Aglnet: Towards Real-Time Semantic Segmentation Of Self-Driving Images Via Attention-Guided Lightweight Network
APPLIED SOFT COMPUTING(2020)
摘要
The extensive computational burden limits the usage of convolutional neural networks (CNNs) in edge devices for image semantic segmentation, which plays a significant role in many real-world applications, such as augmented reality, robotics, and self-driving. To address this problem, this paper presents an attention-guided lightweight network, namely AGLNet, which employs an encoder-decoder architecture for real-time semantic segmentation. Specifically, the encoder adopts a novel residual module to abstract feature representations, where two new operations, channel split and shuffle, are utilized to greatly reduce computation cost while maintaining higher segmentation accuracy. On the other hand, instead of using complicated dilated convolution and artificially designed architecture, two types of attention mechanism are subsequently employed in the decoder to upsample features to match input resolution. Specifically, a factorized attention pyramid module (FAPM) is used to explore hierarchical spatial attention from high-level output, still remaining fewer model parameters. To delineate object shapes and boundaries, a global attention upsample module (GAUM) is adopted as global guidance for high-level features. The comprehensive experiments demonstrate that our approach achieves state-of-the-art results in terms of speed and accuracy on three self-driving datasets: CityScapes, CamVid, and Mapillary Vistas. AGLNet achieves 71.3%, 69.4%, and 30.7% mean IoU on these datasets with only 1.12M model parameters. Our method also achieves 52 FPS, 90 FPS, and 53 FPS inference speed, respectively, using a single GTX 1080Ti GPU. Our code is open-source and available at https://github.com/xiaoyufenfei/Efficient-Segmentation-Networks. (C) 2020 Elsevier B.V. All rights reserved.
更多查看译文
关键词
Robot vision, Self-driving, Real-time semantic segmentation, Convolutional neural networks, Encoder-decoder networks
AI 理解论文
溯源树
样例
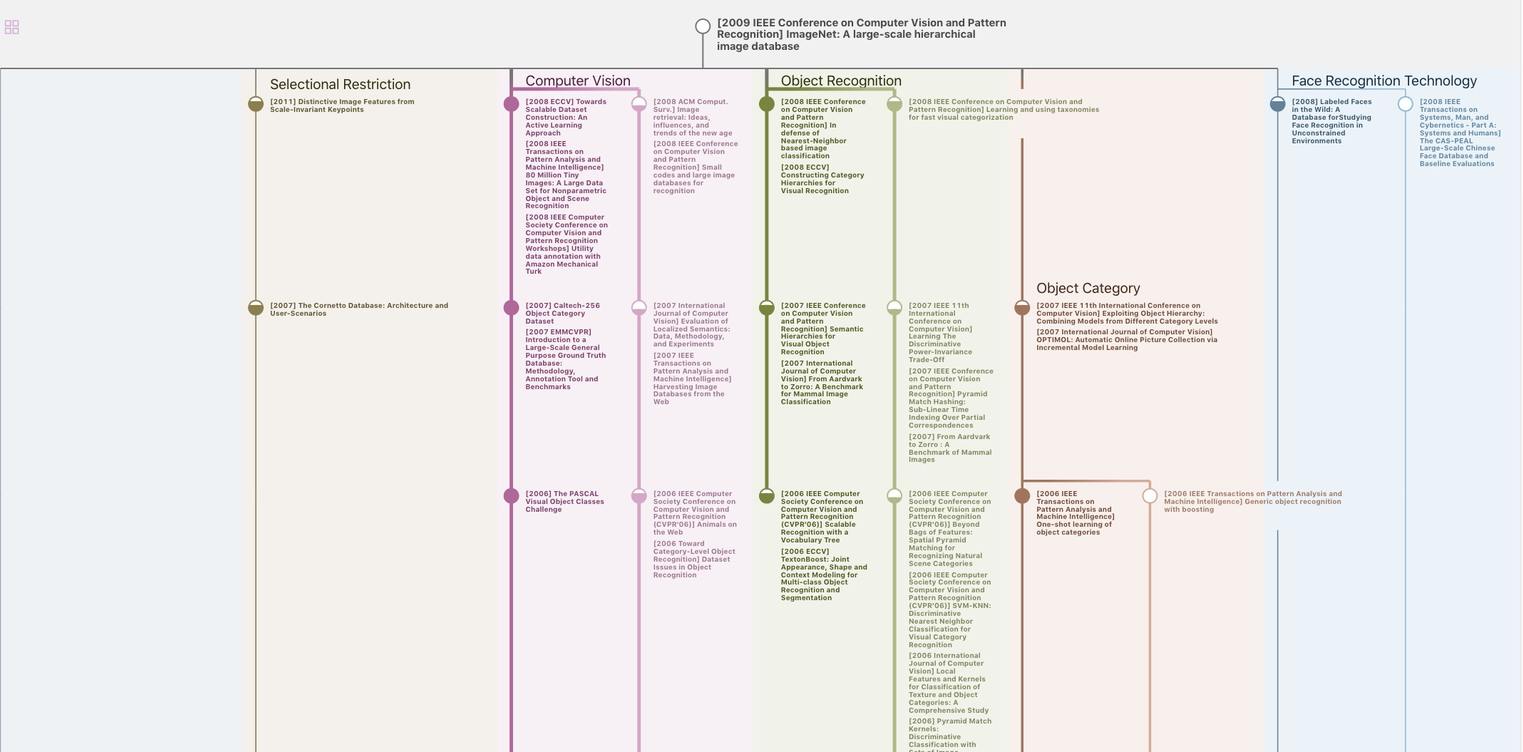
生成溯源树,研究论文发展脉络
Chat Paper
正在生成论文摘要