Deep Learning for Understanding Satellite Imagery: An Experimental Survey
Frontiers in artificial intelligence(2020)
摘要
Translating satellite imagery into maps requires intensive effort and time, especially leading to inaccurate maps of the affected regions during disaster and conflict. The combination of availability of recent datasets and advances in computer vision made through deep learning paved the way toward automated satellite image translation. To facilitate research in this direction, we introduce the Satellite Imagery Competition using a modified SpaceNet dataset. Participants had to come up with different segmentation models to detect positions of buildings on satellite images. In this work, we present five approaches based on improvements of U-Net and Mask R-Convolutional Neuronal Networks models, coupled with unique training adaptations using boosting algorithms, morphological filter, Conditional Random Fields and custom losses. The good results-as high as AP = 0.937 and AR = 0.959-from these models demonstrate the feasibility of Deep Learning in automated satellite image annotation.
更多查看译文
关键词
deep learning,machine learning,remote sensing,satellite imagery,semantic segmentation
AI 理解论文
溯源树
样例
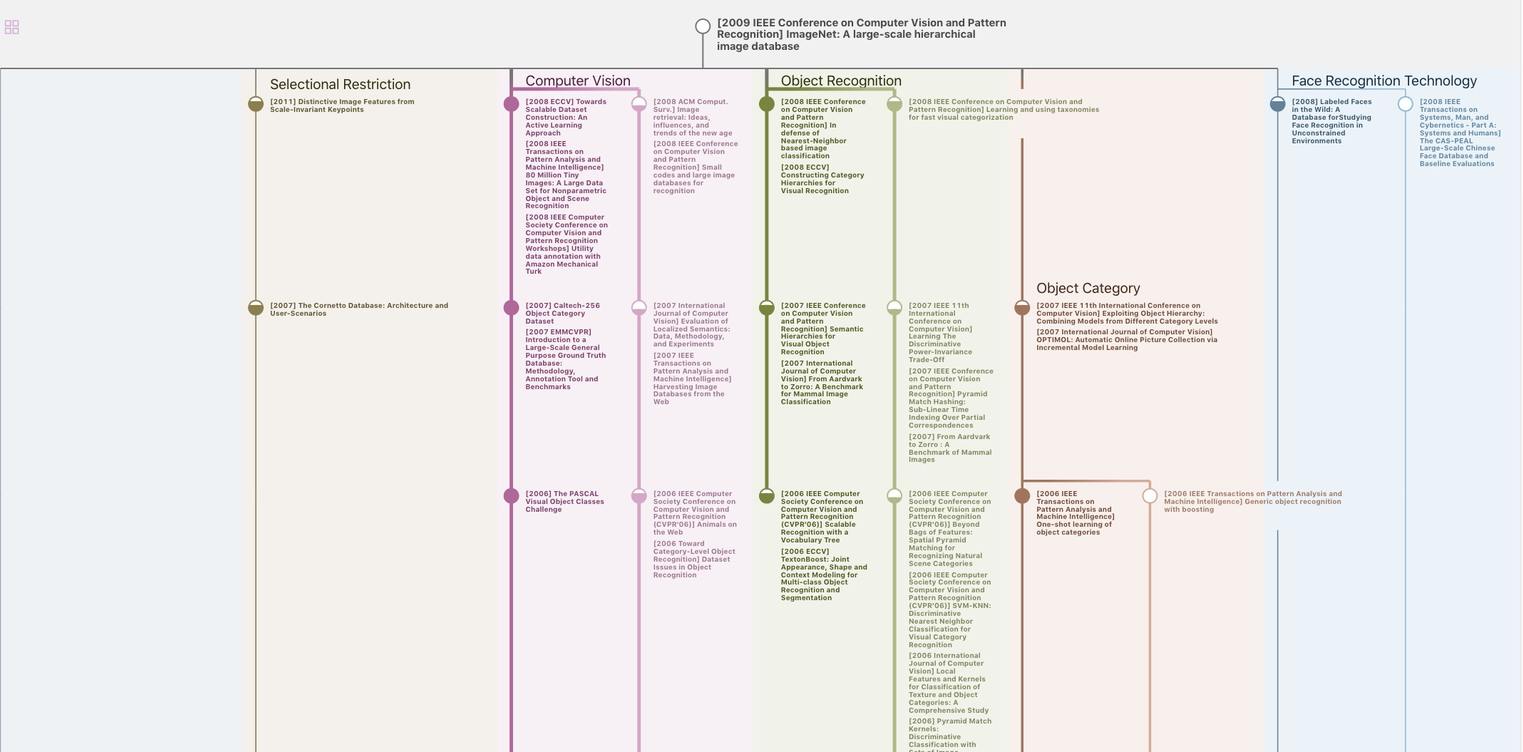
生成溯源树,研究论文发展脉络
Chat Paper
正在生成论文摘要