Crash Risk Assessment for Heterogeneity Traffic and Different Vehicle-Following Patterns Using Microscopic Traffic Flow Data
SUSTAINABILITY(2020)
摘要
This paper investigates the impacts of heavy vehicles (HV) on speed variation and assesses the rear-end crash risk for four vehicle-following patterns in a heterogeneous traffic flow condition using three surrogate safety measures: speed variation, time-to-collision (TTC), and deceleration rate to avoid a crash (DRAC). A video-based data collection approach was employed to collect the speed of each individual vehicle and vehicle-following headway; a total of 3859 vehicle-following pairs were identified. Binary logistic regression modeling was employed to assess the impacts of HV percentage on crash risk. TTCs and DRACs were calculated based on the collected traffic flow data. Analytical models were developed to estimate the minimum safe vehicle-following headways for the four vehicle-following patterns. Field data revealed that the variation of speed first increased with HV percentage and reached the maximum when HV percentage was at around 0.35; then, it displayed a decreasing trend with HV percentage. Binary logistic regression modeling results suggest that a high risk of rear-end collision is expected when HV percentage is between 0.19 and 0.5; while, when HV percentage is either below 0.19 or exceed 0.5, a low risk of rear-end collision is anticipated. Analytical modeling results show that the passenger car (PC)-HV vehicle-following pattern requires the largest minimum safe space headway, followed by HV-HV, PC-PC, and HV-PC vehicle-following patterns. Findings from this research present insights to transportation engineers regarding the development of crash mitigation strategies and have the potential to advance the design of real-time in-vehicle forward collision warnings to minimize the risk of rear-end crash.
更多查看译文
关键词
heterogeneity traffic,vehicle-following pattern,speed variation,crash risk assessment,minimum safe headway
AI 理解论文
溯源树
样例
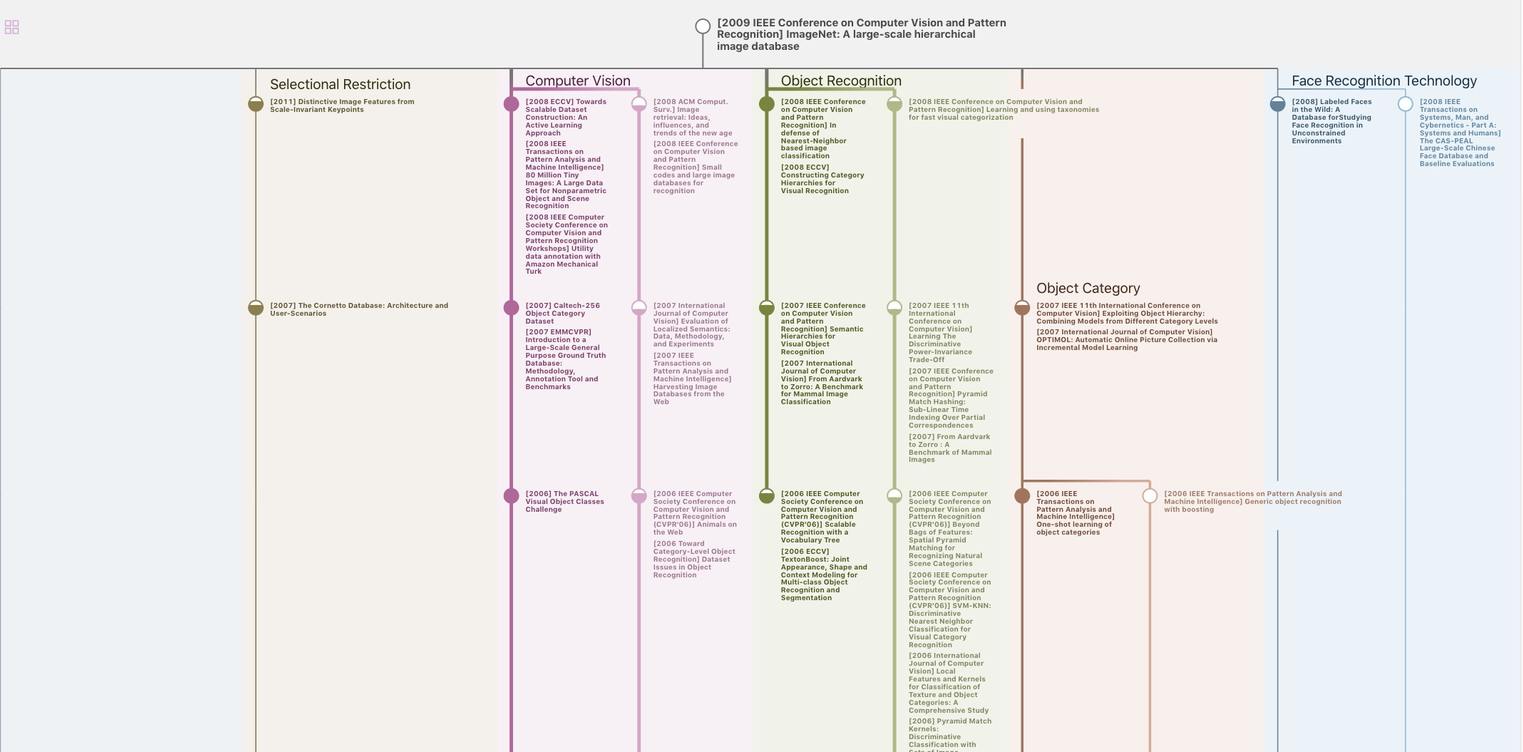
生成溯源树,研究论文发展脉络
Chat Paper
正在生成论文摘要