A dynamic inverse distance weighting-based local face descriptor
MULTIMEDIA TOOLS AND APPLICATIONS(2020)
摘要
This paper proposes a novel high-performance dynamic inverse distance weighting based local descriptor (DIDWLD) for facial recognition. Studies proposed thus far have focused on finding local descriptors that can represent the texture of the face best. However, the robustness of the descriptors against rotational variances and noise affects have been largely omitted. Thus, this study does not only concern with proposing a high-discriminative descriptor, but also a robust one against rotational changes and noise affects. DIDWLD mainly basis on Inverse Distance Weighting (IDW). That is, for each pixel in the image, a new descriptive value is calculated, taking into account the intensity values of the neighboring pixels and their distance to the reference pixels. A dynamic distance-decay parameter is applied throughout the image rather than keeping it uniform as done in ordinary IDW. The calculated descriptor is independent of the changes in the rotation. Because, when calculating the descriptor, the intensity values of the surrounding pixels with their distances to the reference pixel are taken into consideration, yet their directional relation to the reference pixel is ignored. Furthermore, when a pixel is suffered to noise, inherently, its neighboring pixels are also affected. Hence, by taking into account the effect of the surrounding pixels and also the original intensity value of the pixel, the degrading impact of noise on recognition performance is mitigated. The results of extensive simulations show the remarkable and competitive performance of the proposed method regarding recognition accuracy, and robustness against rotational variances and, noise effects.
更多查看译文
关键词
Facial recognition,Local descriptor,Inverse distance weighting,Classification,Rotation invariant
AI 理解论文
溯源树
样例
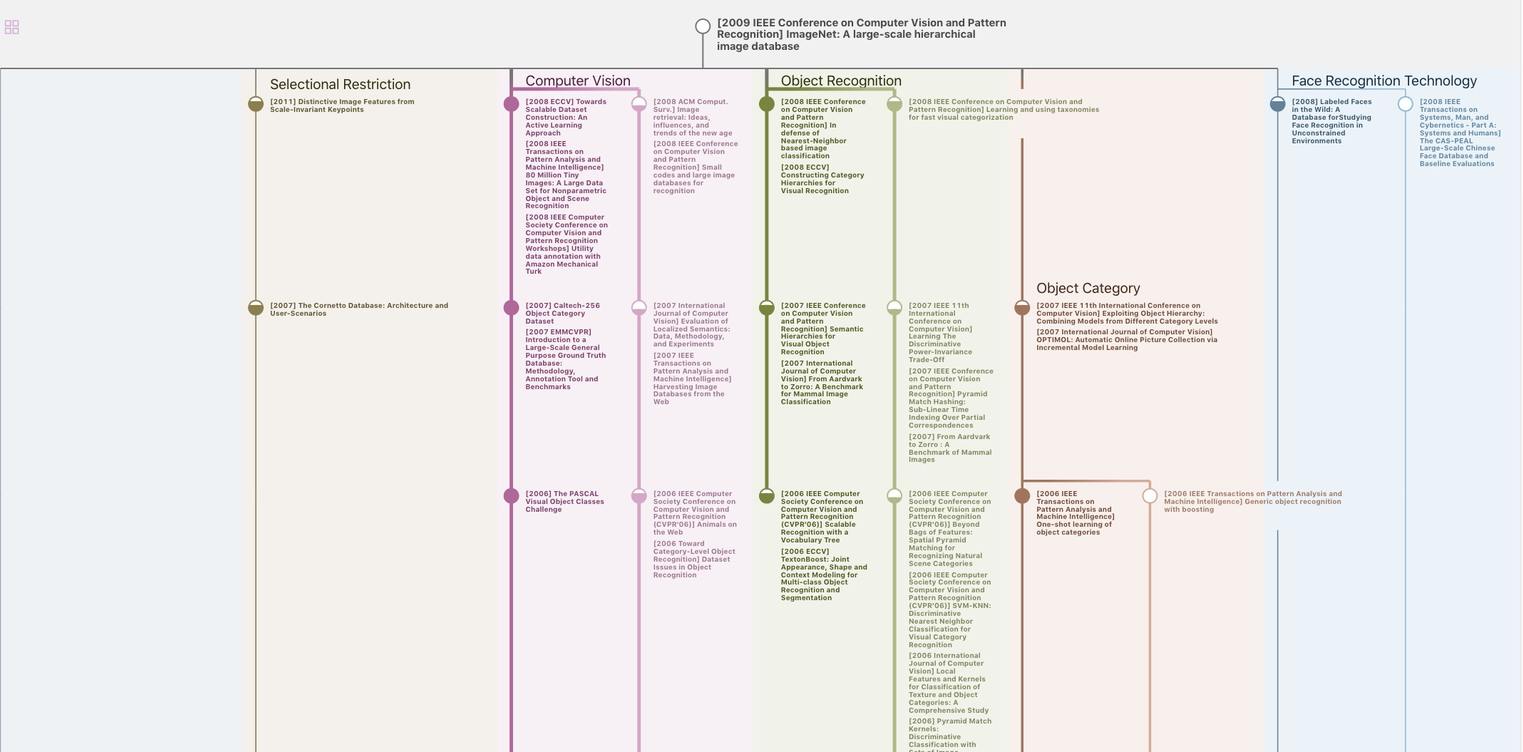
生成溯源树,研究论文发展脉络
Chat Paper
正在生成论文摘要