An Efficient Small Traffic Sign Detection Method Based on YOLOv3
JOURNAL OF SIGNAL PROCESSING SYSTEMS FOR SIGNAL IMAGE AND VIDEO TECHNOLOGY(2020)
摘要
In recent years, target detection framework based on deep learning has made brilliant achievements. However, real-life traffic sign detection remains a great challenge for most of the state-of-the-art object detection methods. The existing deep learning models are inadequate to effectively extract the features of small traffic signs from large images in real-world conditions. In this paper, we address the small traffic sign detection challenge by proposing a novel small traffic sign detection method based on a highly efficient end-to-end deep network model. The proposed method features fast speed and high precision as it attaches three key insights to the established You Only Look Once (YOLOv3) architecture and other correlated algorithms. Besides, network pruning is appropriately introduced to minimize network redundancy and model size while keeping a competitive detection accuracy. Furtherly, four scale prediction branches are also adopted to significantly enrich the feature maps of multi-scales prediction. In our method, we adjust the loss function to balance the contribution of error source to the total loss. The effectiveness, and robustness of the network is further proved with experiments on Tsinghua-Tencent 100 K traffic sign dataset. The experimental results indicate that our proposed method has achieved better accuracy than that of the original YOLOv3 model. Compared with the schemes in relevant literatures our proposed method not only emerges performance superiors in detection recall and accuracy, but also achieves 1.9–2.7x improvement in detection speed.
更多查看译文
关键词
Computer vision,Convolutional neural networks,YOLO,Traffic sign detection
AI 理解论文
溯源树
样例
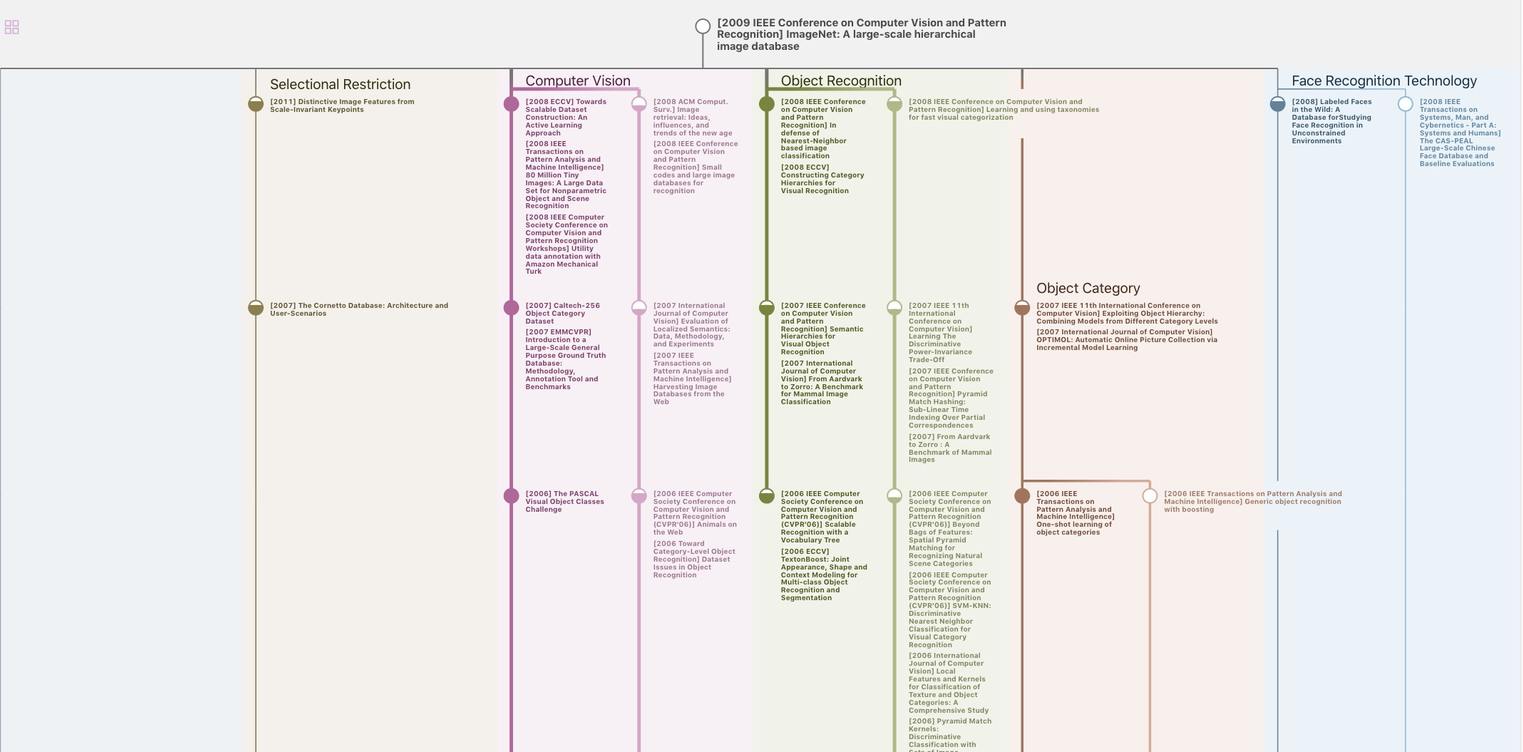
生成溯源树,研究论文发展脉络
Chat Paper
正在生成论文摘要