Flounder-Net: An efficient CNN for crowd counting by aerial photography
Neurocomputing(2021)
摘要
Crowd counting on aerial images using the embedded system is a challenging task, due to high-definition images, low computing power, and limited memory. To tackle this task, we propose an efficient deep learning model named Flounder-Net structured like a flounder. In the Flounder-Net, a novel interleaved group convolution is proposed to eliminate the redundancy of network, and a rapid shrink of feature maps is employed to tackle the high-resolution problem. Since we would like to investigate the case of online aerial surveillance, we use the embedded system of a drone to run our algorithm. We also use the vision system of this drone to collect a set of high-definition aerial photographs as a benchmark. Extensive experiments on existing datasets and our aerial dataset show that Flounder-Net achieves FCN-level accuracy with three types of photograph devices: handheld cameras, surveillance cameras, and drone-based cameras. Additionally, Flounder-Net has 17× fewer parameters and 20× faster speed than FCN and allows an input image with arbitrary sizes.
更多查看译文
关键词
Crowd counting,Embedded system,Deep learning
AI 理解论文
溯源树
样例
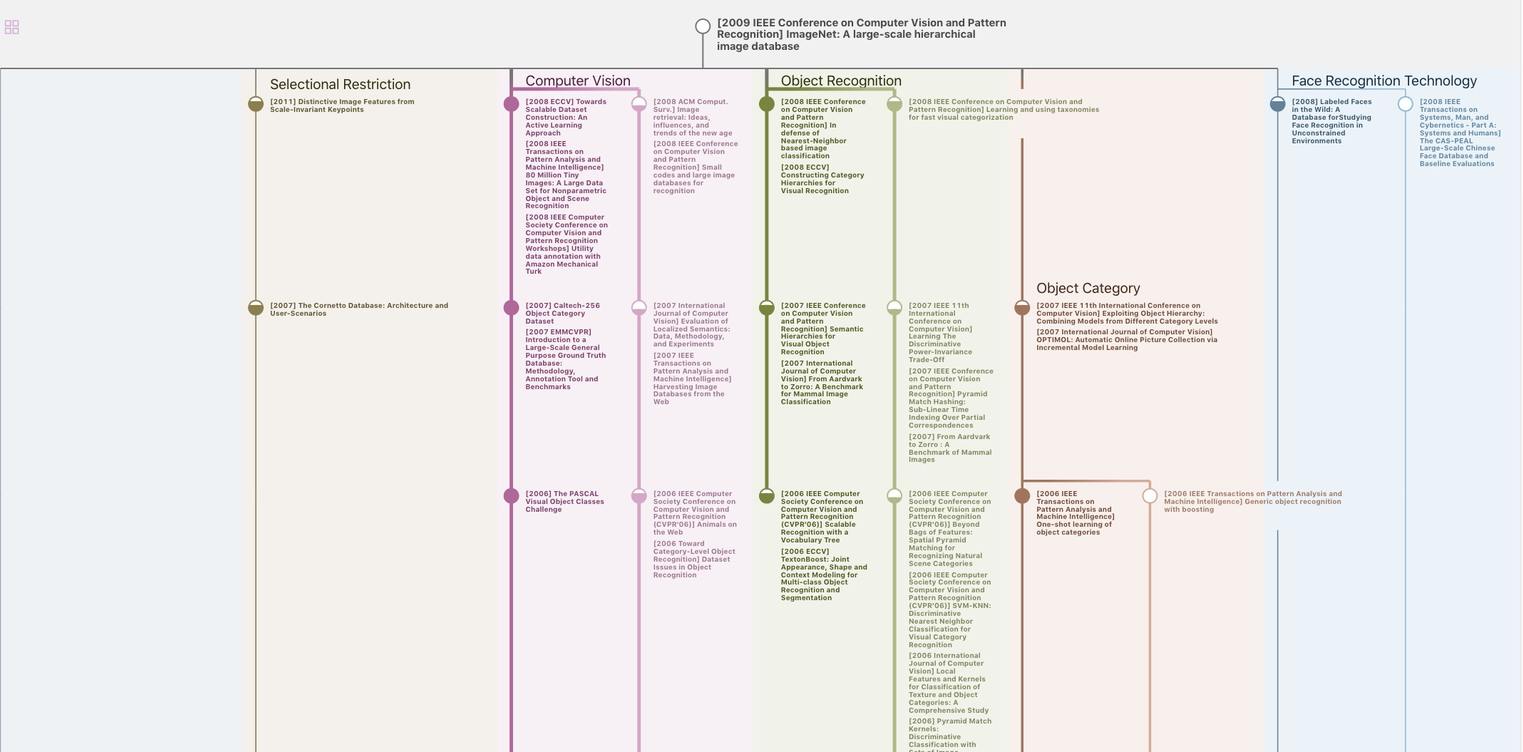
生成溯源树,研究论文发展脉络
Chat Paper
正在生成论文摘要