Missing-Insensitive Short-Term Load Forecasting Leveraging Autoencoder And Lstm
IEEE ACCESS(2020)
摘要
In most deep learning-based load forecasting, an intact dataset is required. Since many real-world datasets contain missing values for various reasons, missing imputation using deep learning is actively studied. However, missing imputation and load forecasting have been considered independently so far. In this article, we provide a deep learning framework that jointly considers missing imputation and load forecasting. We consider a family of autoencoder/long short-term memory (LSTM) combined models for missing-insensitive load forecasting. Specifically, autoencoder (AE), denoising autoencoder (DAE), convolutional autoencoder (CAE), and denoising convolutional autoencoder (DCAE) are considered for extracting features, of which the encoded outputs are fed into the input of LSTM. Our experiments show that the proposed DCAE/LSTM combined model significantly improves forecasting accuracy no matter what missing rate or type (random missing, consecutive block missing) occurs compared to the baseline LSTM.
更多查看译文
关键词
Deep learning,short-term load forecasting,missing data imputation,feature extraction
AI 理解论文
溯源树
样例
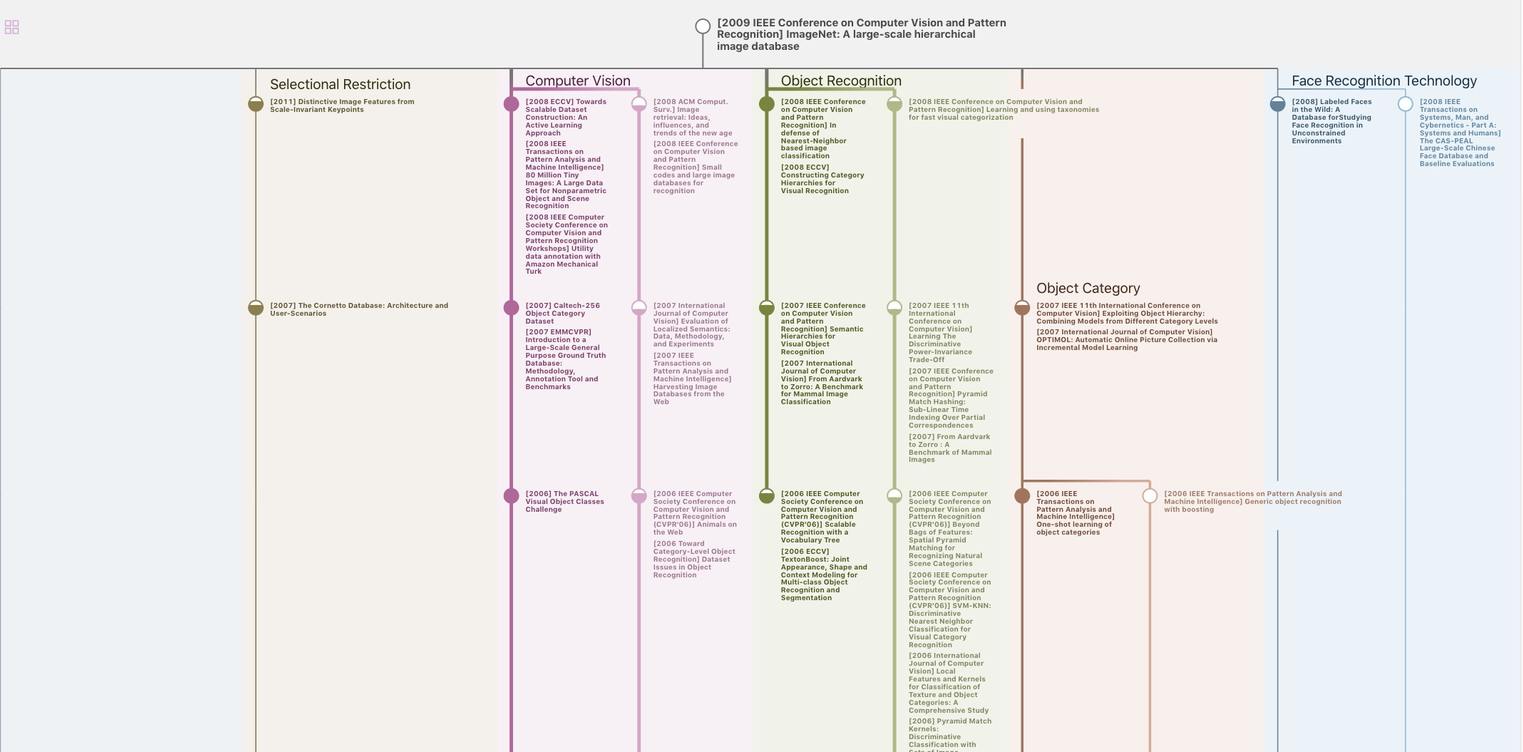
生成溯源树,研究论文发展脉络
Chat Paper
正在生成论文摘要