Identification and Classification of Cyberbullying Posts: A Recurrent Neural Network Approach Using Under-Sampling and Class Weighting
ICONIP (5)(2020)
摘要
With the number of users of social media and web platforms increasing day-by-day in recent years, cyberbullying has become a ubiquitous problem on the internet. Controlling and moderating these social media platforms manually for online abuse and cyberbullying has become a very challenging task. This paper proposes a Recurrent Neural Network (RNN) based approach for the identification and classification of cyberbullying posts. In highly imbalanced input data, a Tomek Links approach does under-sampling to reduce the data imbalance and remove ambiguities in class labelling. Further, the proposed classification model uses Max-Pooling in combination with Bi-directional Long Short-Term Memory (LSTM) network and attention layers. The proposed model is evaluated using Wikipedia datasets to establish the effectiveness of identifying and classifying cyberbullying posts. The extensive experimental results show that our approach performs well in comparison to competing approaches in terms of precision, recall, with F1 score as 0.89, 0.86 and 0.88, respectively.
更多查看译文
关键词
Cyberbullying,Natural language processing,Under-sampling,Recurrent Neural Network,Social media
AI 理解论文
溯源树
样例
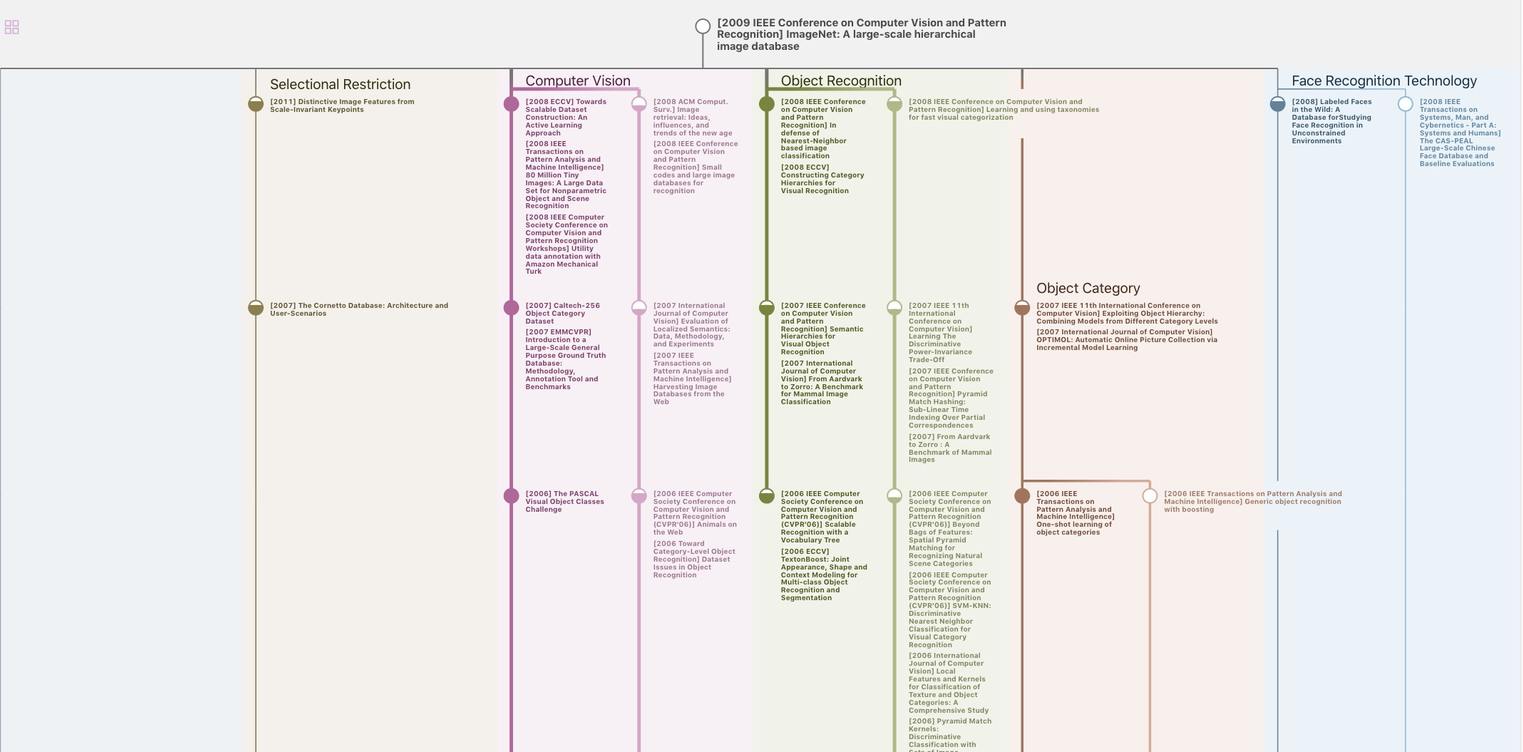
生成溯源树,研究论文发展脉络
Chat Paper
正在生成论文摘要