Combination of Temporal‐channels Correlation Information and Bilinear Feature for Action Recognition
IET computer vision(2020)
摘要
In this study, the authors focus on improving the spatio–temporal representation ability of three‐dimensional (3D) convolutional neural networks (CNNs) in the video domain. They observe two unfavourable issues: (i) the convolutional filters only dedicate to learning local representation along input channels. Also they treat channel‐wise features equally, without emphasising the important features; (ii) traditional global average pooling layer only captures first‐order statistics, ignoring finer detail features useful for classification. To mitigate these problems, they proposed two modules to boost 3D CNNs’ performance, which are temporal‐channel correlation (TCC) and bilinear pooling module. The TCC module can capture the information of inter‐channel correlations over the temporal domain. Moreover, the TCC module generates channel‐wise dependencies, which can adaptively re‐weight the channel‐wise features. Therefore, the network can focus on learning important features. With regards to the bilinear pooling module, it can capture more complex second‐order statistics in deep features and generate a second‐order classification vector. We can get more accurate classification results by combining the first‐order and second‐order classification vector. Extensive experiments show that adding our proposed modules to I3D network could consistently improve the performance and outperform the state‐of‐the‐art methods. The code and models are available at https://github.com/caijh33/I3D_TCC_Bilinear.
更多查看译文
关键词
learning (artificial intelligence),vectors,object recognition,image representation,image classification,stereo image processing,convolutional neural nets,correlation methods,higher order statistics,video signal processing,temporal‐channels correlation information,bilinear feature,three‐dimensional convolutional neural networks,video domain,3D CNN,convolutional filters,channel‐wise features,first‐order statistics,temporal‐channel correlation,bilinear pooling module,TCC module,channel‐wise dependencies,second‐order statistics,deep features,second‐order classification vector,first‐order classification vector,spatio–temporal representation,I3D network
AI 理解论文
溯源树
样例
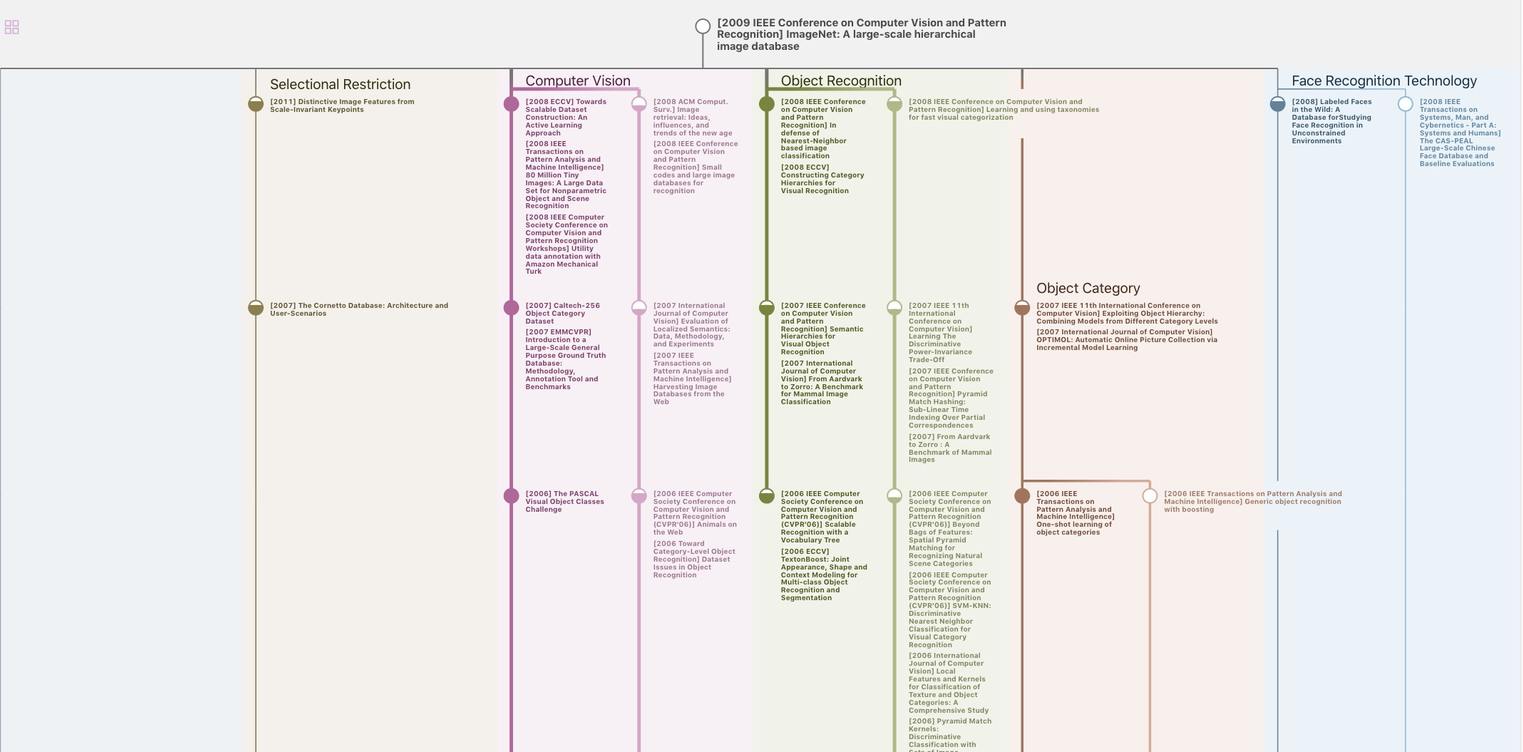
生成溯源树,研究论文发展脉络
Chat Paper
正在生成论文摘要