Open Set Domain Adaptation by Extreme Value Theory
arxiv(2020)
摘要
Common domain adaptation techniques assume that the source domain and the target domain share an identical label space, which is problematic since when target samples are unlabeled we have no knowledge on whether the two domains share the same label space. When this is not the case, the existing methods fail to perform well because the additional unknown classes are also matched with the source domain during adaptation. In this paper, we tackle the open set domain adaptation problem under the assumption that the source and the target label spaces only partially overlap, and the task becomes when the unknown classes exist, how to detect the target unknown classes and avoid aligning them with the source domain. We propose to utilize an instance-level reweighting strategy for domain adaptation where the weights indicate the likelihood of a sample belonging to known classes and to model the tail of the entropy distribution with Extreme Value Theory for unknown class detection. Experiments on conventional domain adaptation datasets show that the proposed method outperforms the state-of-the-art models.
更多查看译文
关键词
set domain adaptation
AI 理解论文
溯源树
样例
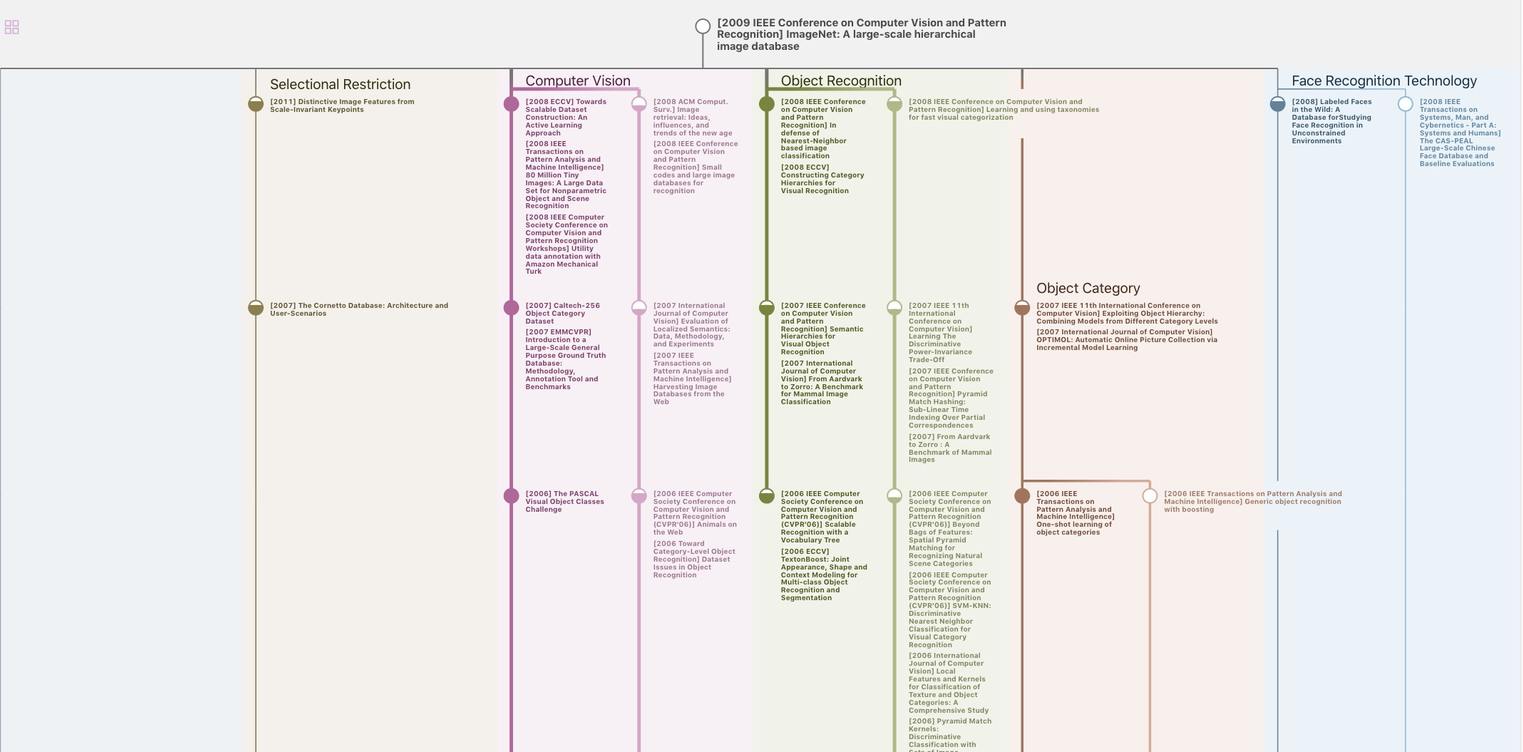
生成溯源树,研究论文发展脉络
Chat Paper
正在生成论文摘要