Learning Local And Global Priors For Jpeg Image Artifacts Removal
IEEE SIGNAL PROCESSING LETTERS(2020)
摘要
Lossy compression will inevitably introduce image artifacts in the decoded image and degrade the image quality. In recent years, convolutional neural network (CNN) has been exploited for removing compression artifacts with great success. However, most existing CNN-based methods only utilize image's local prior without considering the global prior on the training of their networks. In this letter, a novel CNN, called the local and global priors network (LGPNet), is proposed that simultaneously learns both the local and the global priors for removing compression image artifacts. To achieve this goal, a dual-attention unit (DAU) is developed and incorporated into the well-known U-Net architecture for learning a better local prior. Meanwhile, the global prior is also learned from the entire image via our proposed global prior network. Extensive experimental results have clearly demonstrated that our proposed LGPNet is able to effectively remove image artifacts and greatly improve the image quality of JPEG-compressed images.
更多查看译文
关键词
Image coding, Training, Convolution, Deep learning, Transform coding, Task analysis, Image quality, Compression artifacts removal, convolutional neural network, deep learning, image priors, image restoration, JPEG
AI 理解论文
溯源树
样例
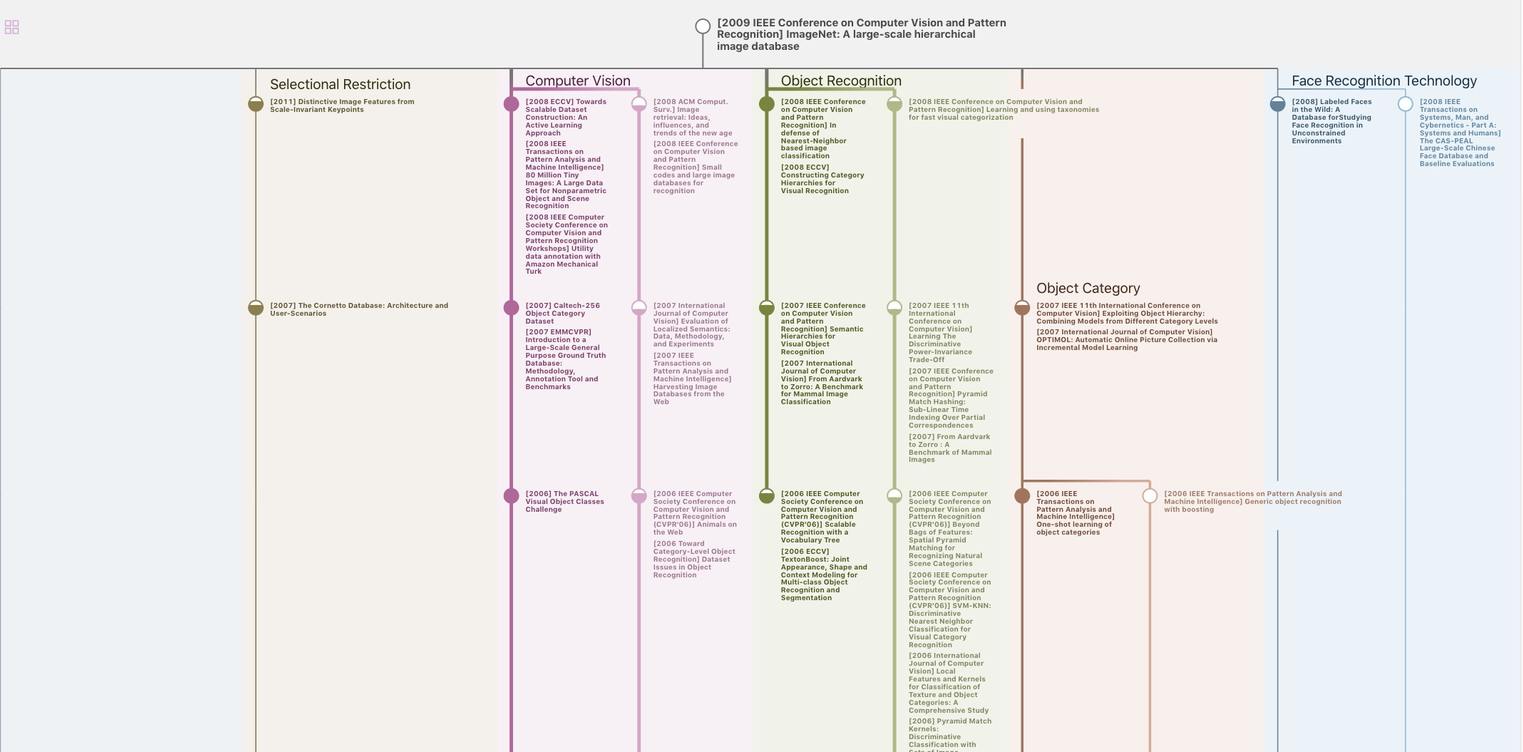
生成溯源树,研究论文发展脉络
Chat Paper
正在生成论文摘要