Learning cascaded convolutional networks for blind single image super-resolution
Neurocomputing(2020)
摘要
This paper studies the blind super-resolution of real low-quality and low-resolution (LR) images. Existing convolutional network (CNN) based approaches usually learn a single image super-resolution (SISR) model for a specific downsampler (e.g., bicubic downsampling, blurring followed by downsampling). The learned model, however, is tailored to the specific downsampler and fails to super-resolve real LR images which are degraded in more sophisticated and diverse manners. Moreover, the ground-truth high-resolution (HR) of real LR images are generally unavailable. Instead of learning from unpaired real LR-HR images or a specific downsampler, this paper learns blind SR network from a realistic, parametric degradation model by considering blurring, noise, downsampling, and even JPEG compression. In contrast to direct blind reconstruction of HR image, the proposed model adopts a cascaded architecture for noise estimation, blurring estimation, and non-blind SR, which can be jointly end-to-end learned from training data and benefit generalization ability. By taking the bicubicly upscaled LR image as input to non-blind SR, the proposed method can present a single unified model for blind SR with any upscaling factors and varying degradation parameters. Experimental results show that the proposed method performs favorably on synthetic and real LR images.
更多查看译文
关键词
Image restoration,Blind image super-resolution
AI 理解论文
溯源树
样例
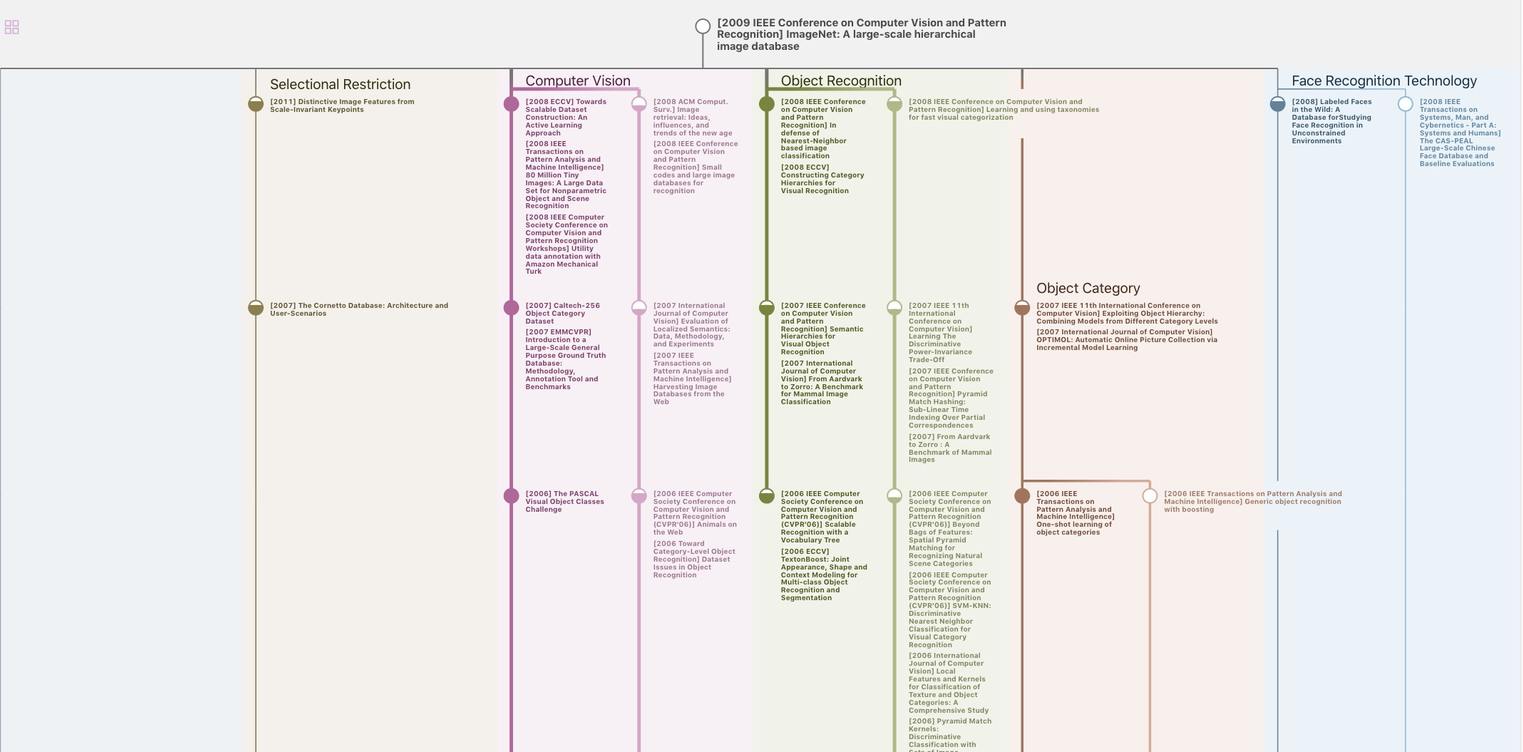
生成溯源树,研究论文发展脉络
Chat Paper
正在生成论文摘要