Novel Bounds on the Probability of Misclassification in Majority Voting: Leveraging the Majority Size
IEEE control systems letters(2021)
摘要
Majority voting is often employed as a tool to increase the robustness of data-driven decisions and control policies, a fact which calls for rigorous, quantitative evaluations of the limits and the potentials of majority voting schemes. This letter focuses on the case where the voting agents are binary classifiers and introduces novel bounds on the probability of misclassification conditioned on the size of the majority. We show that these bounds can be much smaller than the traditional upper bounds on the probability of misclassification. These bounds can be used in a `Probably Approximately Correct' (PAC) setting, which allows for a practical implementation.
更多查看译文
关键词
Training,Upper bound,Electric shock,Tools,Statistical learning,Probability distribution,Machine learning algorithms,Machine learning,agents-based systems,statistical learning
AI 理解论文
溯源树
样例
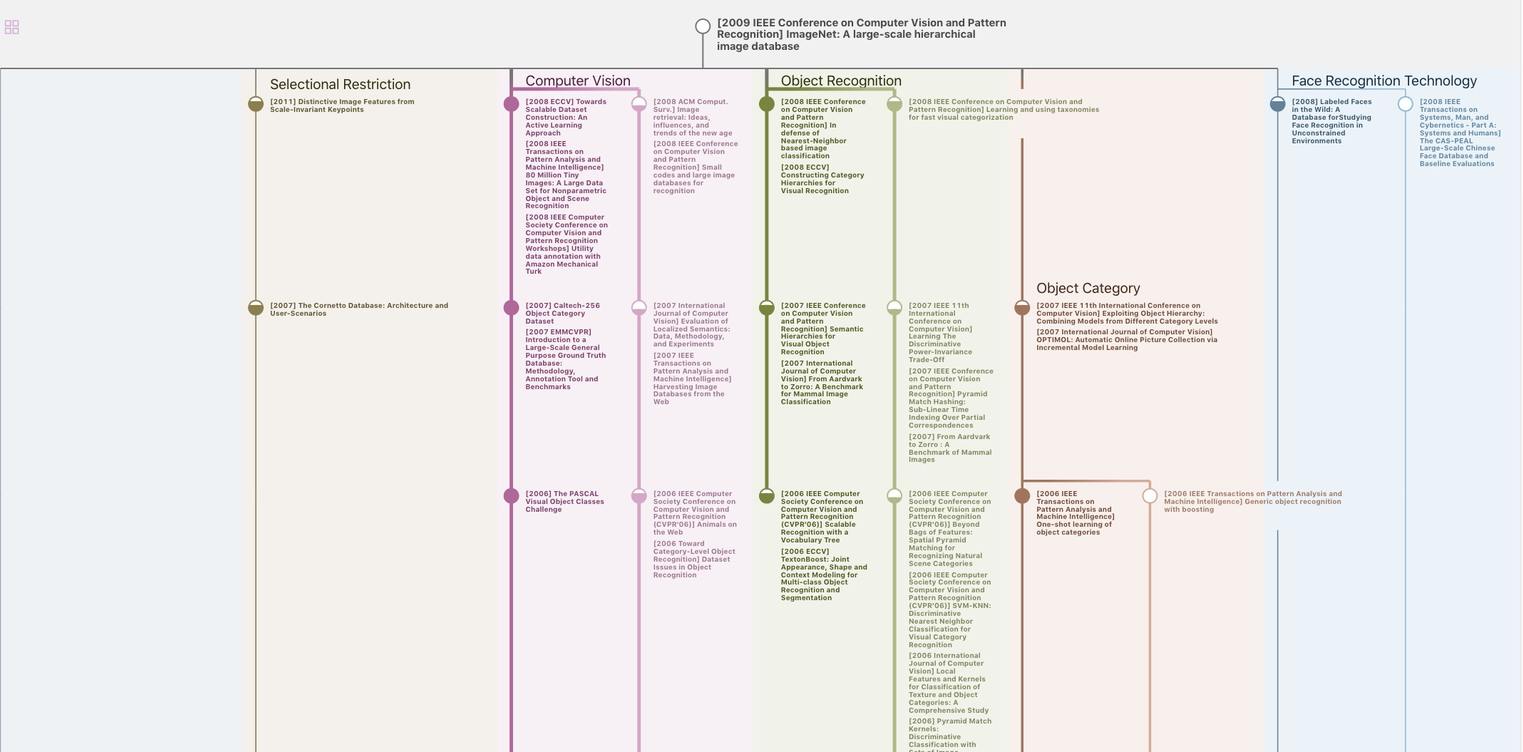
生成溯源树,研究论文发展脉络
Chat Paper
正在生成论文摘要