Meta-MSNet: Meta-Learning Based Multi-Source Data Fusion for Traffic Flow Prediction
IEEE Signal Processing Letters(2021)
摘要
Traffic flow prediction is a challenging task while most existing works are faced with two main problems in extracting complicated intrinsic and extrinsic features. In terms of intrinsic features, current methods don't fully exploit different functions of short-term neighboring and long-term periodic temporal patterns. As for extrinsic features, recent works mainly employ hand-crafted fusion strategies to integrate external factors but remain generalization issues. To solve these problems, we propose a meta-learning based multi-source spatio-temporal network (Meta-MSNet). The Meta-MSNet is designed with an encoder-decoder structure. The encoder captures neighboring temporal dependencies while the decoder extracts periodic features. Furthermore, two meta-learning based fusion modules are designed to integrate multi-source external data both on temporal and spatial dimensions. Experiments on three real-world traffic datasets have verified the superiority of the proposed model.
更多查看译文
关键词
Data fusion,deep learning,graph convolution,meta-learning,traffic flow prediction,traffic network
AI 理解论文
溯源树
样例
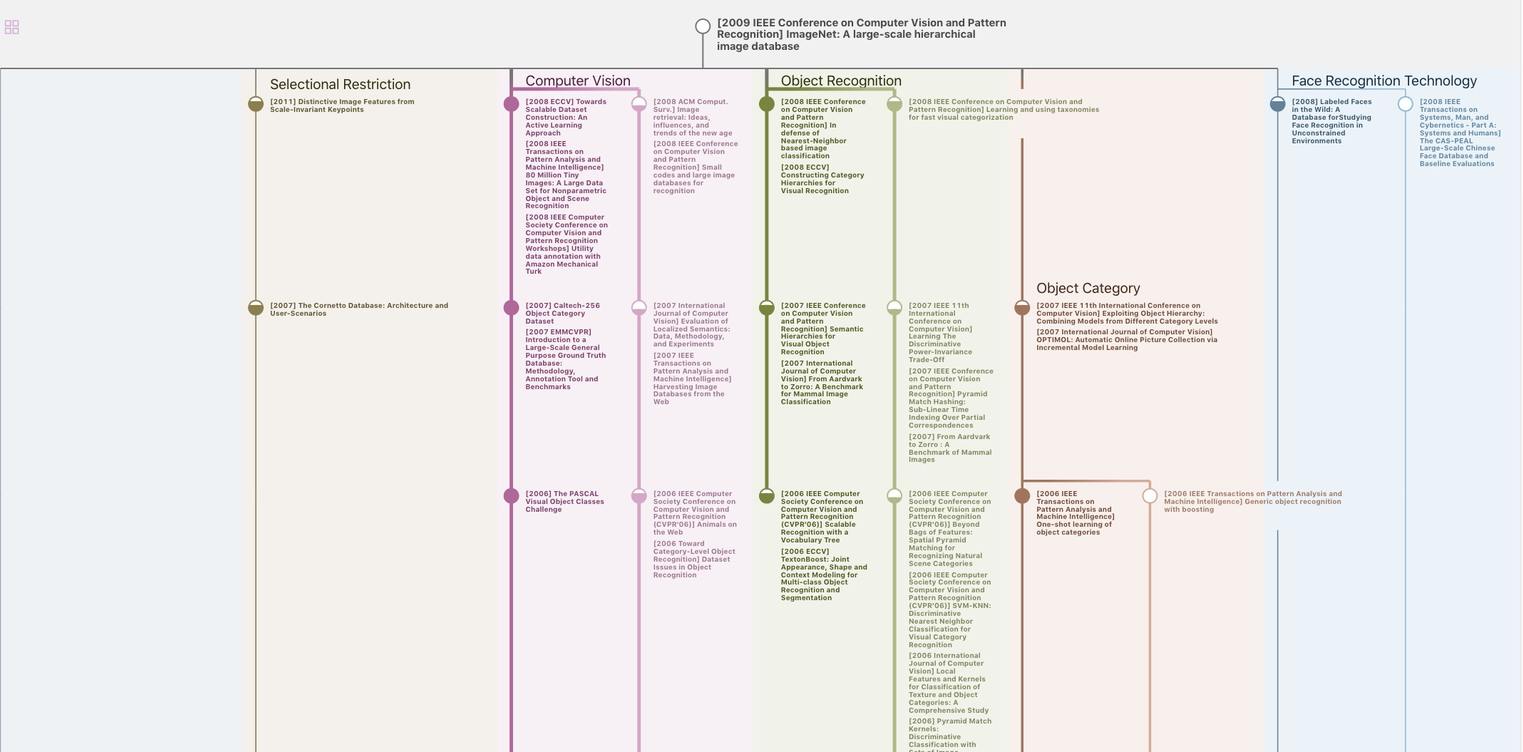
生成溯源树,研究论文发展脉络
Chat Paper
正在生成论文摘要