The detection, tracking, and temporal action localisation of swimmers for automated analysis
NEURAL COMPUTING & APPLICATIONS(2020)
摘要
It is very important for swimming coaches to analyse a swimmer’s performance at the end of each race, since the analysis can then be used to change strategies for the next round. Coaches rely heavily on statistics, such as stroke length and instantaneous velocity, when analysing performance. These statistics are usually derived from time-consuming manual video annotations. To automatically obtain the required statistics from swimming videos, we need to solve the following four challenging computer vision tasks: swimmer head detection; tracking; stroke detection; and camera calibration. We collectively solve these problems using a two-phased deep learning approach, we call Deep Detector for Actions and Swimmer Heads (DeepDASH). DeepDASH achieves a 20.8% higher F1 score for swimmer head detection and operates 6 times faster than the popular Faster R-CNN object detector. We also propose a hierarchical tracking algorithm based on the existing SORT algorithm which we call HISORT. HISORT produces significantly longer tracks than SORT by preserving swimmer identities for longer periods of time. Finally, DeepDASH achieves an overall F1 score of 97.5% for stroke detection across all four swimming stroke styles.
更多查看译文
关键词
Object detection, Tracking, Temporal action recognition, Deep learning, Convolutional neural networks
AI 理解论文
溯源树
样例
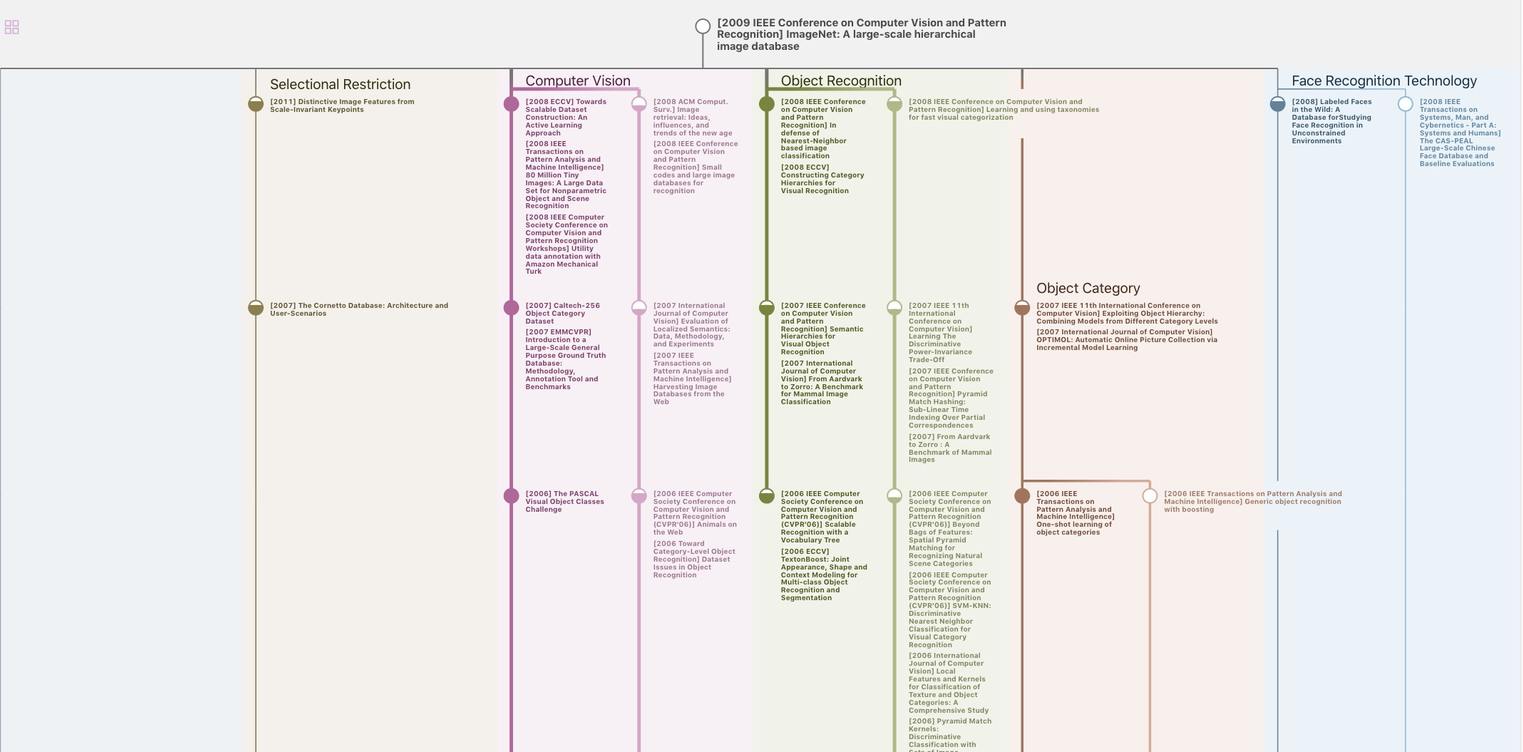
生成溯源树,研究论文发展脉络
Chat Paper
正在生成论文摘要