Conditional Generative Adversarial Networks for Inorganic Chemical Compositions
Chemistry letters(2021)
摘要
In recent years, inverse material design using machine learning techniques has attracted attention for material development. Almost all studies have used crystal structures of materials, although material engineers rarely store the crystal information and they only save chemical compositions and target properties for high-throughput materials discovery. Thus, we propose a method to generate chemical compositions for desired target properties by using conditional generative adversarial networks (CondGAN) and a post-processing method to balance the oxidation numbers. Numerical experimental results demonstrate that our CondGAN generates chemical compositions holding the desired properties.
更多查看译文
关键词
Deep generative model,Materials discovery,Inverse material design
AI 理解论文
溯源树
样例
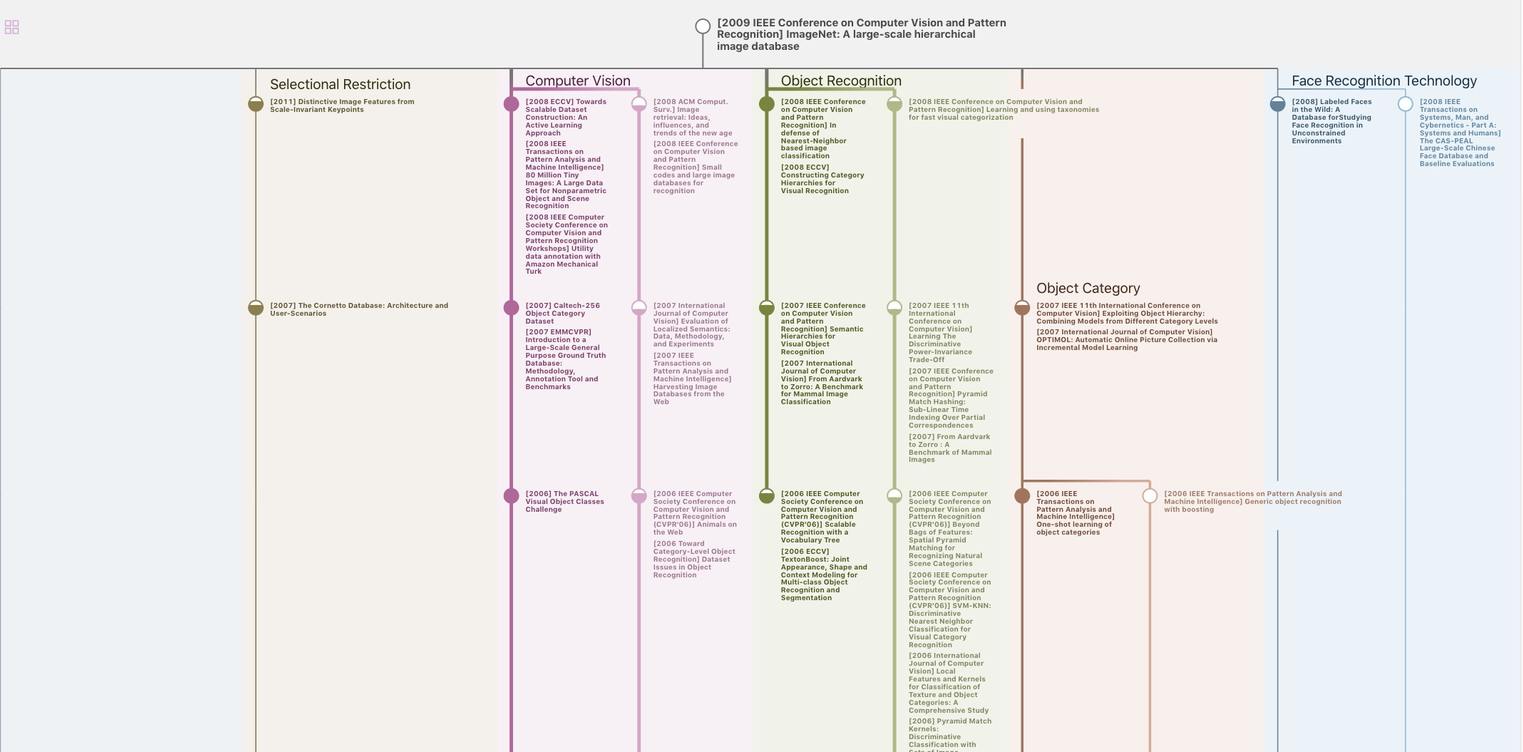
生成溯源树,研究论文发展脉络
Chat Paper
正在生成论文摘要