CoachNet: An Adversarial Sampling Approach for Reinforcement Learning
arxiv(2021)
摘要
Despite the recent successes of reinforcement learning in games and robotics, it is yet to become broadly practical. Sample efficiency and unreliable performance in rare but challenging scenarios are two of the major obstacles. Drawing inspiration from the effectiveness of deliberate practice for achieving expert-level human performance, we propose a new adversarial sampling approach guided by a failure predictor named "CoachNet". CoachNet is trained online along with the agent to predict the probability of failure. This probability is then used in a stochastic sampling process to guide the agent to more challenging episodes. This way, instead of wasting time on scenarios that the agent has already mastered, training is focused on the agent's "weak spots". We present the design of CoachNet, explain its underlying principles, and empirically demonstrate its effectiveness in improving sample efficiency and test-time robustness in common continuous control tasks.
更多查看译文
关键词
adversarial sampling approach,reinforcement learning,coachnet
AI 理解论文
溯源树
样例
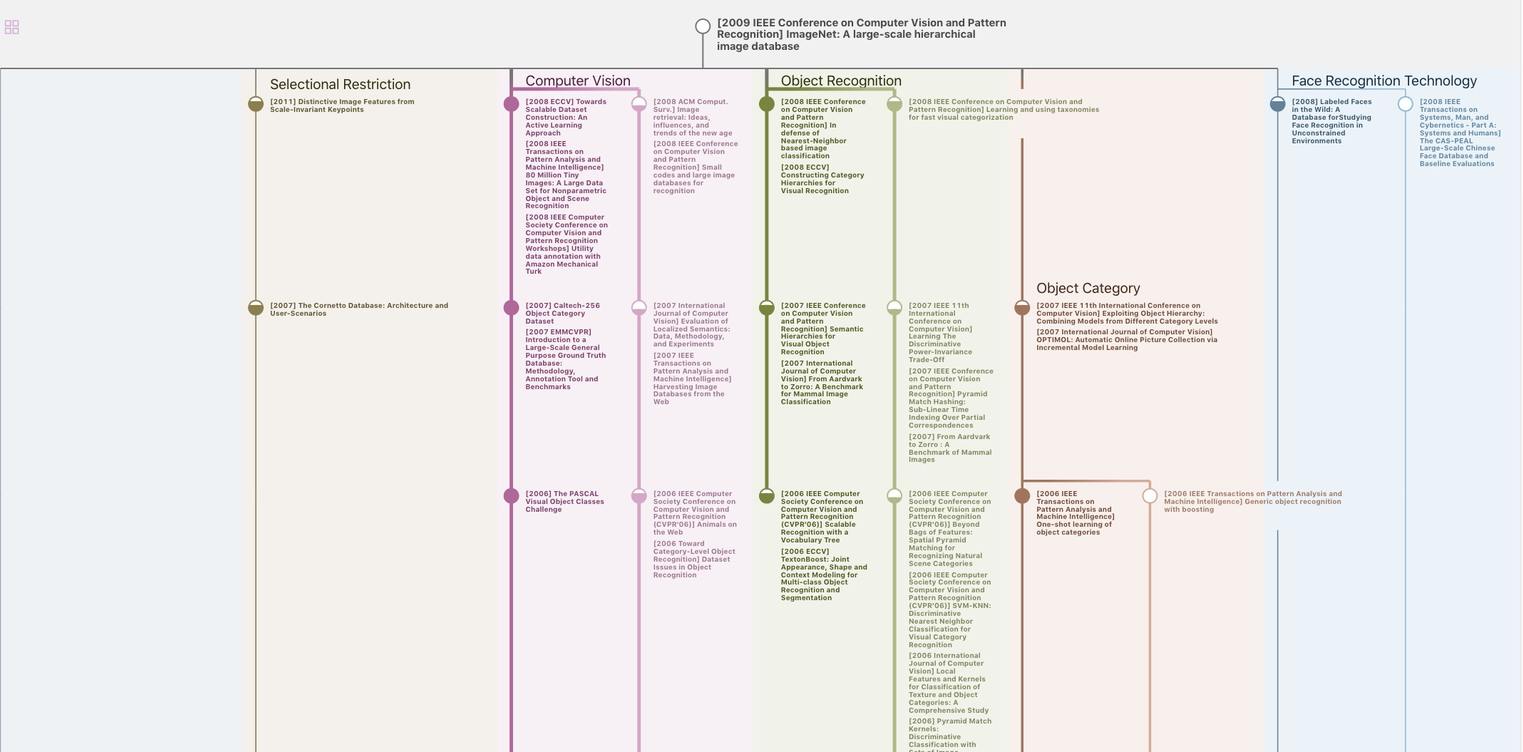
生成溯源树,研究论文发展脉络
Chat Paper
正在生成论文摘要