Enhancing Representation of Deep Features for Sensor-Based Activity Recognition
MOBILE NETWORKS & APPLICATIONS(2020)
摘要
Sensor-based activity recognition (AR) depends on effective feature representation and classification. However, many recent studies focus on recognition methods, but largely ignore feature representation. Benefitting from the success of Convolutional Neural Networks (CNN) in feature extraction, we propose to improve the feature representation of activities. Specifically, we use a reversed CNN to generate the significant data based on the original features and combine the raw training data with significant data to obtain to enhanced training data. The proposed method can not only train better feature extractors but also help better understand the abstract features of sensor-based activity data. To demonstrate the effectiveness of our proposed method, we conduct comparative experiments with CNN Classifier and CNN-LSTM Classifier on five public datasets, namely the UCIHAR, UniMiB SHAR, OPPORTUNITY, WISDM, and PAMAP2. In addition, we evaluate our proposed method in comparison with traditional methods such as Decision Tree, Multi-layer Perceptron, Extremely randomized trees, Random Forest, and k-Nearest Neighbour on a specific dataset, WISDM. The results show our proposed method consistently outperforms the state-of-the-art methods.
更多查看译文
关键词
Activity recognition, Reversed CNN, Enhancing features, Significant features
AI 理解论文
溯源树
样例
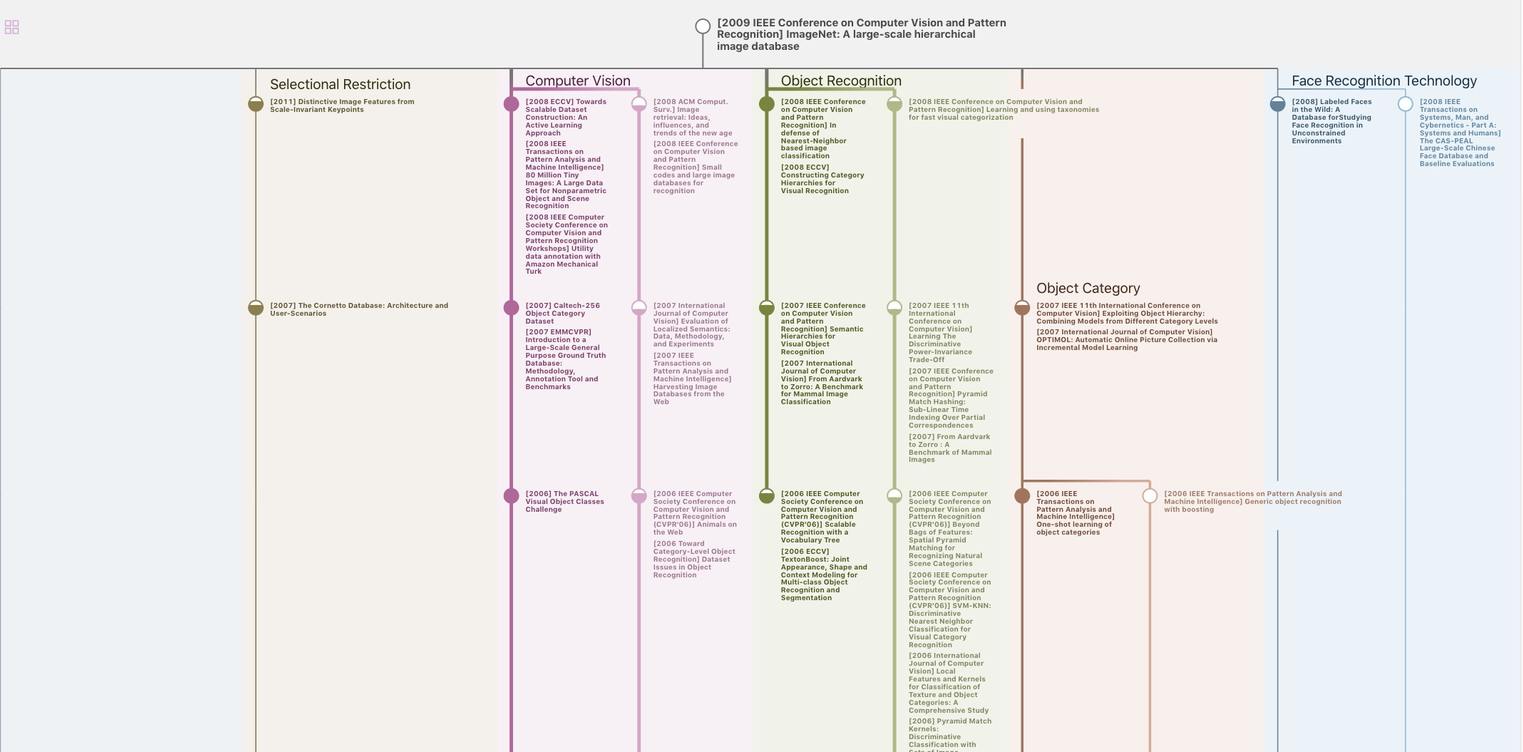
生成溯源树,研究论文发展脉络
Chat Paper
正在生成论文摘要