Dnn-Based Speed-Of-Sound Reconstruction For Automated Breast Ultrasound
PROCEEDINGS OF THE 2020 IEEE INTERNATIONAL ULTRASONICS SYMPOSIUM (IUS)(2020)
摘要
The gold-standard for breast cancer screening is x-ray mammography. Alongside, ultrasound scans are being used as additional source of information for patients with dense breast tissue. However, conventional ultrasound imaging is a qualitative approach and is prone to errors. Quantitative approaches can provide valuable information about tissue properties, e.g. the speed-of-sound in the tissue can be used as a biomarker for breast tissue malignancy. Recent studies showed the possibility of speed-of-sound reconstruction from ultrasound raw data using Deep Neural Networks (DNNs). In this study, we investigate the feasibility of DNN-based speed-of-sound reconstruction for automated breast ultrasound with simulated and real data. We set up a DNN for speed-of-sound reconstruction. The network is fully trained on simulated data. Simulations are based on the LightABVS transducer, a linear transducer with 192 active channels. The input of the network is raw channel data from a single plane-wave acquisition. The output of the network is a speed-of-sound map with a resolution of 0.1 mm. We achieved Mean Absolute Percentage Error of 0.39 +/- 0.03% and Root-Mean-Square Error of 14.85 +/- 0.52 m/s on simulated dataset and promising results on real dataset which demonstrates great potential of this method for integration in conventional ultrasound systems.
更多查看译文
关键词
Speed-of-Sound, Deep Neural Networks, Breast Ultrasound
AI 理解论文
溯源树
样例
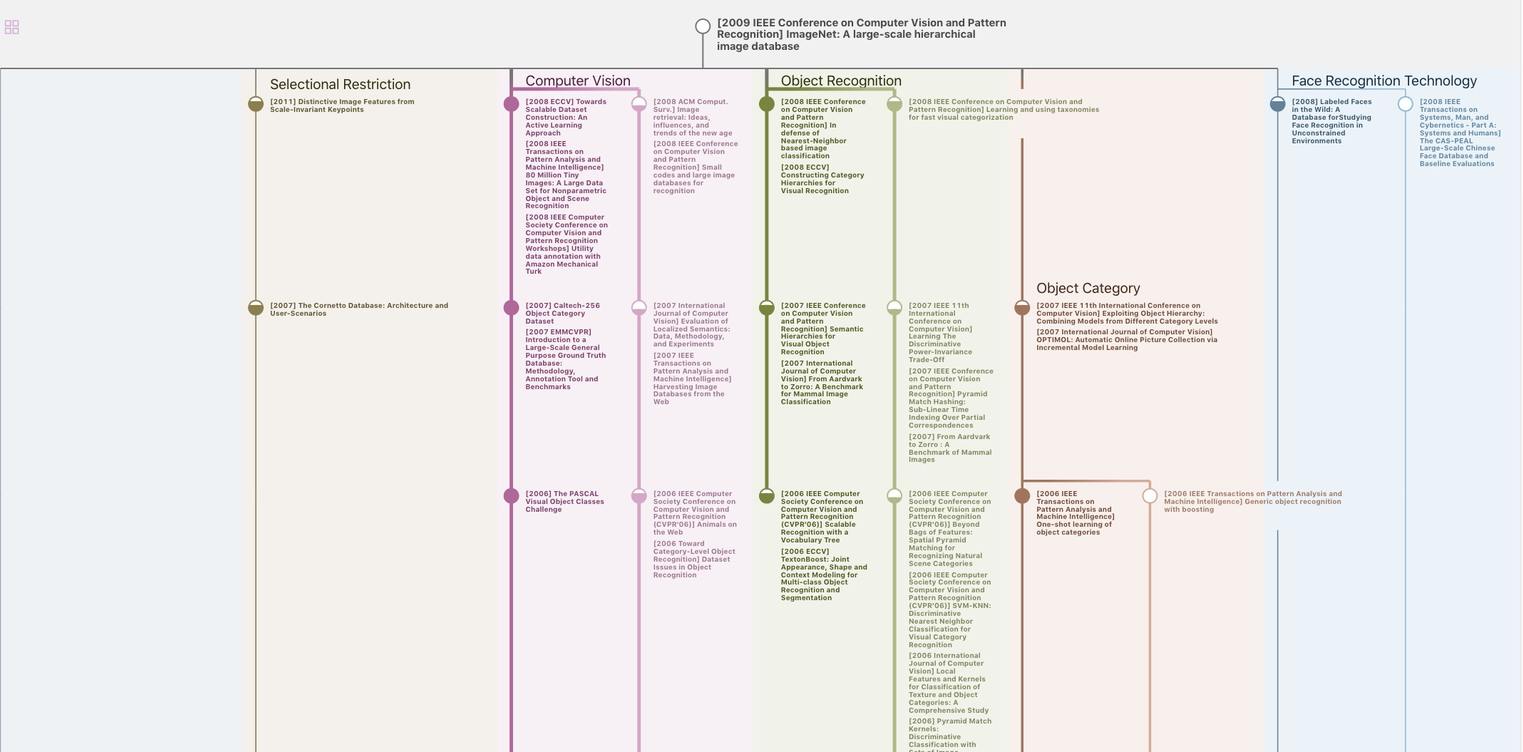
生成溯源树,研究论文发展脉络
Chat Paper
正在生成论文摘要