Statistical Analysis of Clustering Performances of NMF, Spectral Clustering, and K-means
2020 2nd International Conference on Computer and Information Sciences (ICCIS)(2020)
摘要
Nonnegative matrix factorization (NMF), spectral clustering, and k-means are the most used clustering methods in machine learning research. They have been used in many domains including text, image, and cancer clustering. However, there is still a limited number of works that discuss statistical significance of performance differences between these methods. This issue is epecially important in NMF as this method is still very actively researched with a sheer number of new algorithms are published every year, and being able to demonstrate newly proposed algorithms statistically outperform previous ones is certainly desired. In this paper, we present statistical analysis of clustering performance differences between NMF, spectral clustering, and k-means. We use ten NMF algorithms, six spectral clustering algorithms, and one standard k-means algorithm for benchmark. For data, eleven publicly available microarray gene expression datasets with numbers of classes range from two to ten are used. The experimental results show that statistically performance differences between NMF algorithms and the standard k-means algorithm are not significant, and spectral methods surprisingly perform less well than NMF and k-means.
更多查看译文
关键词
k-means,nonnegative matrix factorization,spectral clustering,statistical analysis
AI 理解论文
溯源树
样例
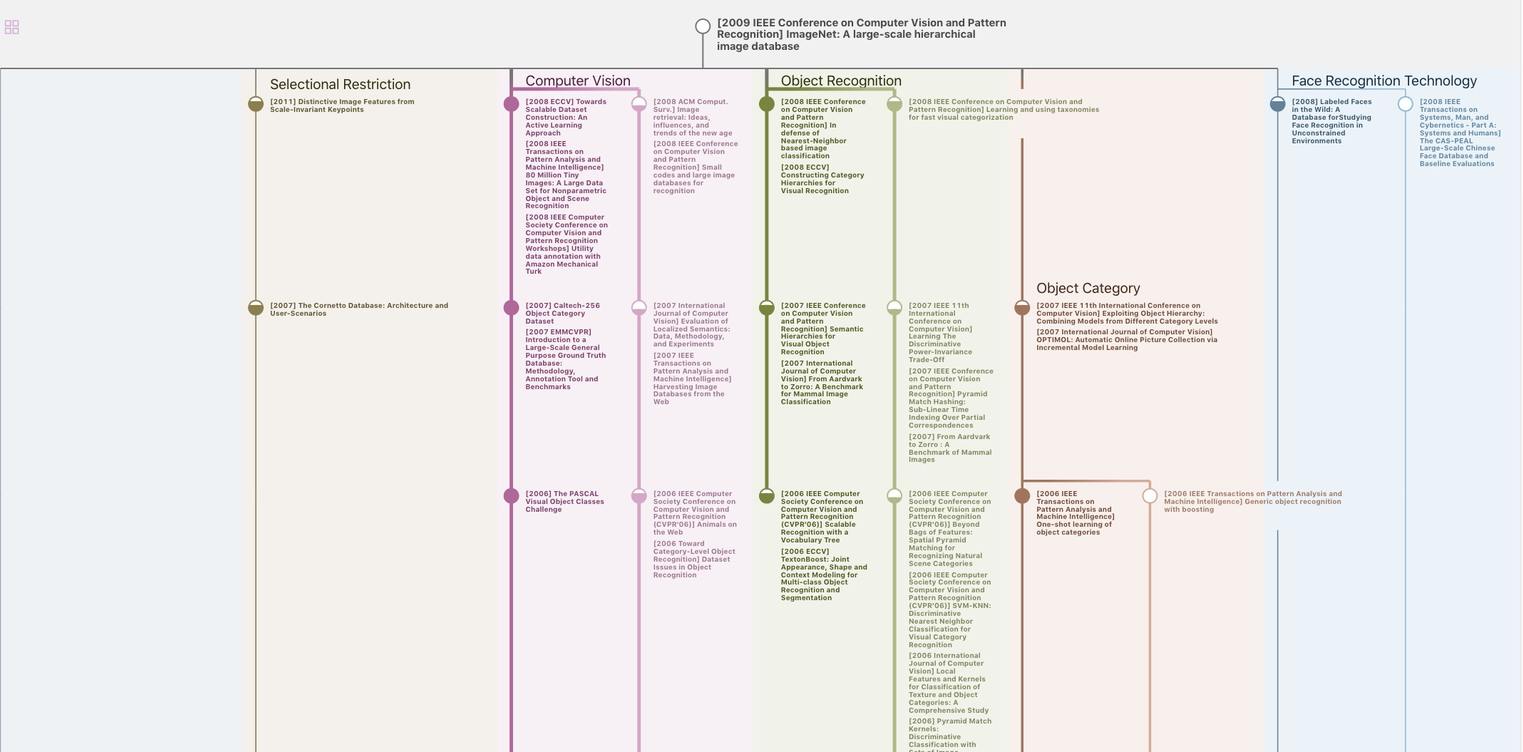
生成溯源树,研究论文发展脉络
Chat Paper
正在生成论文摘要