Conditional Activation Gan: Improved Auxiliary Classifier Gan
IEEE ACCESS(2020)
摘要
A conditional generative adversarial network (cGAN) is a generative adversarial network (GAN) that generates data with a desired condition from a latent vector. Among the different types of cGAN, the auxiliary classifier GAN (ACGAN) is the most frequently used. In this study, we describe the problems of an AC-GAN and propose replacing it with a conditional activation GAN (CAGAN) to reduce the number of hyperparameters and improve the training speed. The loss function of a CAGAN is defined as the sum of the loss of each GAN created for each condition. The proposed CAGAN is an integration of multiple GANs, where each GAN shares all hidden layers, and their integration can be considered as a single GAN. Therefore, the structure of the integrated GANs does not significantly increase the number of computations. Additionally, to prevent the conditions given in the discriminator of a cGAN from being ignored with batch normalization, we propose mixed batch training, in which every batch for the discriminator keeps the ratio of the real and generated data consistent.
更多查看译文
关键词
Generative adversarial networks, Training, Generators, Gallium nitride, Licenses, Probability distribution, Entropy, Artificial neural networks, auxiliary classifier GAN, batch normalization, conditional GAN, deep learning, generative adversarial networks, loss function
AI 理解论文
溯源树
样例
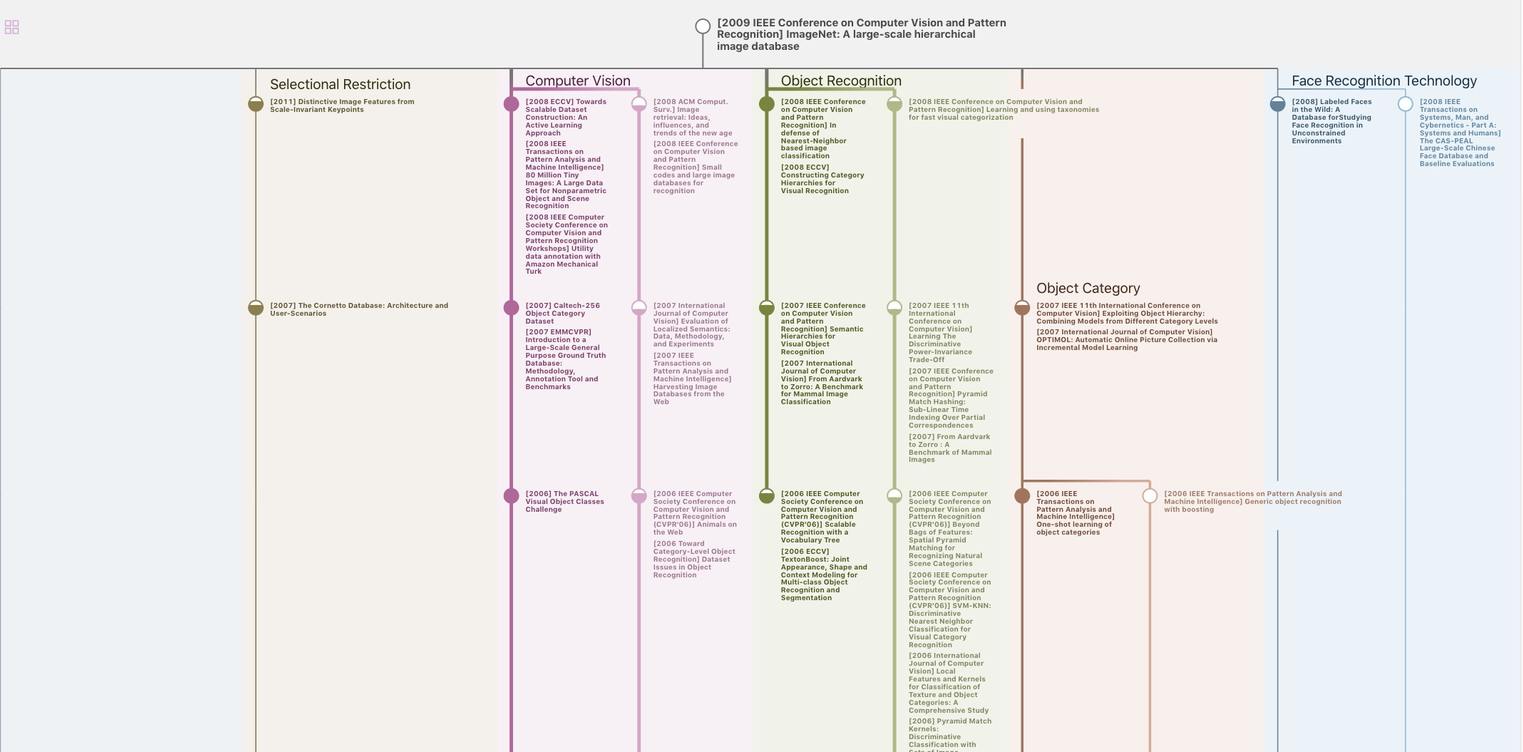
生成溯源树,研究论文发展脉络
Chat Paper
正在生成论文摘要