Skeleton edge motion networks for human action recognition
Neurocomputing(2021)
摘要
Human skeleton is receiving increasing attention from the community of human action recognition due to its robustness to complex image backgrounds. Previous methods usually utilize body joint-based representation, i.e., joint locations, while leaving edge-based movement poorly investigated. In this paper, we propose a new human action recognition method, skeleton edge motion networks (SEMN), to further explore the motion information of human body parts. Specifically, we address the movement of skeleton edge by using the angle changes of skeleton edge and the movement of the corresponding body joints. We then devise the proposed skeleton edge motion networks by stacking multiple spatial-temporal blocks to learn a robust deep representation from skeleton sequences. Furthermore, we propose a new progressive ranking loss to help the proposed skeleton edge motion networks maintain temporal order information in a self-supervised manner. Experimental results on five popular human action recognition datasets, PennAction, UTD-MHAD, NTU RGB+D, NTU RGB+D 120, and CSL, demonstrate the effectiveness of the proposed method.
更多查看译文
关键词
Skeleton edge motion,Spatial-temporal block,Deep learning,Human action recognition
AI 理解论文
溯源树
样例
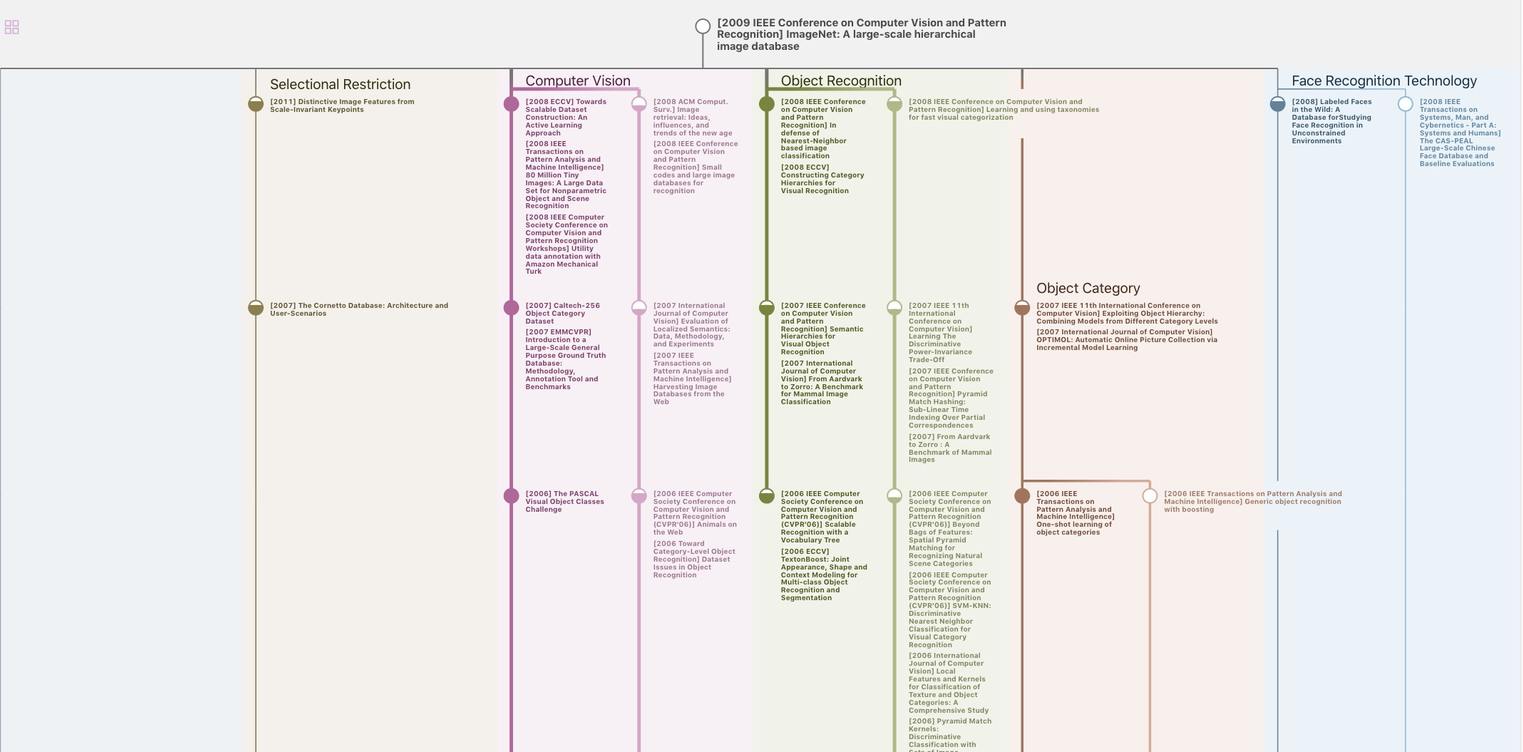
生成溯源树,研究论文发展脉络
Chat Paper
正在生成论文摘要