A Dimensionality Reduction Algorithm for Mapping Tokamak Operation Regimes Using Variational Autoencoder (VAE) Neural Network
Bulletin of the American Physical Society(2020)
摘要
A variational autoencoder (VAE) is a type of unsupervised neural network which is able to learn meaningful data representations in a reduced dimensional space. We present an application of VAE in identifying the operational stability boundary of tokamak plasma discharges. This model was implemented using a dataset of over 3000 discharges from the high beta tokamak-extended pulse (HBT-EP) device. We found the VAE model to be capable of forming a continuous low-dimensional operational space map and identifying the operational boundaries using a specified warning time window. By projecting the operational parameters onto the same reduced space, this provides an intuitive way for the machine operator or an automated control system to perform disruption avoidance using a relevant control actuator as a discharge approaches a boundary. Pre-programmed GPU control experiments were conducted to demonstrate this control technique using HBT-EP's saddle control coils as a horizontal position actuator, showing the ability to avoid the oncoming disruptive event and extend the duration of the discharge.
更多查看译文
关键词
disruption avoidance, machine learning, neural network, variational autoencoder, HBT-EP, feedback control
AI 理解论文
溯源树
样例
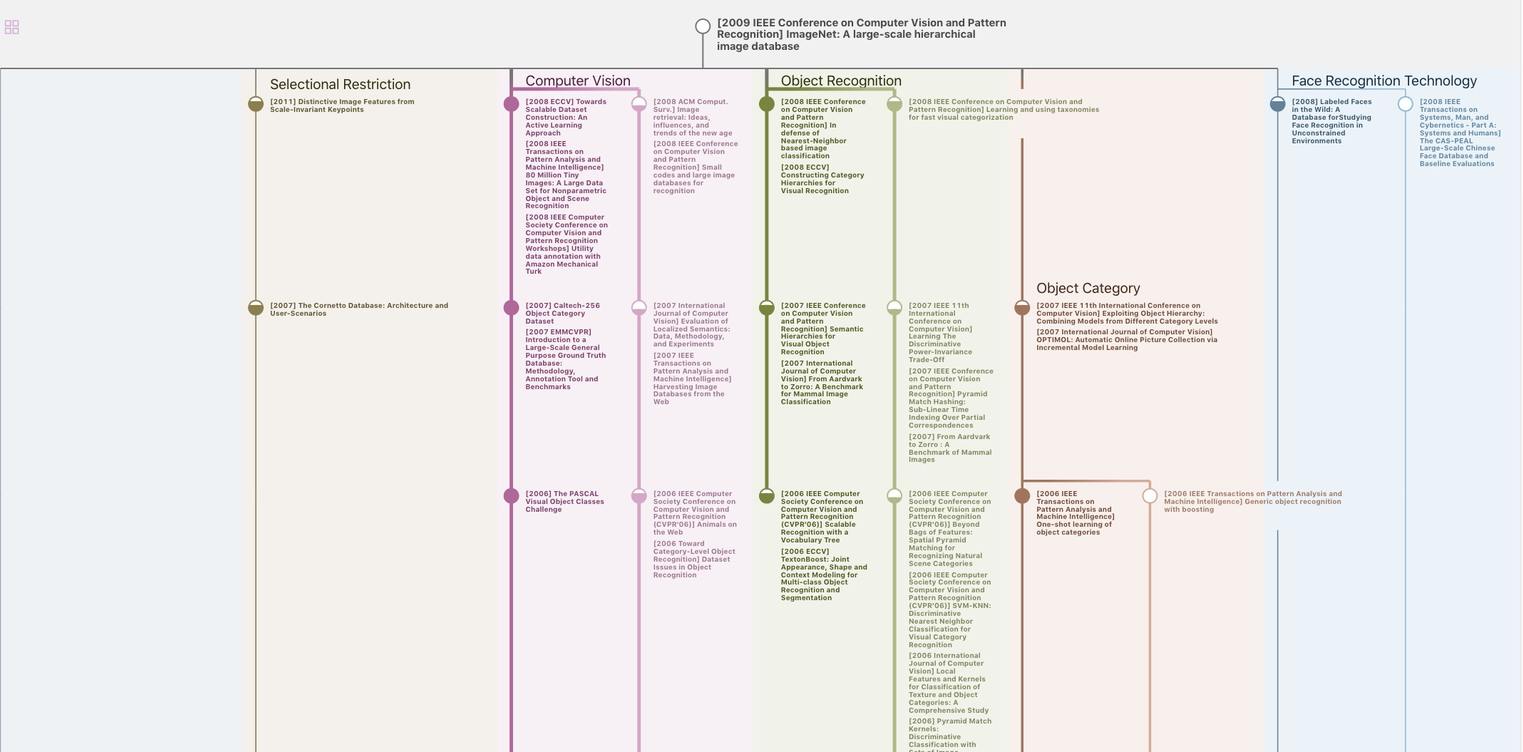
生成溯源树,研究论文发展脉络
Chat Paper
正在生成论文摘要