An Eeg Feature Extraction Method Based On Sparse Dictionary Self-Organizing Map For Event-Related Potential Recognition
ALGORITHMS(2020)
摘要
In the application of the brain-computer interface, feature extraction is an important part of Electroencephalography (EEG) signal classification. Using sparse modeling to extract EEG signal features is a common approach. However, the features extracted by common sparse decomposition methods are only of analytical meaning, and cannot relate to actual EEG waveforms, especially event-related potential waveforms. In this article, we propose a feature extraction method based on a self-organizing map of sparse dictionary atoms, which can aggregate event-related potential waveforms scattered inside an over-complete sparse dictionary into the code book of neurons in the self-organizing map network. Then, the cosine similarity between the EEG signal sample and the code vector is used as the classification feature. Compared with traditional feature extraction methods based on sparse decomposition, the classification features obtained by this method have more intuitive electrophysiological meaning. The experiment conducted on a public auditory event-related potential (ERP) brain-computer interface dataset showed that, after the self-organized mapping of dictionary atoms, the neurons' code vectors in the self-organized mapping network were remarkably similar to the ERP waveform obtained after superposition and averaging. The feature extracted by the proposed method used a smaller amount of data to obtain classification accuracy comparable to the traditional method.
更多查看译文
关键词
self-organizing map, feature extraction, event-related potential, brain-computer interface, sparse dictionary
AI 理解论文
溯源树
样例
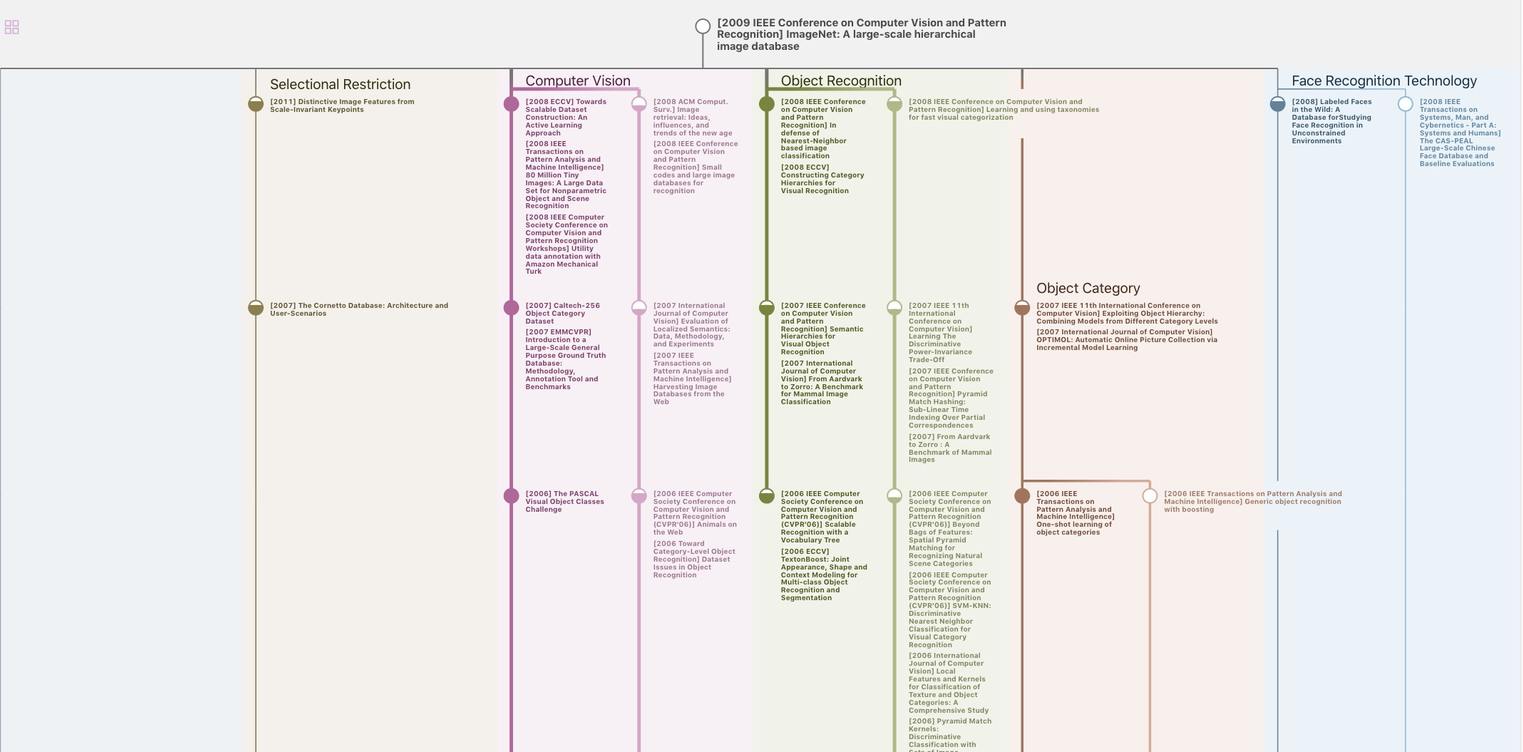
生成溯源树,研究论文发展脉络
Chat Paper
正在生成论文摘要