Creative And Diverse Artwork Generation Using Adversarial Networks
IET COMPUTER VISION(2020)
摘要
Existing style transfer methods have achieved great success in artwork generation by transferring artistic styles onto everyday photographs while keeping their contents unchanged. Despite this success, these methods have one inherent limitation: they cannot produce newly created image contents, lacking creativity and flexibility. On the other hand, generative adversarial networks (GANs) can synthesise images with new content, whereas cannot specify the artistic style of these images. The authors consider combining style transfer with convolutional GANs to generate more creative and diverse artworks. Instead of simply concatenating these two networks: the first for synthesising new content and the second for transferring artistic styles, which is inefficient and inconvenient, they design an end-to-end network called ArtistGAN to perform these two operations at the same time and achieve visually better results. Moreover, to generate images of higher quality, they propose the bi-discriminator GAN containing a pixel discriminator and a feature discriminator that constrain the generated image from pixel level and feature level, respectively. They conduct extensive experiments and comparisons to evaluate their methods quantitatively and qualitatively. The experimental results verify the effectiveness of their methods.
更多查看译文
关键词
art,image texture,unsupervised learning,convolutional neural nets,photography,creative artwork generation,diverse artwork generation,style transfer methods,artistic style,photographs,convolutional generative adversarial networks,end‐to‐end network,ArtistGAN,bidiscriminator GAN,convolutional GAN,pixel discriminator,feature discriminator,image generation
AI 理解论文
溯源树
样例
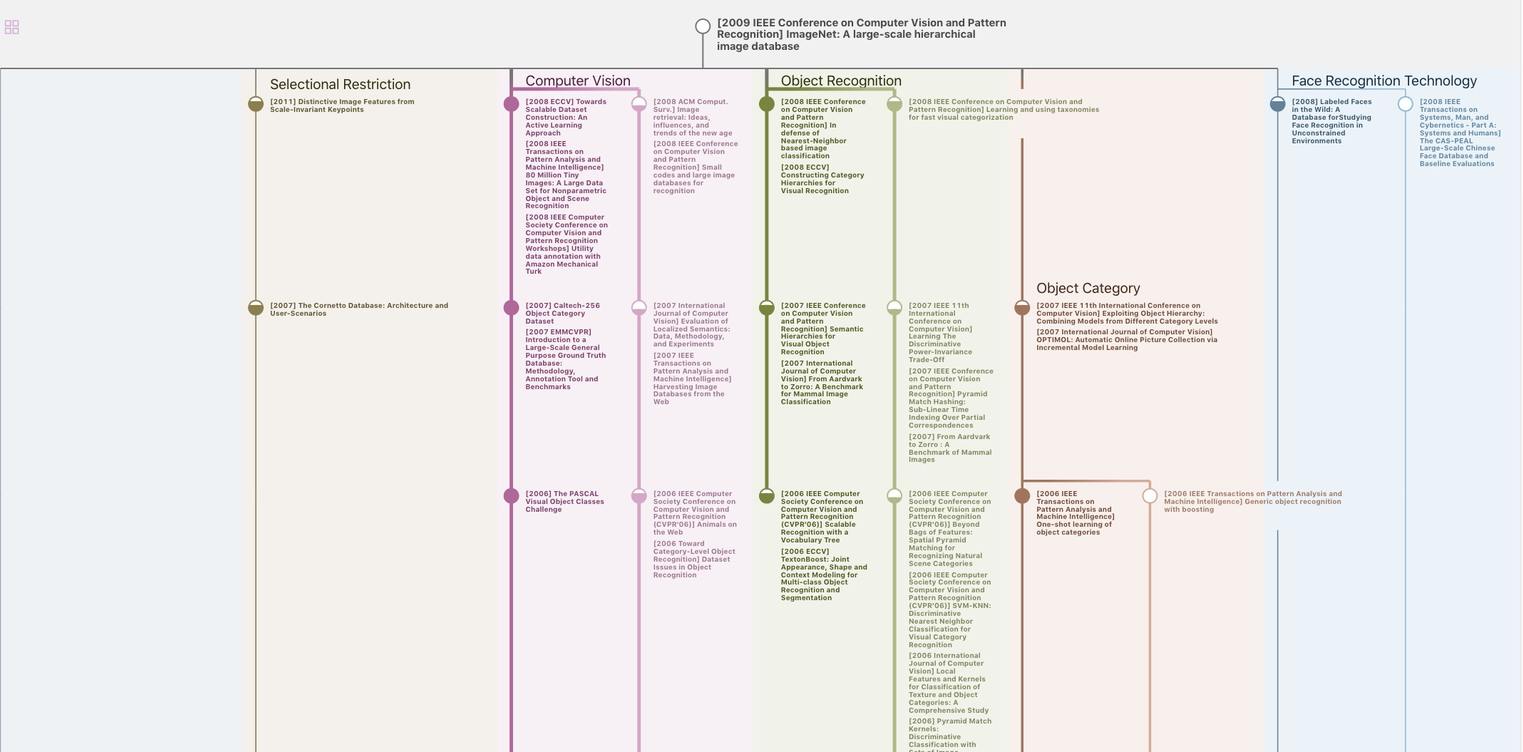
生成溯源树,研究论文发展脉络
Chat Paper
正在生成论文摘要