Policy-Based Branch-And-Bound For Infinite-Horizon Multi-Model Markov Decision Processes
COMPUTERS & OPERATIONS RESEARCH(2021)
摘要
Markov decision processes (MDPs) are models for sequential decision-making that inform decision making in many fields, including healthcare, manufacturing, and others. However, the optimal policy for an MDP may be sensitive to the reward and transition parameters which are often uncertain because parameters are typically estimated from data or rely on expert opinion. To address parameter uncertainty in MDPs, it has been proposed that multiple models of the parameters be incorporated into the solution process, but solving these problems can be computationally challenging. In this article, we propose a policy based branch-and-bound approach that leverages the structure of these problems and numerically compare several important algorithmic designs. We demonstrate that our approach outperforms existing methods on test cases from the literature including randomly generated MDPs, a machine maintenance MDP, and an MDP for medical decision making. (C) 2020 Elsevier Ltd. All rights reserved.
更多查看译文
关键词
Markov decision processes, Parameter uncertainty, Branch-and-bound
AI 理解论文
溯源树
样例
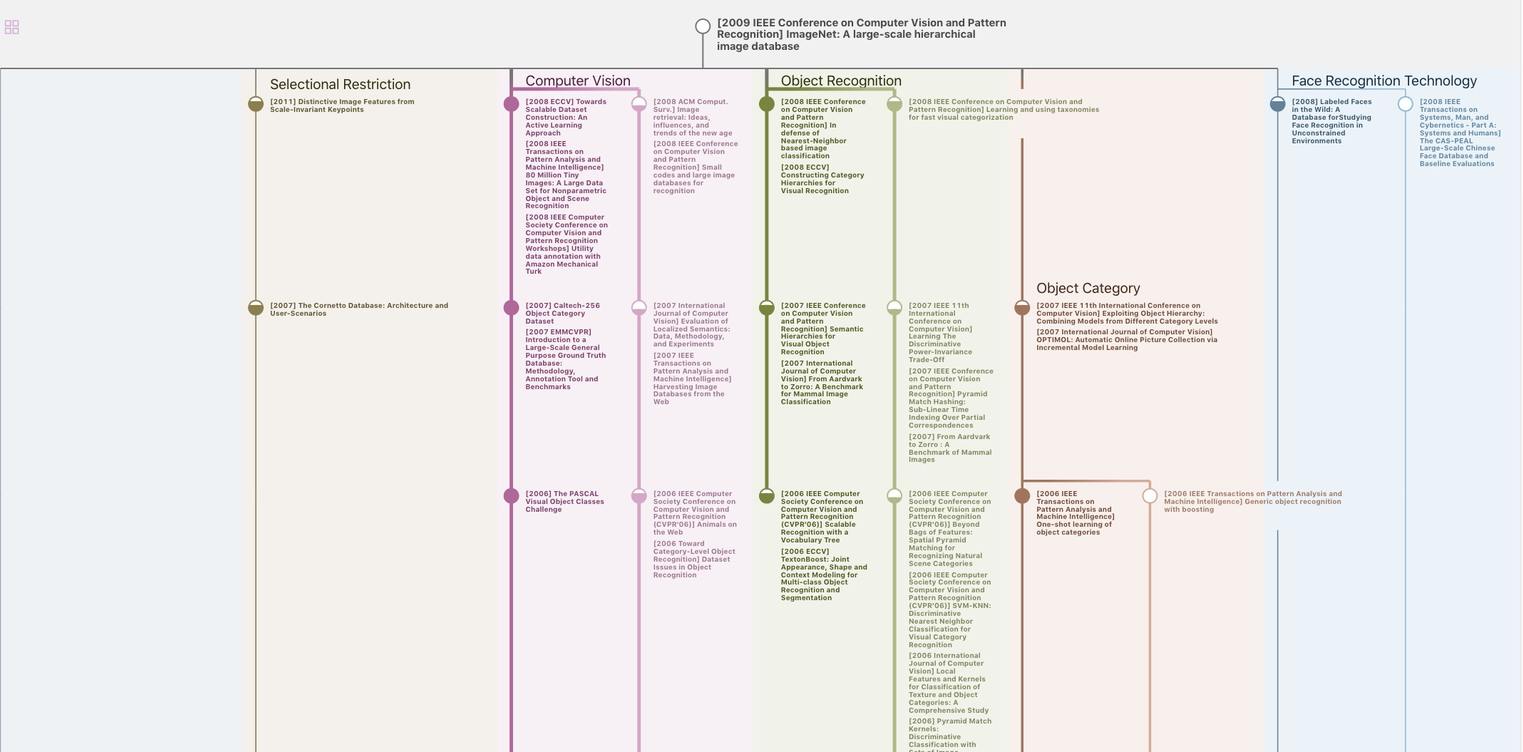
生成溯源树,研究论文发展脉络
Chat Paper
正在生成论文摘要