrupted data sets.
更多查看译文
关键词
Low-rank regression,Jointly sparse learning,Adaptive graph embedding,Unsupervised feature extraction
AI 理解论文
溯源树
样例
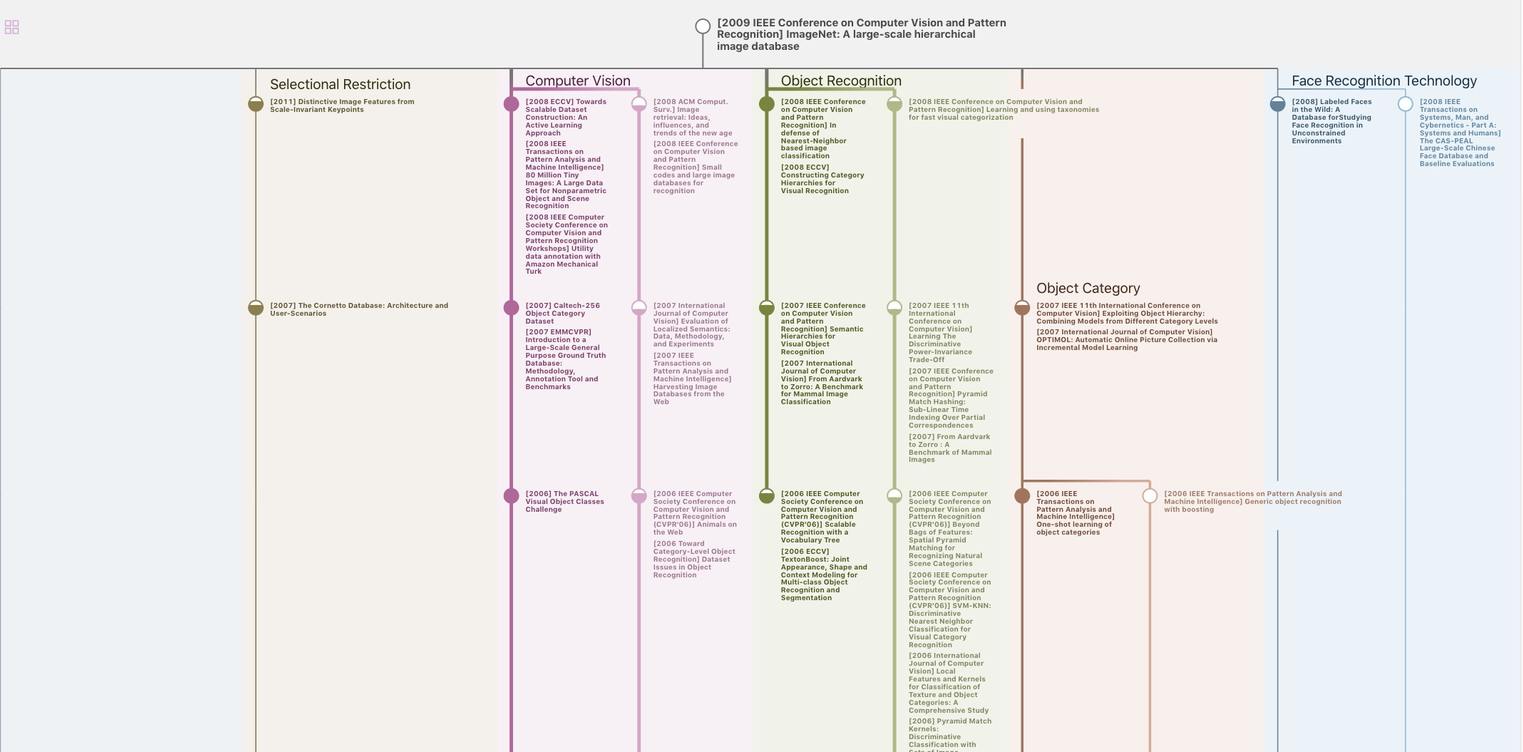
生成溯源树,研究论文发展脉络
Chat Paper
正在生成论文摘要