3d Convolutional Siamese Network For Few-Shot Hyperspectral Classification
JOURNAL OF APPLIED REMOTE SENSING(2020)
摘要
Hyperspectral classification is a widely discussed problem in the remote sensing field. Many researchers have reported good results of hyperspectral classification. However, when applied to the real world, the strong demand for labeled data for hyperspectral classification will be a big obstacle. To address this problem, researchers have explored few-shot learning and semisupervised methods in a variety of papers. We propose a siamese network composed of three-dimensional convolutional neural networks named 3DCSN. We design a structure for 3DCSN that combines contrast information with label information and get a satisfying classification result. With only a few labeled samples, it performs better than the baseline methods. Moreover, it is an end-to-end network that can use joint training. The experiments indicate the great potential of our method. (C) 2020 Society of Photo-Optical Instrumentation Engineers (SPIE)
更多查看译文
关键词
deep learning, hyperspectral imaging, few-shot learning, siamese network
AI 理解论文
溯源树
样例
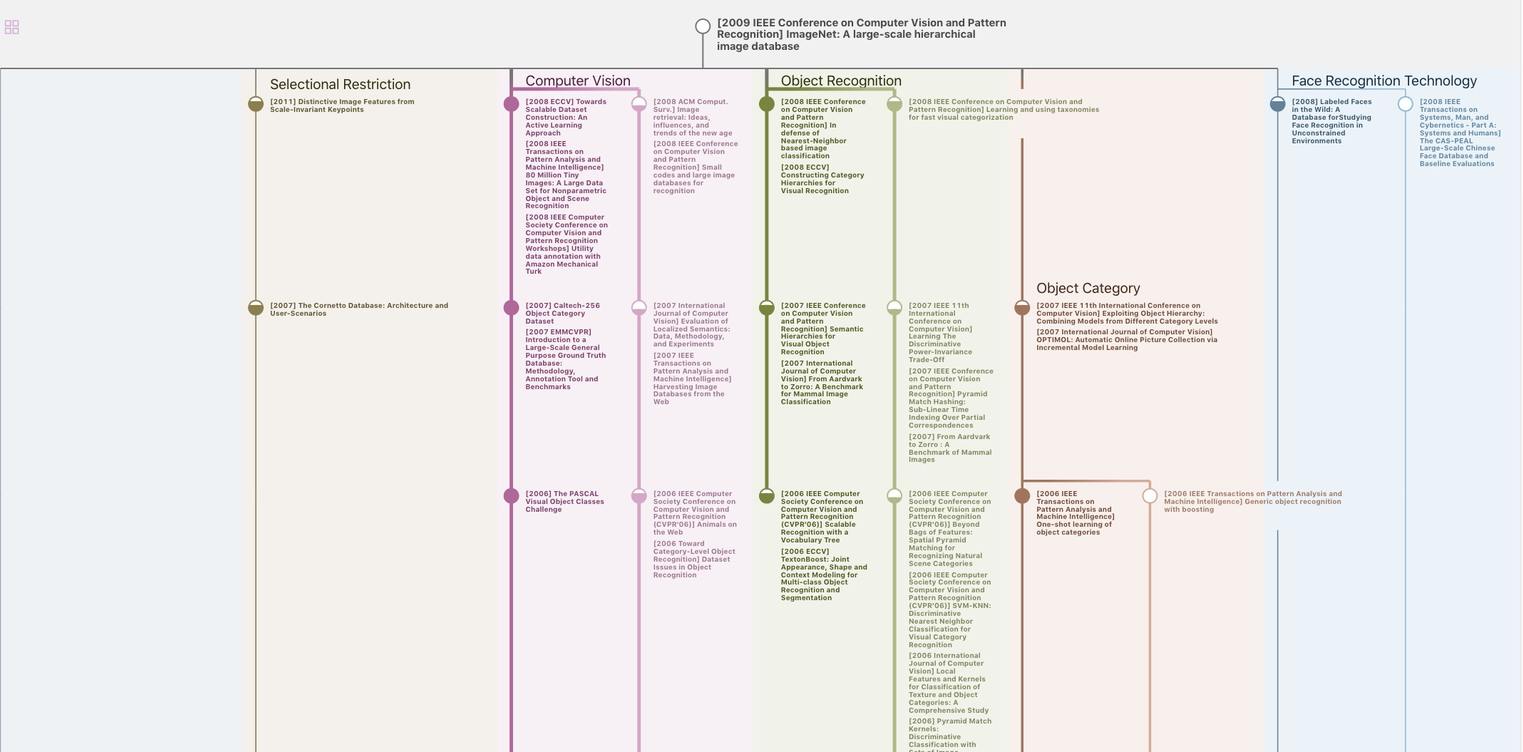
生成溯源树,研究论文发展脉络
Chat Paper
正在生成论文摘要