Machine learning techniques for credit risk evaluation: a systematic literature review
Journal of Banking and Financial Technology(2020)
摘要
Credit risk is the risk of financial loss when a borrower fails to meet the financial commitment. While there are many factors that constitute credit risk, due diligence while giving loan (credit scoring), continuous monitoring of customer payments and other behaviour patterns could reduce the probability of accumulating non-performing assets (NPA) and frauds. In the past few years, the quantum of NPAs and frauds have gone up significantly, and therefore it has become imperative that banks and financial institutions use robust mechanisms to predict the performance of loans. The past two decades has seen an immense growth in the area of artificial intelligence, most notably machine learning (ML) with improved access to internet, data, and compute. Whilst there are credit rating agencies and credit scoring companies that provide their analysis of a customer to banks on a fee, the researchers continue to explore various ML techniques to improve the accuracy level of credit risk evaluation. In this survey paper, we performed a systematic literature review on existing research methods and ML techniques for credit risk evaluation. We reviewed a total of 136 papers on credit risk evaluation published between 1993 and March 2019. We studied the implications of hyper parameters on ML techniques being used to evaluate credit risk and, analyzed the limitations of the current studies and research trends. We observed that Ensemble and Hybrid models with neural networks and SVM are being more adopted for credit scoring, NPA prediction and fraud detection. We also realized that lack of comprehensive public datasets continue to be an area of concern for researchers.
更多查看译文
关键词
Credit risk, Machine learning, Credit scoring, NPA prediction, Fraud detection
AI 理解论文
溯源树
样例
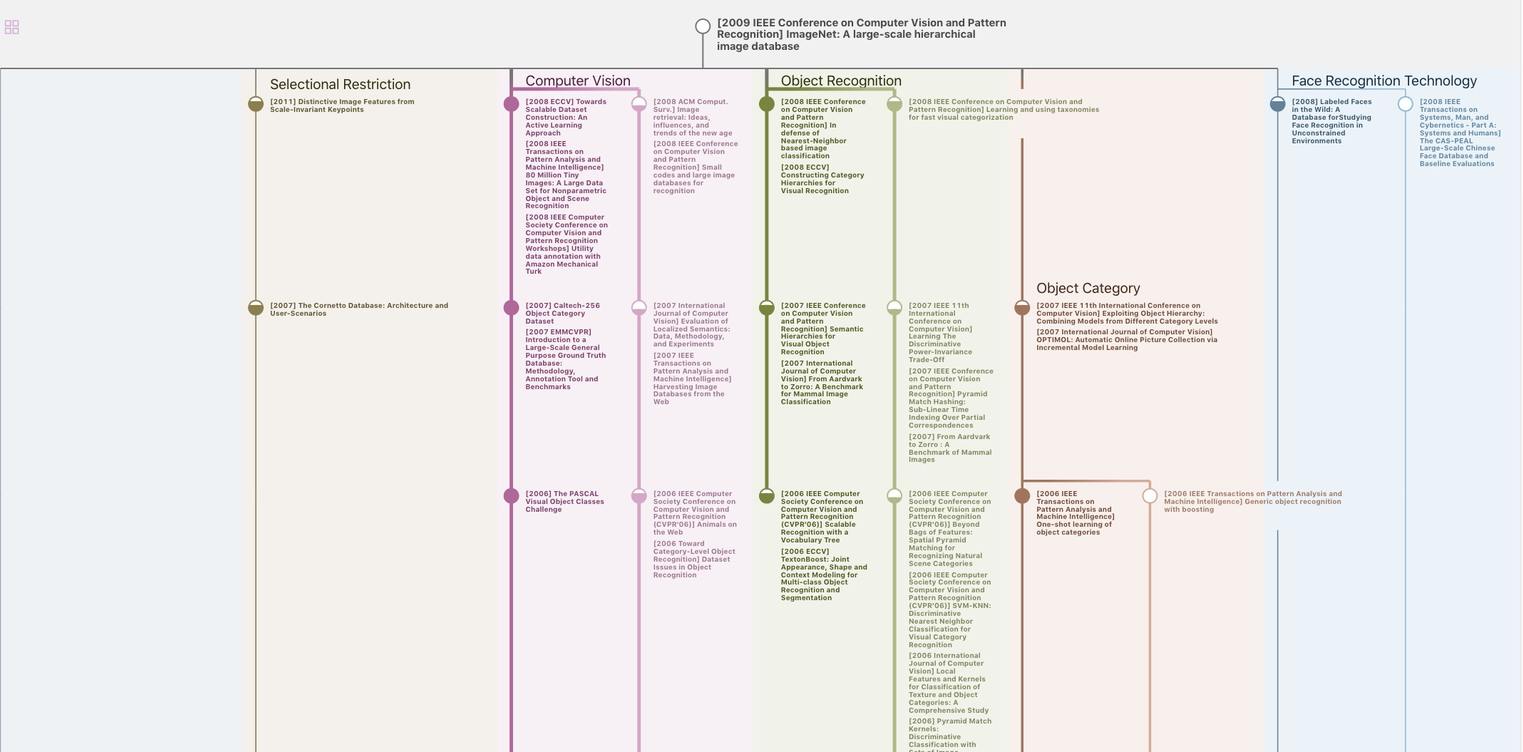
生成溯源树,研究论文发展脉络
Chat Paper
正在生成论文摘要