Multi-scale skip-connection network for image super-resolution
MULTIMEDIA SYSTEMS(2020)
摘要
A skip-connection learning framework-based convolution neural network (CNN) has recently achieved great success in image super-resolution (SR). However, most CNN models based on the skip-connection learning framework do not fully make use of potential multi-scale features of images. In this paper, we propose a multi-scale skip-connection network (MSN) to improve the visual quality of the image SR. First, convolution kernels with different sizes are exploited to capture the multi-scale features of LR images. All the feature-maps captured by convolution kernels of the same size are direct input into a multi-scale hybrid group (MHG); second, the convolution layers of each MHG are composed of dilated convolutions and standard convolutions. The hybrid convolutions can fully train feature details obtained from preceding and current scale convolution layers; three, the output of each hybrid convolution layer is fed into subsequent hybrid convolution layers by skip-connections, thus producing dense connections; lastly, the meta-upscale module is used as the upscale module, which can magnify the trained feature maps arbitrary scale factors. By being evaluated on a wide variety of images, the proposed MSN network achieves an advantage over the state-of-the-art methods in terms of both numerical results and visual quality.
更多查看译文
关键词
Super-resolution image,Skip connection,Convolution neural network,Multi-scale feature,Hybrid convolution
AI 理解论文
溯源树
样例
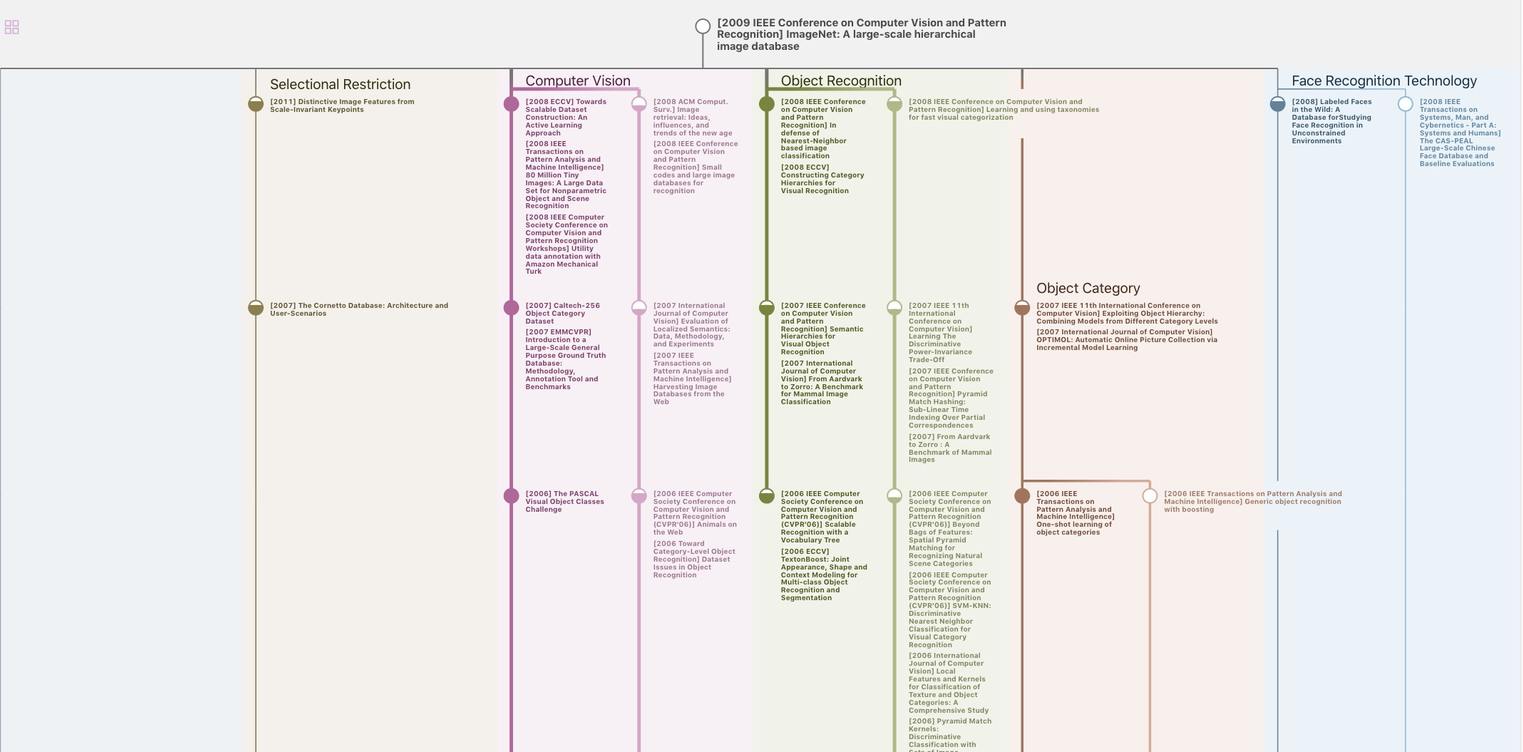
生成溯源树,研究论文发展脉络
Chat Paper
正在生成论文摘要