Jointly Learning Visual Motion and Confidence from Local Patches in Event Cameras
European Conference on Computer Vision(2020)
摘要
We propose the first network to jointly learn visual motion and confidence from events in spatially local patches. Event-based sensors deliver high temporal resolution motion information in a sparse, non-redundant format. This creates the potential for low computation, low latency motion recognition. Neural networks which extract global motion information, however, are generally computationally expensive. Here, we introduce a novel shallow and compact neural architecture and learning approach to capture reliable visual motion information along with the corresponding confidence of inference. Our network makes a prediction of the visual motion at each spatial location using only local events. Our confidence network then identifies which of these predictions will be accurate. In the task of recovering pan-tilt ego velocities from events, we show that each individual confident local prediction of our network can be expected to be as accurate as state of the art optimization approaches which utilize the full image. Furthermore, on a publicly available dataset, we find our local predictions generalize to scenes with camera motions and the presence of independently moving objects. This makes the output of our network well suited for motion based tasks, such as the segmentation of independently moving objects. We demonstrate on a publicly available motion segmentation dataset that restricting predictions to confident regions is sufficient to achieve results that exceed state of the art methods.
更多查看译文
关键词
visual motion,event cameras,learning,confidence
AI 理解论文
溯源树
样例
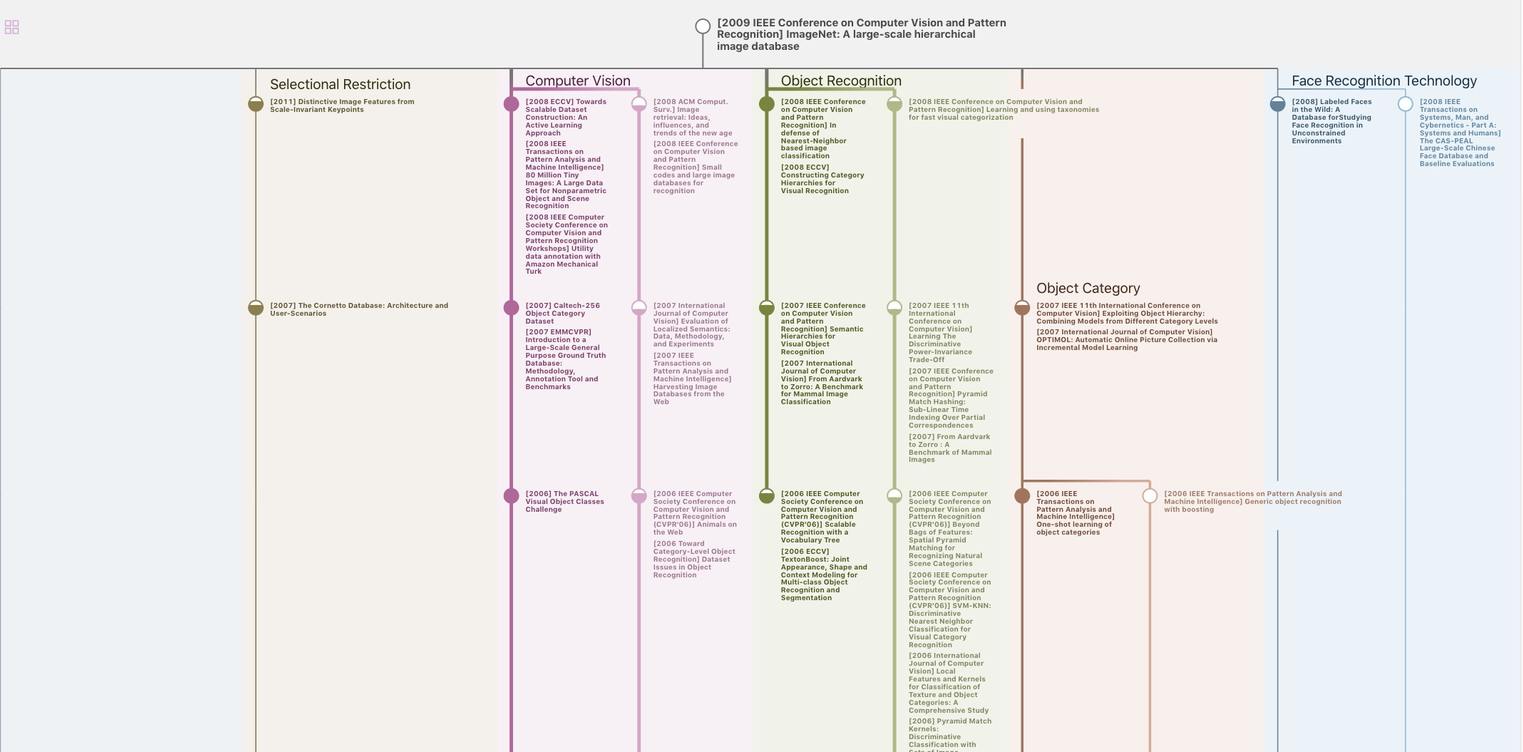
生成溯源树,研究论文发展脉络
Chat Paper
正在生成论文摘要