Explicit Modeling Of Meteorological Explanatory Variables In Short-Term Forecasting Of Maximum Ozone Concentrations Via A Multiple Regression Time Series Framework
ATMOSPHERE(2020)
摘要
Statistical time series forecasting is a useful tool for predicting air pollutant concentrations in urban areas, especially in emerging economies, where the capacity to implement comprehensive air quality models is limited. In this study, a general multiple regression with seasonal autoregressive moving average errors model was estimated and implemented to forecast maximum ozone concentrations with a short time resolution: overnight, morning, afternoon and evening. In contrast to a number of short-term air quality time series forecasting applications, the model was designed to explicitly include the effects of meteorological variables on the ozone level as exogenous variables. As the application location, the model was constructed with data from five monitoring stations in the Monterrey Metropolitan Area of Mexico. The results show that, together with structural stochastic components, meteorological parameters have a significant contribution for obtaining reliable forecasts. The resulting model is an interpretable, useful and easily implementable model for forecasting ozone maxima. Moreover, it proved to be consistent with the general dynamics of ozone formation and provides a suitable platform for forecasting, showing similar or better performance compared to models in other existing studies.
更多查看译文
关键词
air pollution, SARMA model, stochastic model, exogenous variables
AI 理解论文
溯源树
样例
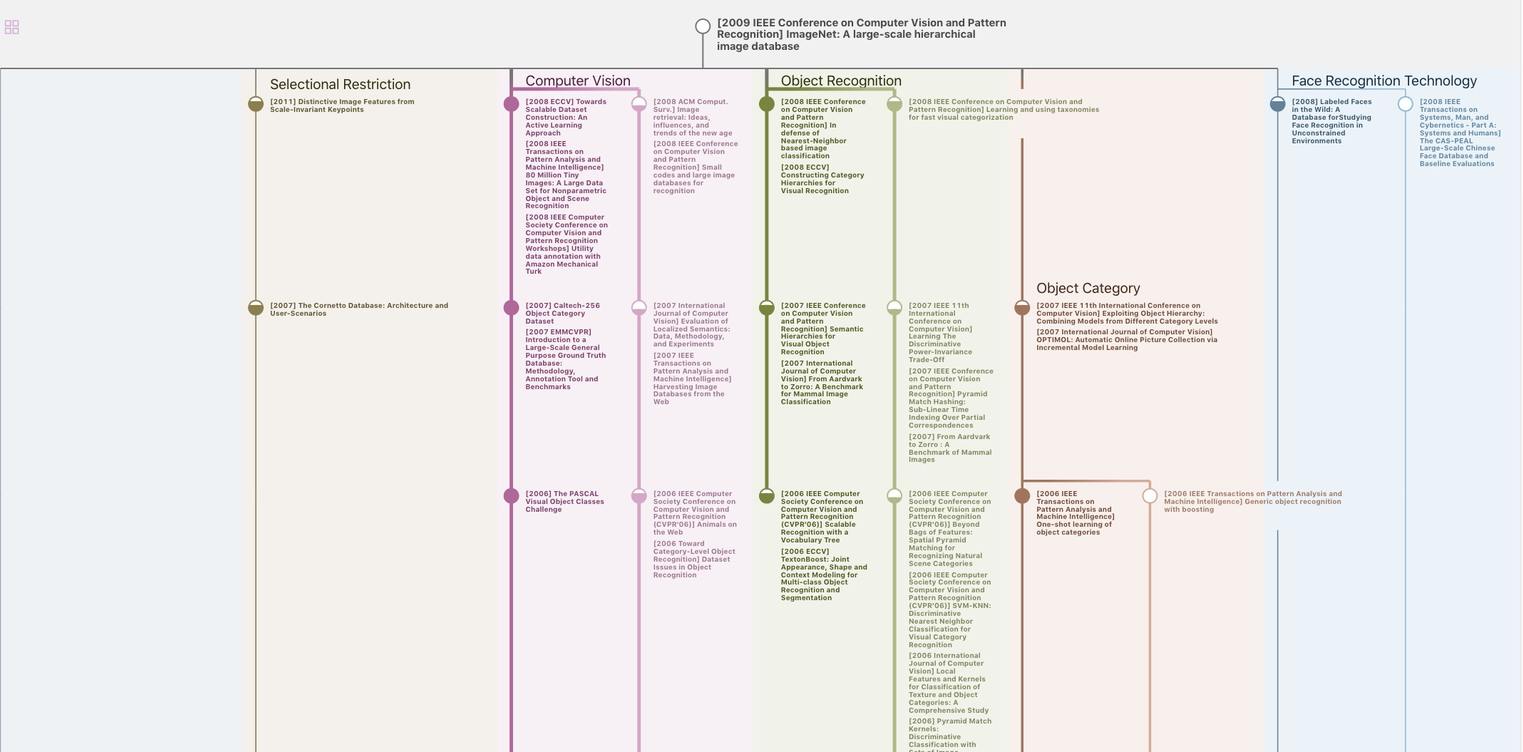
生成溯源树,研究论文发展脉络
Chat Paper
正在生成论文摘要