Two-Step Thresholds Tbd Algorithm For Time Sensitive Target Based On Dynamic Programming
IEEE ACCESS(2020)
摘要
Surveillance radar is confronted with serious two-dimensional ambiguity (i.e., range dimension and velocity dimension) and low signal-to-noise ratio (SNR) problems in the time sensitive (TS) target detection process, which may result in the deterioration of target detection performance. Combining the advantages of the intra-frame detection and the inter-frame detection, a novel two-step thresholds track before detect (TBD) algorithm based on the integration techniques, i.e., two-dimensional ambiguity resolution and multi-frame joint detection, is proposed in this paper. Firstly, the fusion design strategy, including the pulse repetition frequency, the carrier frequency and the orthogonal waveform, is adopted in the transmitting pulse sequence. As a result, not only the range ambiguity number and the spatial filling time are reduced synchronously, but the decoupling of range-velocity ambiguity is realized, which is conductive to multi-target detection in low SNR environment. Then, the first level adaptive threshold is obtained by employing the judgment criterion based on cumulative value function (CVF), and thus the false trajectory number is effectively eliminated from the perspective of cumulative amplitude. Finally, through analyzing the inherent relation between the local trajectory characteristics and the global trajectory characteristics comprehensively, different types of false alarm trajectories are further reduced by means of the polynomial coefficient variance statistics. Experimental results verify the effectiveness of the proposed algorithm.
更多查看译文
关键词
Trajectory,Signal to noise ratio,Heuristic algorithms,Signal resolution,Radar cross-sections,Mathematical model,Correlation,Pulse sequence,track before detect,two-dimensional ambiguity,cumulative value function,trajectory characteristics
AI 理解论文
溯源树
样例
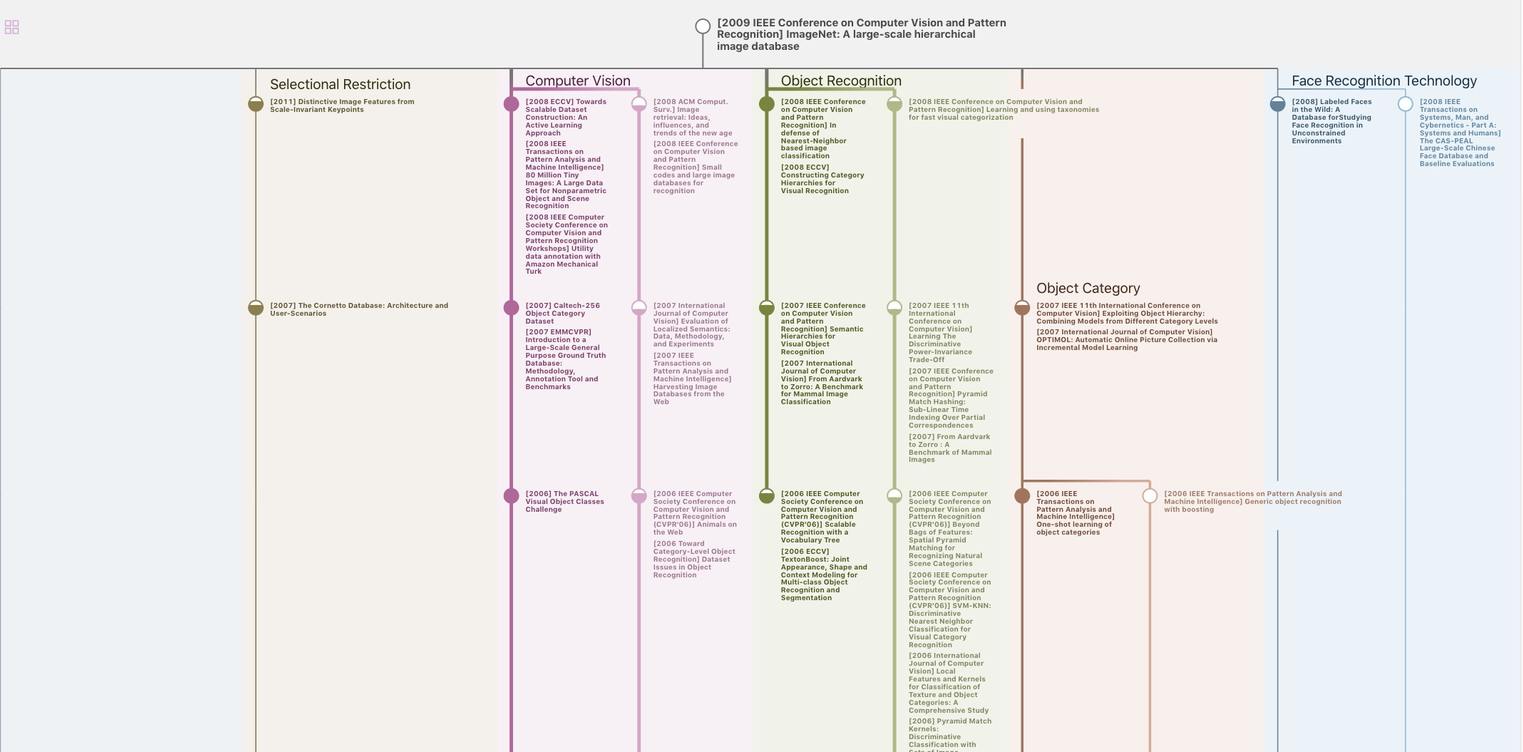
生成溯源树,研究论文发展脉络
Chat Paper
正在生成论文摘要