Steady-state response feature extraction optimization to enhance electronic nose performance
2020 7th International Conference on Electrical Engineering, Computer Sciences and Informatics (EECSI)(2020)
摘要
Feature extraction of electronic nose (e-nose) output response aims to reduce information redundancy so that the e-nose performance can be improved. The use of different sensor types and sample targets can affect the optimization of feature extraction. This research used six types of metal oxide sensors, TGS 813, 822, 825, 826, 2620, and 2611 in an e-nose system to detect three types of herbal drink. Five kinds of feature extraction methods on the original response curve in a steady-state response were used, namely, baseline difference, logarithmic difference, local normalization, global normalization, and global autoscaling. The results of feature extraction were fed into a Principal Component Analysis (PCA) system. As a result, global autoscaling and normalization had the highest total sum of the first and second principal components of 96.96%, followed by local normalization (90.18%), logarithm, and baseline difference (88.92% and 79.26%, respectively). The validation of PCA results was performed using a Backpropagation Neural Network (BPNN). The highest accuracy, 97.44%, was obtained from the global autoscaling method, followed by global normalization, local normalization, logarithm, and baseline difference, with an accuracy level of 94.87%, 92.31 %, 89.74%, and 82.05%, respectively. This demonstrates that the selection of the feature extraction method can affect the classification results and improve e-nose performance.
更多查看译文
关键词
electronic nose,feature extraction,Principal Component Analysis,Backpropagation Neural Network
AI 理解论文
溯源树
样例
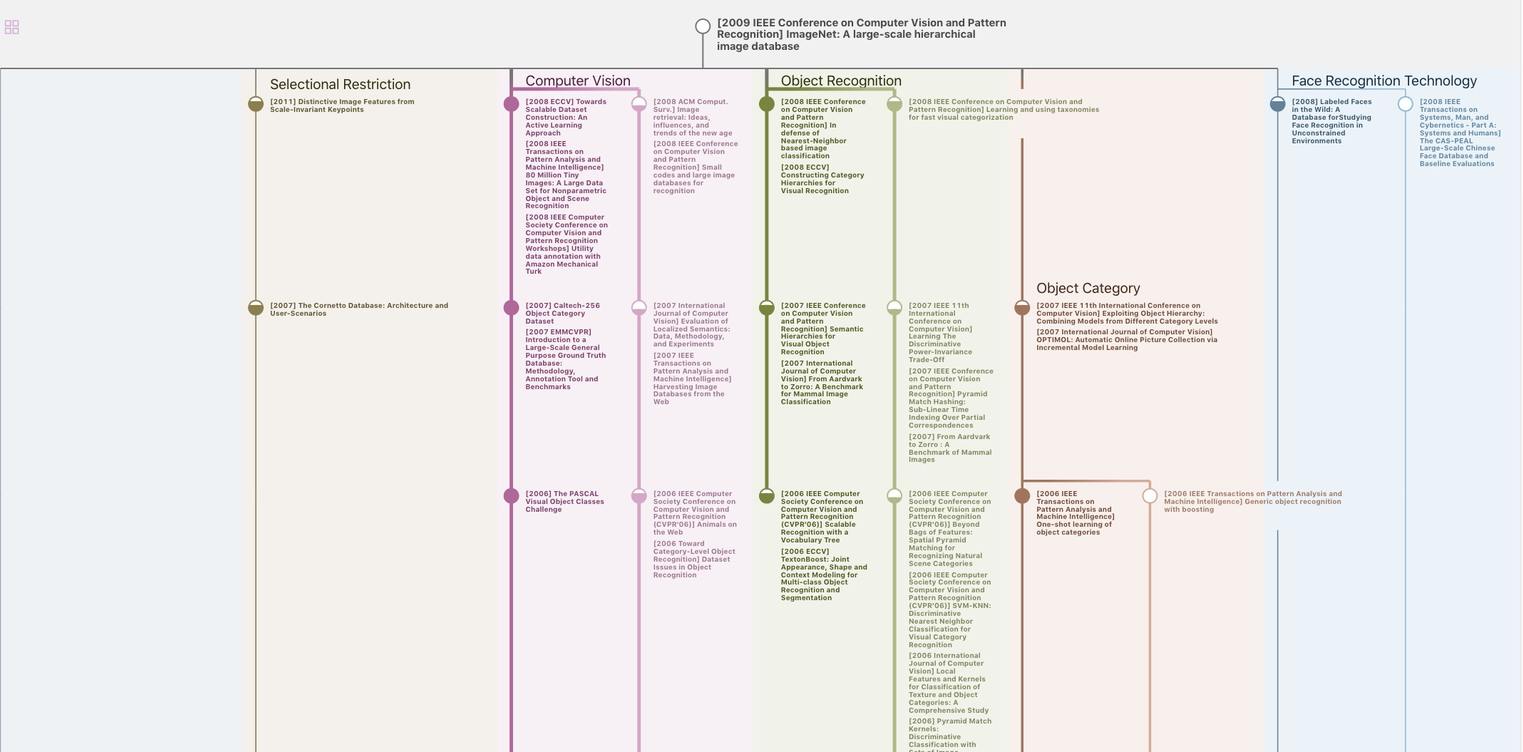
生成溯源树,研究论文发展脉络
Chat Paper
正在生成论文摘要