A Many-Objective Particle Swarm Optimization With Grid Dominance Ranking And Clustering
APPLIED SOFT COMPUTING(2020)
摘要
The existing MOPSOs face a great challenge in dealing with many-objective problems, due to the low discriminability of particles in many-objective spaces, which will affect the selection of leaders, thereby deteriorating the effectiveness of the algorithm. By using the property of domination, with the mapping of grid coordinates, this paper presents a framework of grid-based ranking scheme to sort the particles in grid space, which can select the swarm leaders (gbest, pbest) efficiently in MOPSO and enhance the convergence. Moreover, the clustering operation is conducted to update and maintain the external archive in the grid space to output a more diverse Pareto front. The performance of the proposed algorithm is verified by benchmark comparisons with several state-of-the-art MOPSOs and MOEAs. The DTLZ and WFG test suites with 4 to 10 objectives are used to assess the performance of our proposal. Experimental results demonstrate the promising performance of the proposed algorithm in terms of both optimization quality and convergence speed. Additionally, we discuss the influence of grid partition on efficiency, which verifies the effectiveness of the proposal from the other aspect. (C) 2020 Published by Elsevier B.V.
更多查看译文
关键词
Particle swarm optimization, MOPSO, Many-objective optimization, Swarm intelligence, Clustering
AI 理解论文
溯源树
样例
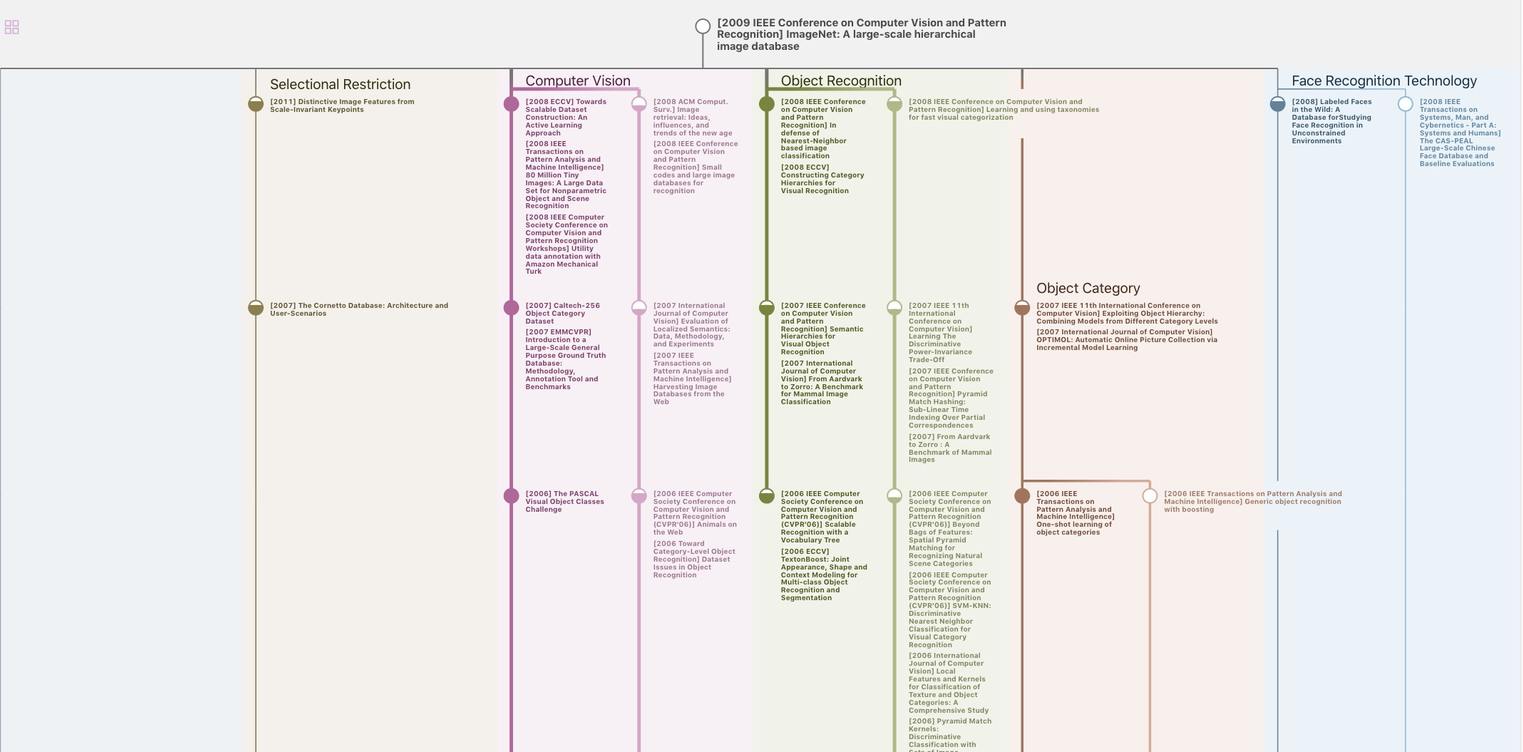
生成溯源树,研究论文发展脉络
Chat Paper
正在生成论文摘要