Bias decomposition and estimator performance in respondent-driven sampling.
SOCIAL NETWORKS(2021)
摘要
Respondent-Driven Sampling (RDS) is a method of network sampling that is used to sample hard-to-reach populations. The resultant sample is non-random, but different weighting methods can account for the over sampling of (1) high-degree individuals and (2) homophilous groups that recruit members more effectively. While accounting for degree-bias is almost universally agreed upon, accounting for recruitment-bias has been debated as it can further increase estimate variance without substantially reducing bias. Simulation-based research has examined which weighting procedures perform best given underlying population network structures, group recruitment differences, and sampling processes. Yet, in the field, analysts do not have a priori knowledge of the network they are sampling. We show that the RDS sample data itself can determine whether degree-based estimator is sufficient. Formulas derived from the decomposition of a 'dual-component' estimator can approximate the 'recruitment component' (RC) and 'degree component' (DC) of a sample's bias. Simulations show that RC and DC values can predict the performance of different classes of estimators. Samples with extreme 'RC' values, a consequence of network homophily and differential recruitment, are better served by a classical estimator. The use of sample data to improve estimator selection is a promising innovation for RDS, as population network features that should guide estimator selection are typically unknown.
更多查看译文
关键词
Respondent-driven sampling,Network sampling
AI 理解论文
溯源树
样例
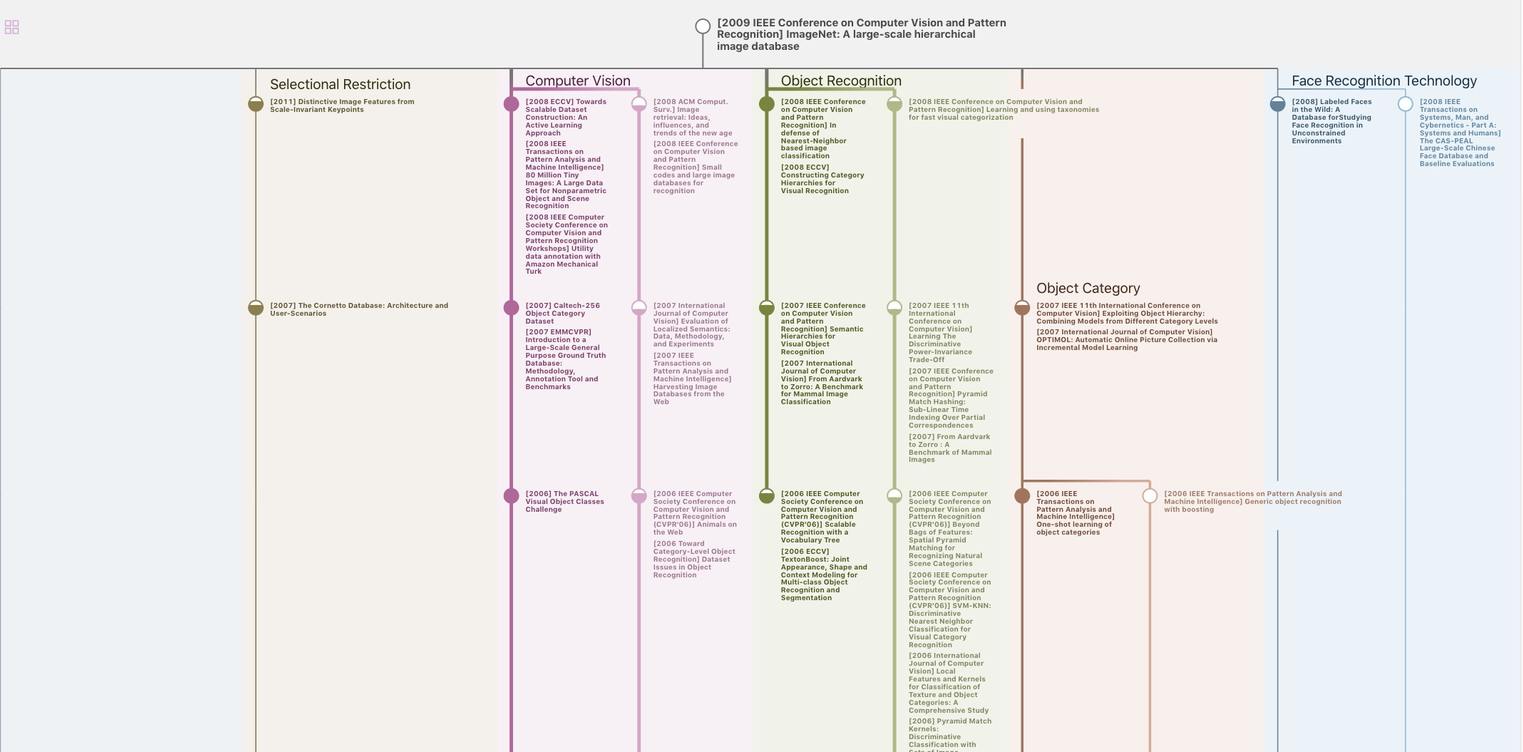
生成溯源树,研究论文发展脉络
Chat Paper
正在生成论文摘要