Development Of An Image Data Set Of Construction Machines For Deep Learning Object Detection
JOURNAL OF COMPUTING IN CIVIL ENGINEERING(2021)
摘要
Deep learning object detection algorithms have proven their capacity to identify a variety of objects from images and videos in near real-time speed. The construction industry can potentially benefit from this machine intelligence by linking algorithms with construction videos to automatically analyze productivity and monitor activities from a safety perspective. However, an effective image data set of construction machines for training deep learning object detection algorithms is not currently available due to the limited accessibility of construction images, the time-and-labor-intensiveness of manual annotations, and the knowledge base required in terms of both construction and deep learning. This research presents a case study on developing an image data set specifically for construction machines named the Alberta Construction Image Data Set (ACID). In the case of ACID, 10,000 images belonging to 10 types of construction machines are manually collected and annotated with machine types and their corresponding positions on the images. To validate the feasibility of ACID, we train the data set using four existing deep learning object detection algorithms, including YOLO-v3, Inception-SSD, R-FCN-ResNet101, and Faster-RCNN-ResNet101. The mean average precision (mAP) is 83.0% for Inception-SSD, 87.8% for YOLO-v3, 88.8% for R-FCN-ResNet101, and 89.2% for Faster-RCNN-ResNet101. The average detection speed of the four algorithms is 16.7 frames per second (fps), which satisfies the needs of most studies in the field of automation in construction.
更多查看译文
关键词
Deep learning, Object detection, Algorithm analysis, Construction machines, Image data set
AI 理解论文
溯源树
样例
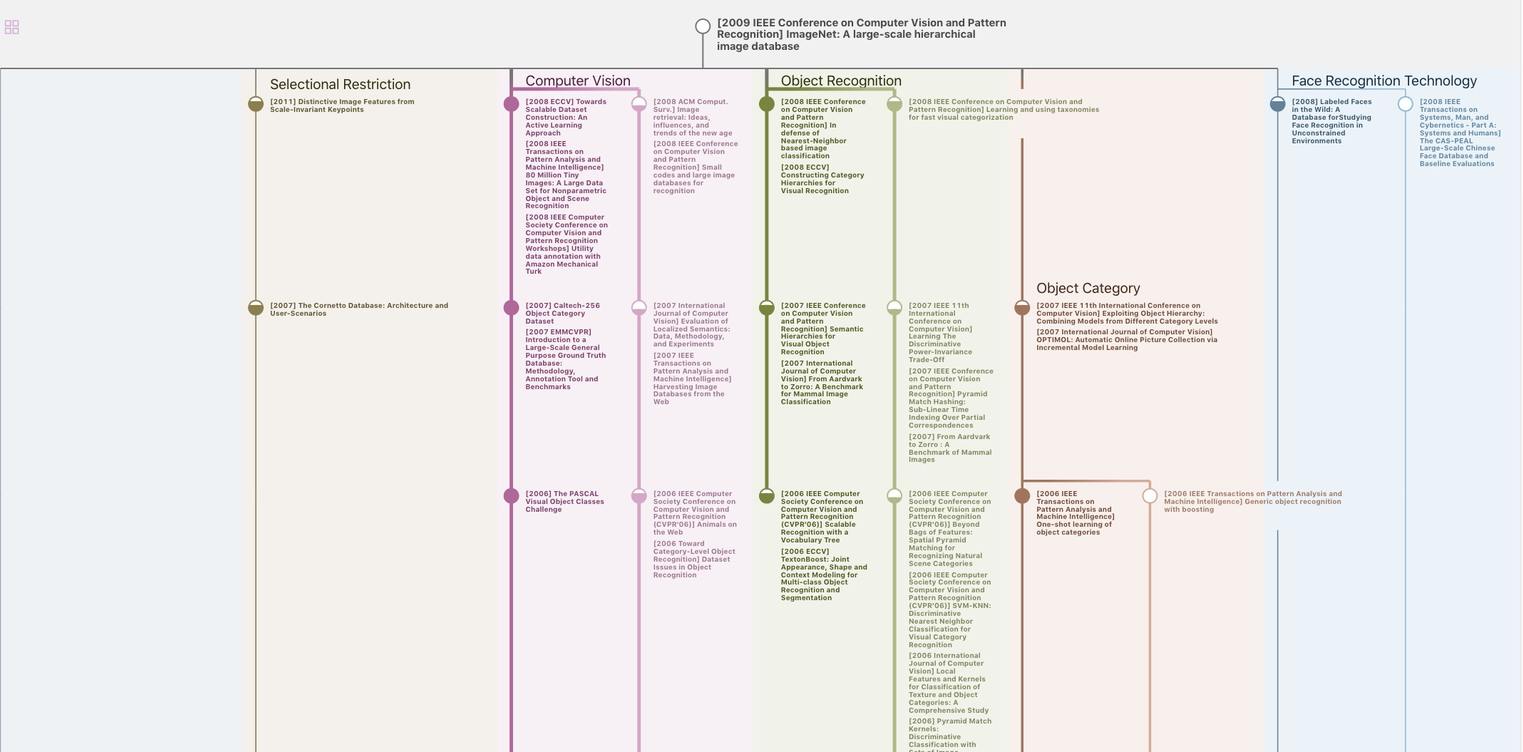
生成溯源树,研究论文发展脉络
Chat Paper
正在生成论文摘要