Convolutional Attention Network with Maximizing Mutual Information for Fine-Grained Image Classification.
SYMMETRY-BASEL(2020)
摘要
Fine-grained image classification has seen a great improvement benefiting from the advantages of deep learning techniques. Most fine-grained image classification methods focus on extracting discriminative features and combining the global features with the local ones. However, the accuracy is limited due to the inter-class similarity and the inner-class divergence as well as the lack of enough labelled images to train a deep network which can generalize to fine-grained classes. To deal with these problems, we develop an algorithm which combines Maximizing the Mutual Information (MMI) with the Learning Attention (LA). We make use of MMI to distill knowledge from the image pairs which contain the same object. Meanwhile we take advantage of the LA mechanism to find the salient region of the image to enhance the information distillation. Our model can extract more discriminative semantic features and improve the performance on fine-grained image classification. Our model has a symmetric structure, in which the paired images are inputted into the same network to extract the local and global features for the subsequent MMI and LA modules. We train the model by maximizing the mutual information and minimizing the cross-entropy stage by stage alternatively. Experiments show that our model can improve the performance of the fine-grained image classification effectively.
更多查看译文
关键词
image classification,mutual information,fine-grained,attention network
AI 理解论文
溯源树
样例
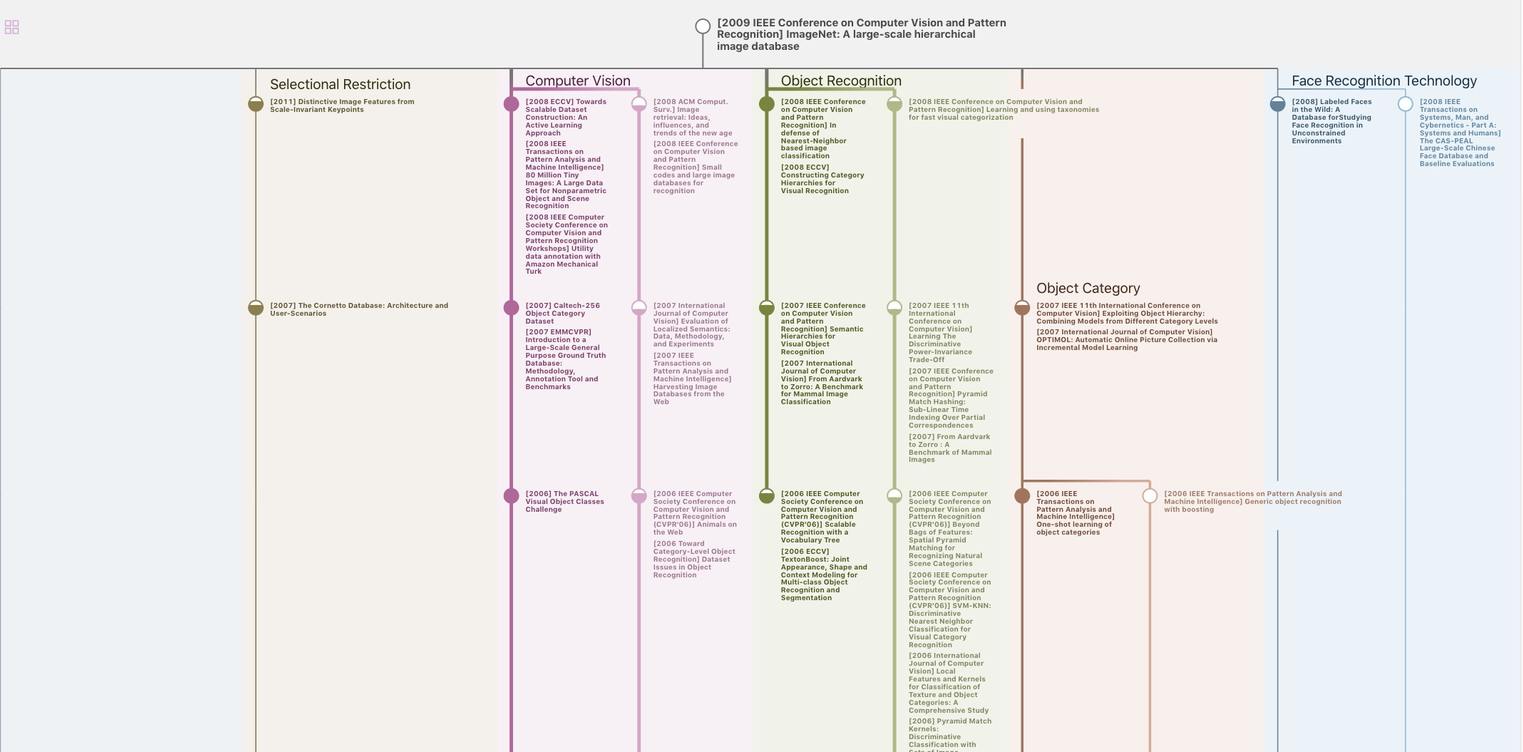
生成溯源树,研究论文发展脉络
Chat Paper
正在生成论文摘要