Adaptive Average Exploration In Multi-Agent Reinforcement Learning
2020 AIAA/IEEE 39TH DIGITAL AVIONICS SYSTEMS CONFERENCE (DASC) PROCEEDINGS(2020)
摘要
The objective of this research project was to improve Multi-Agent Reinforcement Learning performance in the StarCraft II environment with respect to faster training times, greater stability, and higher win ratios by 1) creating an adaptive action selector we call Adaptive Average Exploration, 2) using experiences previously learned by a neural network via Transfer Learning, and 3) updating the network simultaneously with its random action selector epsilon. We describe how agents interact with the StarCraft II environment and the QMIX algorithm used to test our approaches. We compare our AAE action selection approach with the default epsilon greedy method used by QMIX. These approaches are used to train Transfer Learning (TL) agents under a variety of test cases. We evaluate our TL agents using a predefined set of metrics. Finally, we demonstrate the effects of updating the neural networks and epsilon together more frequently on network performance.
更多查看译文
关键词
multi-agent reinforcement learning, exploration and exploitation, micromanagement, StarCraft II
AI 理解论文
溯源树
样例
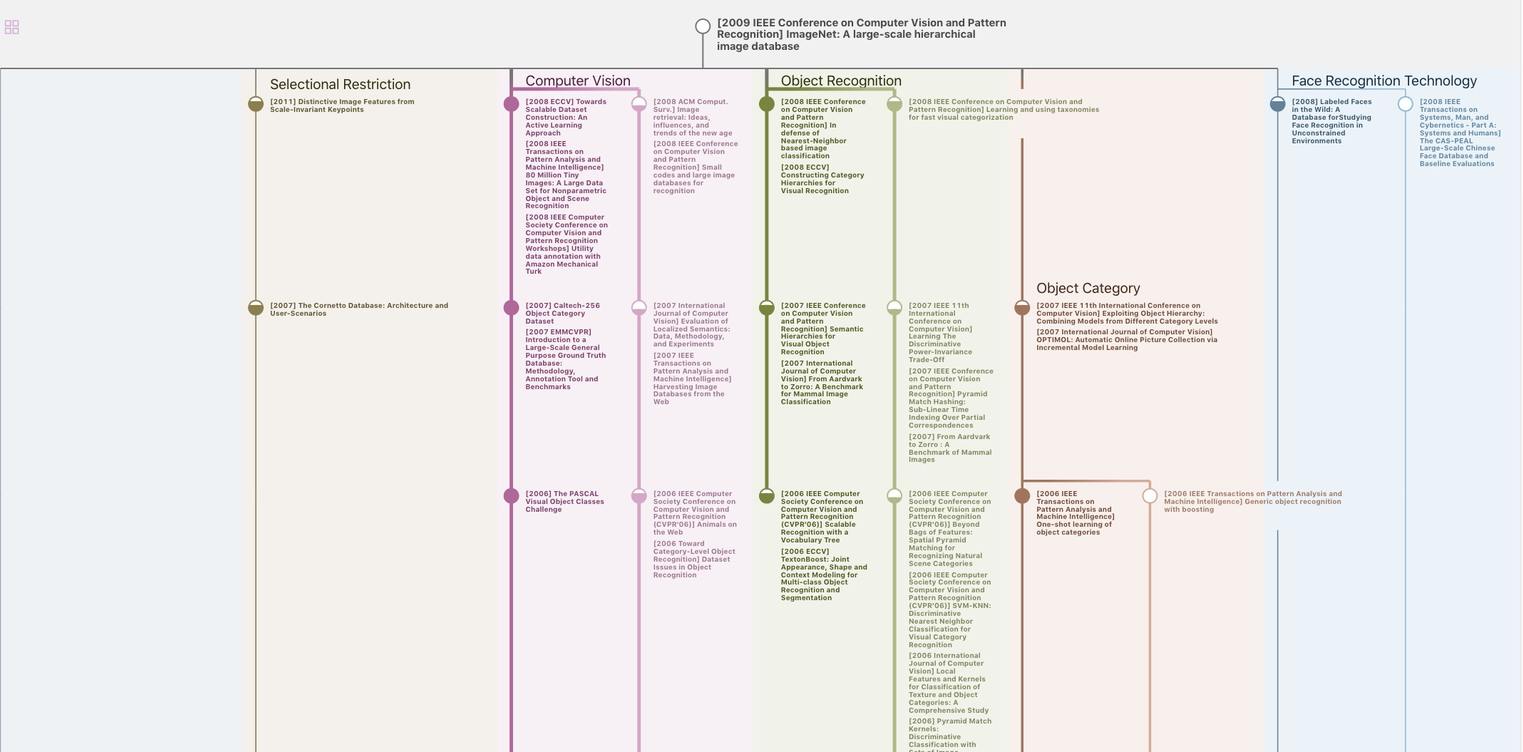
生成溯源树,研究论文发展脉络
Chat Paper
正在生成论文摘要