Condition-Transforming Variational Autoencoder for Generating Diverse Short Text Conversations
ACM Transactions on Asian and Low-Resource Language Information Processing(2020)
摘要
AbstractIn this article, conditional-transforming variational autoencoders (CTVAEs) are proposed for generating diverse short text conversations. In conditional variational autoencoders (CVAEs), the prior distribution of latent variable z follows a multivariate Gaussian distribution with mean and variance modulated by the input conditions. Previous work found that this distribution tended to become condition-independent in practical applications. Thus, this article designs CTVAEs to enhance the influence of conditions in CVAEs. In a CTVAE model, the latent variable z is sampled by performing a non-linear transformation on the combination of the input conditions and the samples from a condition-independent prior distribution N (0, I). In our experiments using a Chinese Sina Weibo dataset, the CTVAE model derives z samples for decoding with better condition-dependency than that of the CVAE model. The earth mover’s distance (EMD) between the distributions of the latent variable z at the training stage, and the testing stage is also reduced by using the CTVAE model. In subjective preference tests, our proposed CTVAE model performs significantly better than CVAE and sequence-to-sequence (Seq2Seq) models on generating diverse, informative, and topic-relevant responses.
更多查看译文
关键词
Neural network, variational autoencoder, conversation, text generation
AI 理解论文
溯源树
样例
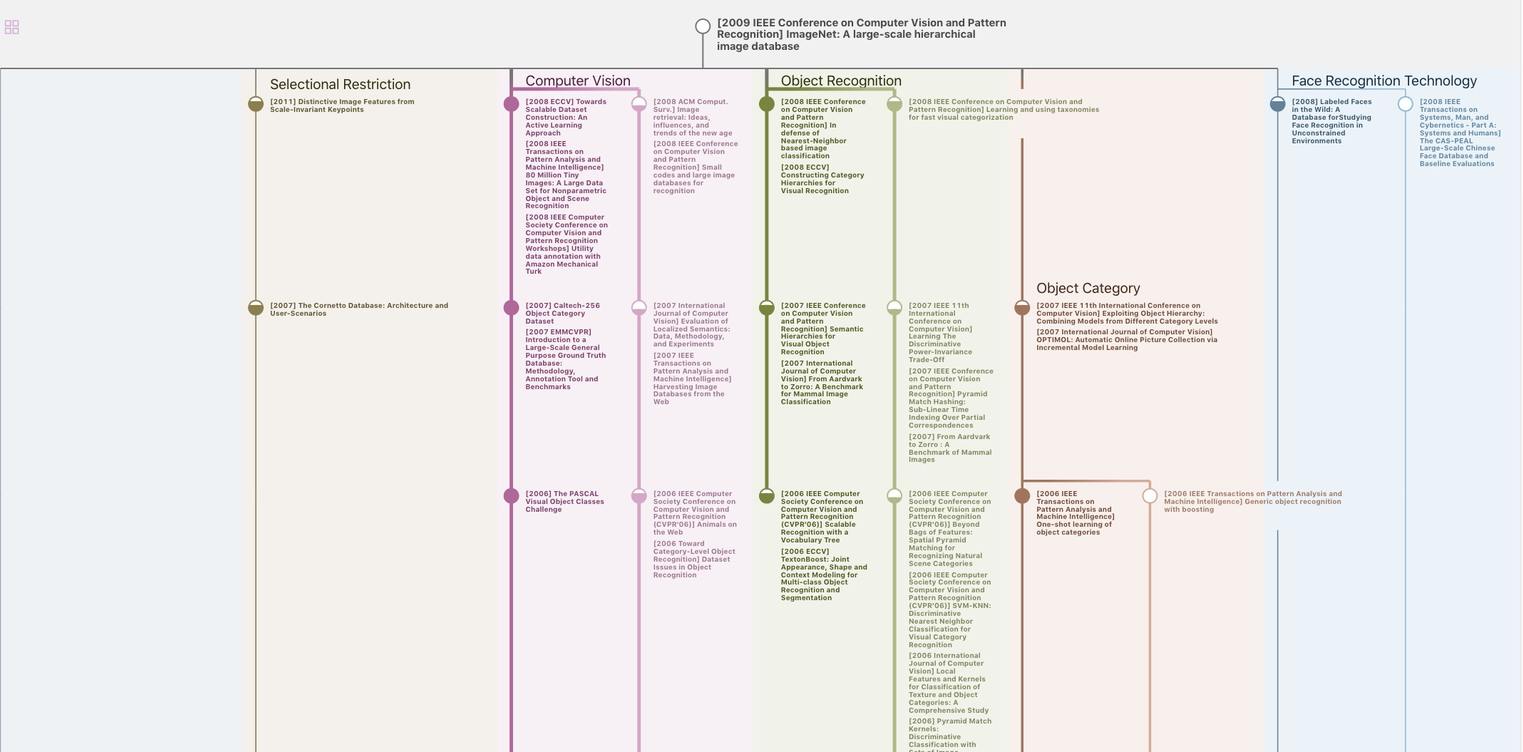
生成溯源树,研究论文发展脉络
Chat Paper
正在生成论文摘要