Tracking Control Using Recurrent-Neural-Network-Based Inversion Model: A Case Study On A Piezo Actuator
IEEE TRANSACTIONS ON INDUSTRIAL ELECTRONICS(2021)
摘要
This article is concerned with designing a broadband, high accuracy, and computationally efficient real-time controller for piezo actuators (PEAs). The essential component proposed is a recurrent-neural-network (RNN) based inversion model (RNNinv) used to compensate for the PEA nonlinearities. However, the obtained RNNinv may not efficiently model the low-frequency and/or time-varying dynamics of the system due to the limited length of the RNN training set. To address this issue, a linear model embedded with an error term is used to model the low-frequency dynamics in case it is not precisely modeled by RNNinv, and a predictive controller based on this linear model is then designed for precise output tracking. To validate the proposed control framework, the closed-loop stability condition is derived, and the RNNinv stability in unforced mode is investigated. To further improve the accuracy, a mechanism is proposed to separate the controlling dynamics to achieve higher accuracy for applications that cover broad and/or high-frequency ranges. The proposed approach was implemented on a commercial PEA and its performance was demonstrated through comparison with other controllers.
更多查看译文
关键词
Computational modeling, Predictive models, Training, Bandwidth, Time series analysis, Recurrent neural networks, Real-time systems, Inversion model, Piezo actuator (PEA), predictive control, recurrent neural network (RNN)
AI 理解论文
溯源树
样例
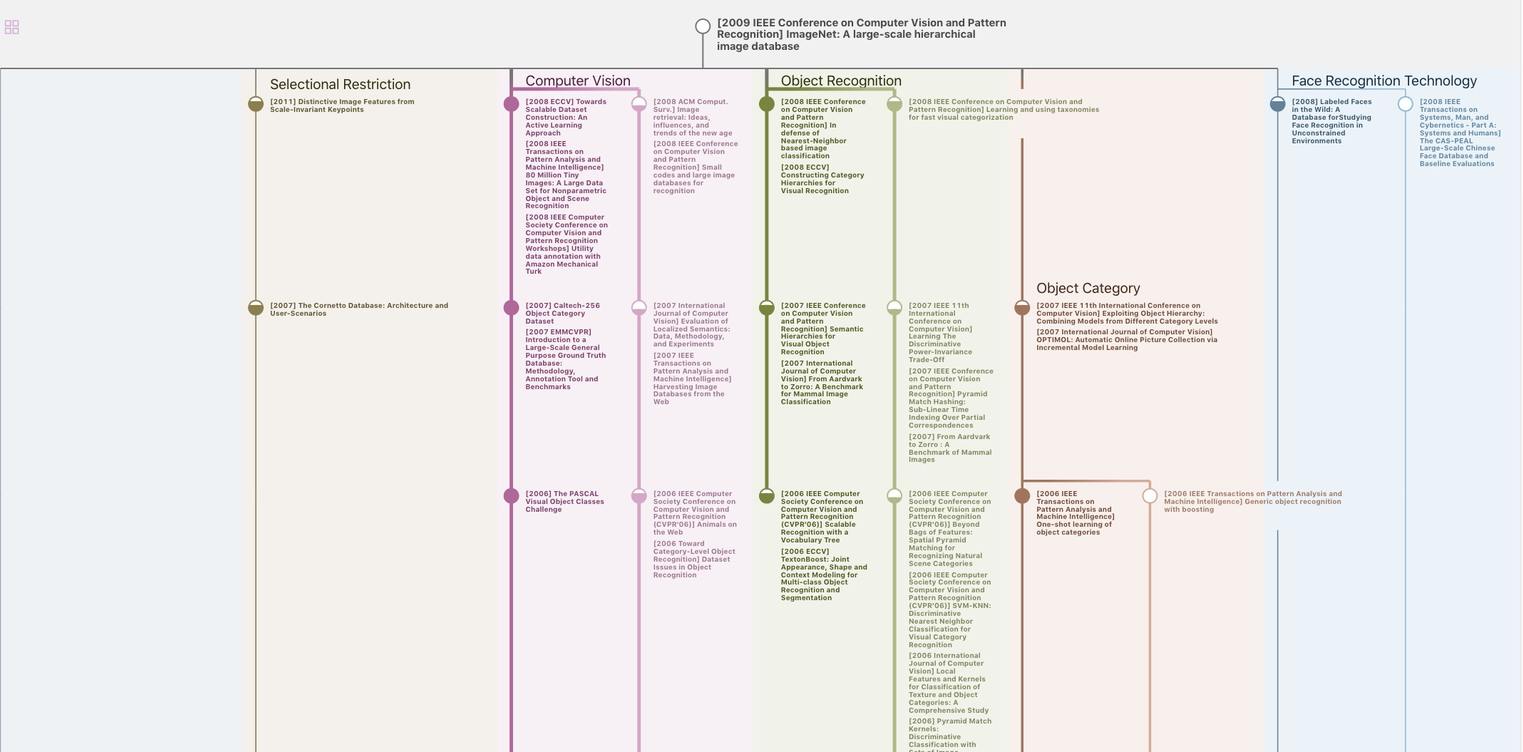
生成溯源树,研究论文发展脉络
Chat Paper
正在生成论文摘要