Optimized Spatiotemporal Data Scheduling Based on Maximum Flow for Multilevel Visualization Tasks.
ISPRS INTERNATIONAL JOURNAL OF GEO-INFORMATION(2020)
摘要
Massive spatiotemporal data scheduling in a cloud environment play a significant role in real-time visualization. Existing methods focus on preloading, prefetching, multithread processing and multilevel cache collaboration, which waste hardware resources and cannot fully meet the different scheduling requirements of diversified tasks. This paper proposes an optimized spatiotemporal data scheduling method based on maximum flow for multilevel visualization tasks. First, the spatiotemporal data scheduling framework is designed based on the analysis of three levels of visualization tasks. Second, the maximum flow model is introduced to construct the spatiotemporal data scheduling topological network, and the calculation algorithm of the maximum data flow is presented in detail. Third, according to the change in the data access hotspot, the adaptive caching algorithm and maximum flow model parameter switching strategy are devised to achieve task-driven spatiotemporal data optimization scheduling. Compared with two typical methods of first come first serve (FCFS) and priority scheduling algorithm (PSA) by simulating visualization tasks at three levels, the proposed maximum flow scheduling (MFS) method has been proven to be more flexible and efficient in adjusting each spatiotemporal data flow type as needed, and the method realizes spatiotemporal data flow global optimization under limited hardware resources in the cloud environment.
更多查看译文
关键词
scheduling optimization,maximum flow,spatiotemporal data,multilevel visualization tasks,cloud environment
AI 理解论文
溯源树
样例
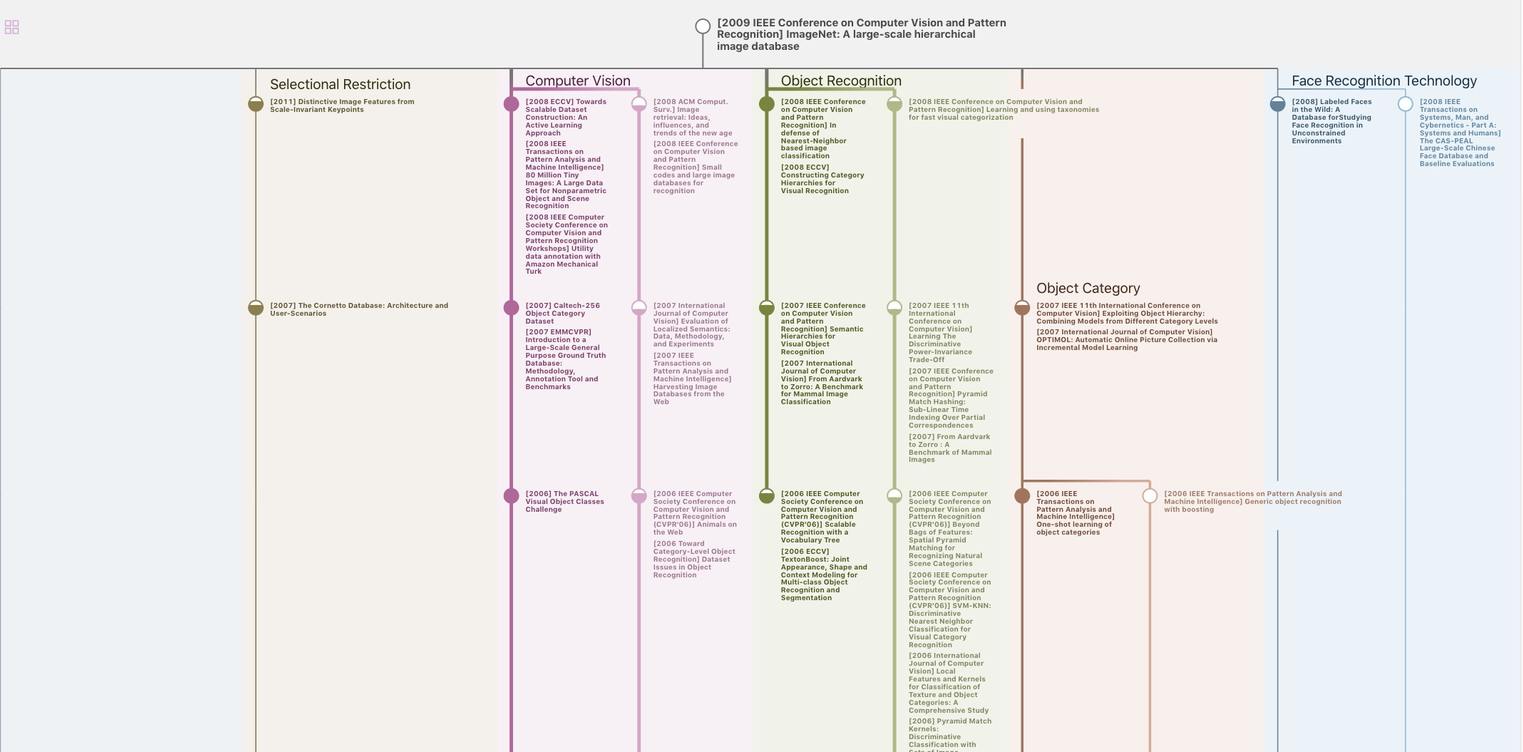
生成溯源树,研究论文发展脉络
Chat Paper
正在生成论文摘要