The Effect of Prior Lipschitz Continuity on the Adversarial Robustness of Bayesian Neural Networks
arxiv(2021)
摘要
It is desirable, and often a necessity, for machine learning models to be robust against adversarial attacks. This is particularly true for Bayesian models, as they are well-suited for safety-critical applications, in which adversarial attacks can have catastrophic outcomes. In this work, we take a deeper look at the adversarial robustness of Bayesian Neural Networks (BNNs). In particular, we consider whether the adversarial robustness of a BNN can be increased by model choices, particularly the Lipschitz continuity induced by the prior. Conducting in-depth analysis on the case of i.i.d., zero-mean Gaussian priors and posteriors approximated via mean-field variational inference, we find evidence that adversarial robustness is indeed sensitive to the prior variance.
更多查看译文
关键词
adversarial robustness,bayesian neural networks,prior lipschitz continuity,neural networks
AI 理解论文
溯源树
样例
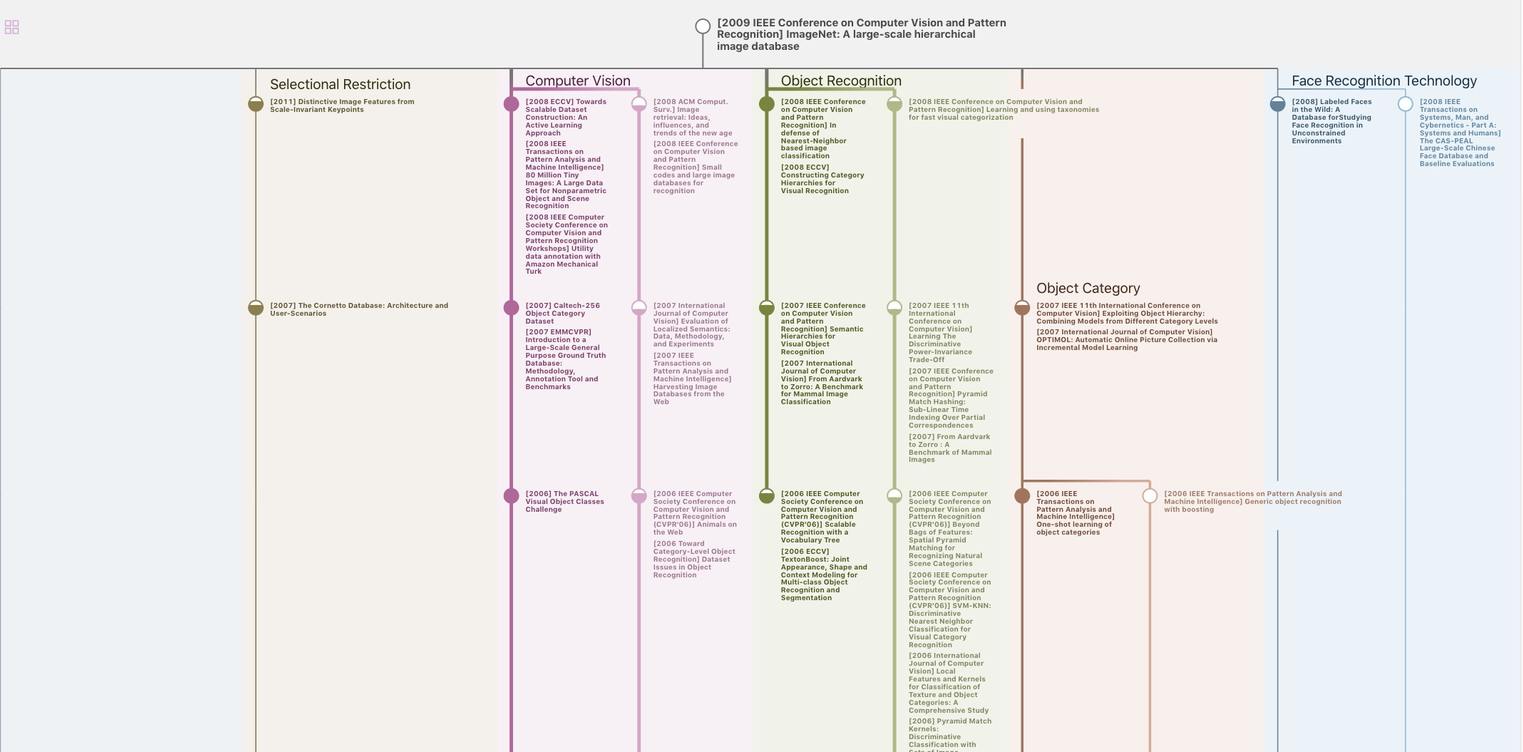
生成溯源树,研究论文发展脉络
Chat Paper
正在生成论文摘要