Predictability of Monthly Streamflow Time Series and its Relationship with Basin Characteristics: an Empirical Study Based on the MOPEX Basins
WATER RESOURCES MANAGEMENT(2020)
摘要
Machine learning (ML) models have been applied to monthly streamflow forecasting in recent decades. In this study, forecasting skills of eight ML models are evaluated based on the Model Parameter Estimation Experiment (MOPEX) dataset. We consider two skill scores, i.e., the Nash–Sutcliffe efficiency (NSE) and the adjusted NSE (ANSE), and the latter is the skill score based on the interannual mean monthly value (MMV) as the reference (benchmark) model. Furthermore, NSE of the MMV model (NSE mmv ) is used as a measure of the seasonality of monthly streamflow, as it is the ratio of variance explained by the MMV process. An important result is that forecasting skills of ML models for monthly streamflow are largely controlled by NSE mmv . Moreover, based on comparisons of different ML models, we have found that the selection of models is not a dominating factor impacting the final skill. Three key factors influencing NSE, i.e., NSE mmv , the base flow index (BFI) and the aridity index (AI), are explored in this paper. Specifically, NSE mmv impacts NSE directly and is the predominant factor; BFI influences the memory of the monthly streamflow and therefore influences NSE. The relationship between AI and NSE is much complex and indirect. Firstly, basins with higher AI tend to have lower NSE mmv , and this will lead to lower NSE; secondly, basins with higher AI tend to have lower BFI, which will also lead to lower NSE; thirdly, for a given BFI level, basins with higher AI tend to have higher memory and higher NSE. For ANSE, basins with AI between 1 and 2 show higher ANSE, which corresponds to higher autocorrelation coefficients.
更多查看译文
关键词
Monthly streamflow forecasting,Machine learning,Aridity index,Streamflow seasonality,Predictability
AI 理解论文
溯源树
样例
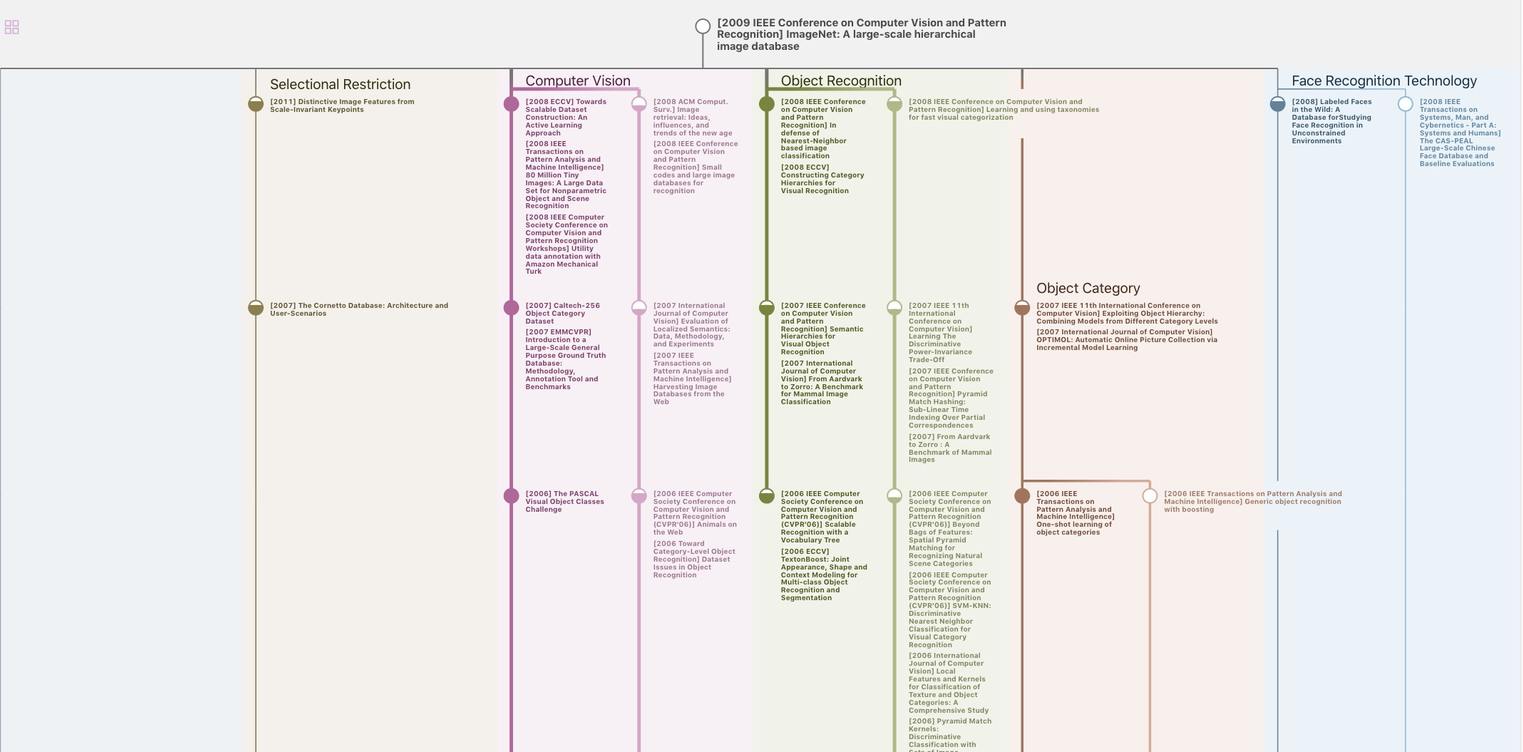
生成溯源树,研究论文发展脉络
Chat Paper
正在生成论文摘要