Domain-Indexing Variational Bayes: Interpretable Domain Index for Domain Adaptation.
ICLR 2023(2023)
摘要
Previous studies have shown that leveraging domain index can significantly boost domain adaptation performance (arXiv:2007.01807, arXiv:2202.03628). However, such domain indices are not always available. To address this challenge, we first provide a formal definition of domain index from the probabilistic perspective, and then propose an adversarial variational Bayesian framework that infers domain indices from multi-domain data, thereby providing additional insight on domain relations and improving domain adaptation performance. Our theoretical analysis shows that our adversarial variational Bayesian framework finds the optimal domain index at equilibrium. Empirical results on both synthetic and real data verify that our model can produce interpretable domain indices which enable us to achieve superior performance compared to state-of-the-art domain adaptation methods. Code is available at https://github.com/Wang-ML-Lab/VDI.
更多查看译文
关键词
interpretable domain-indexing domain-indexing,adaptation
AI 理解论文
溯源树
样例
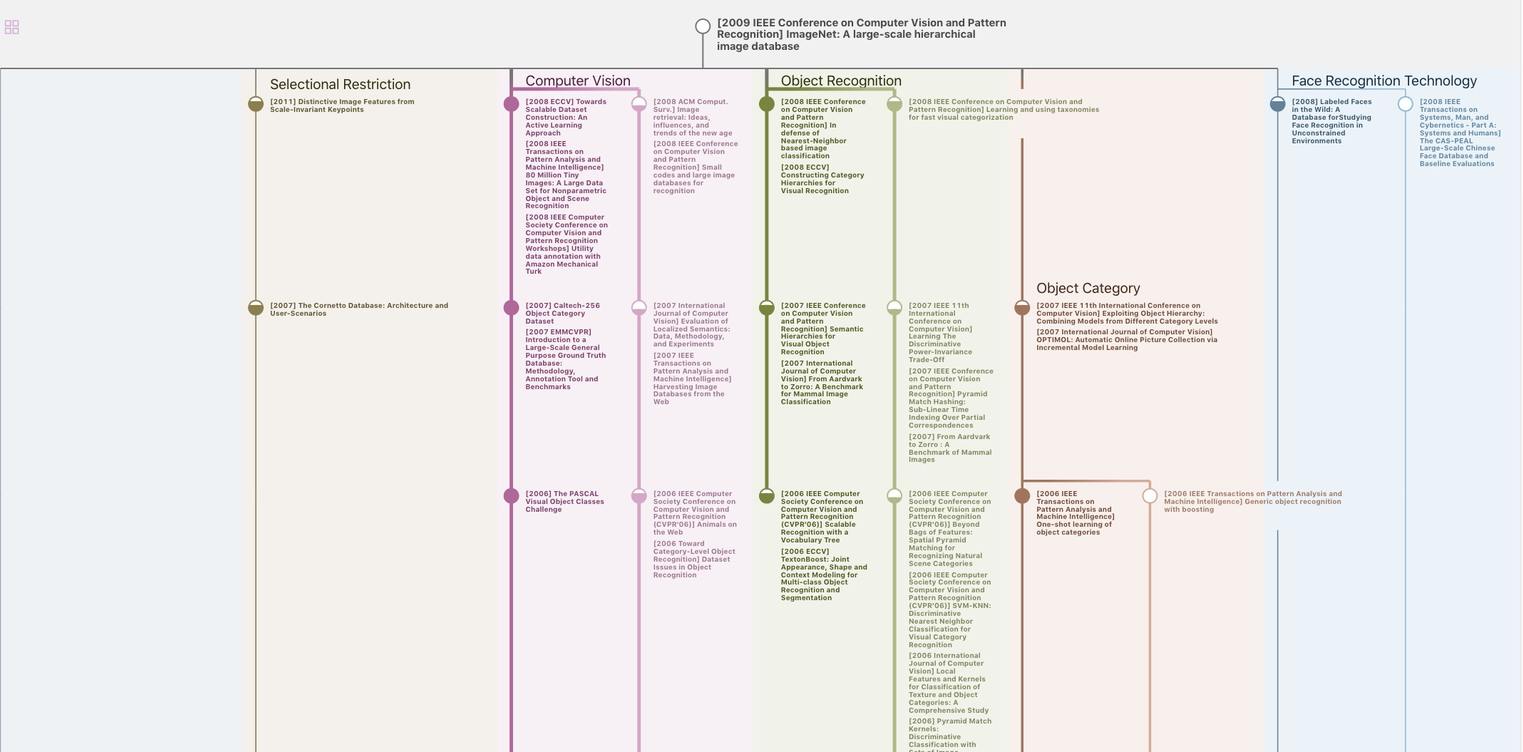
生成溯源树,研究论文发展脉络
Chat Paper
正在生成论文摘要