Cohort analytics: efficiency and applicability
The VLDB Journal(2020)
摘要
The abundant availability of health-care data calls for effective analysis methods to help medical experts gain a better understanding of their patients and their health. The focus of existing work has been largely on prediction. In this paper, we introduce Core , a framework for cohort “representation” and “exploration.” Our contributions are twofold: First, we formalize cohort representation as the problem of aggregating the trajectories of its patients. This problem is challenging because cohorts often consist of hundreds of patients who underwent medical actions of various types at different points in time. We prove that producing a representative cohort trajectory is NP-complete with a reduction in the multiple sequence alignment problem. We propose a heuristic that extends the Needleman–Wunsch algorithm for sequence matching to handle temporal sequences. To further improve cohort representation efficiency, we introduce “trajectory families” and “stratified sampling.” Our second contribution is formalizing the problem of cohort exploration as finding a set of cohorts that are similar to a cohort of interest and that maximize entropy. This problem is challenging because the potential number of similar cohorts is huge. We prove NP-completeness with a reduction in the maximum edge subgraph problem. To address complexity, we develop a multi-staged approach based on limiting the search space to “contrast cohorts.” To speed up the computation of cohort similarity, we use “event sets” that are inspired from the double dictionary encoding proposed for keyword search. Moreover, we explore the usefulness and efficiency of Core using an extensive set of qualitative and quantitative experiments on two real health-care datasets. In a user study with medical experts, we show that Core reduces time-to-insight from hours to seconds and helps them find better insights than baseline approaches. Moreover, we show that the obtained cohort representations offer the right trade-off between quality and performance. We study the benefits of trajectory families and stratified sampling for cohort representation and show their applicability on large and heterogeneous cohorts. We also show the benefit of event sets for cohort exploration in providing interactive performance.
更多查看译文
关键词
Health-care data analysis, Cohort analytics, Cohort representation, Cohort exploration
AI 理解论文
溯源树
样例
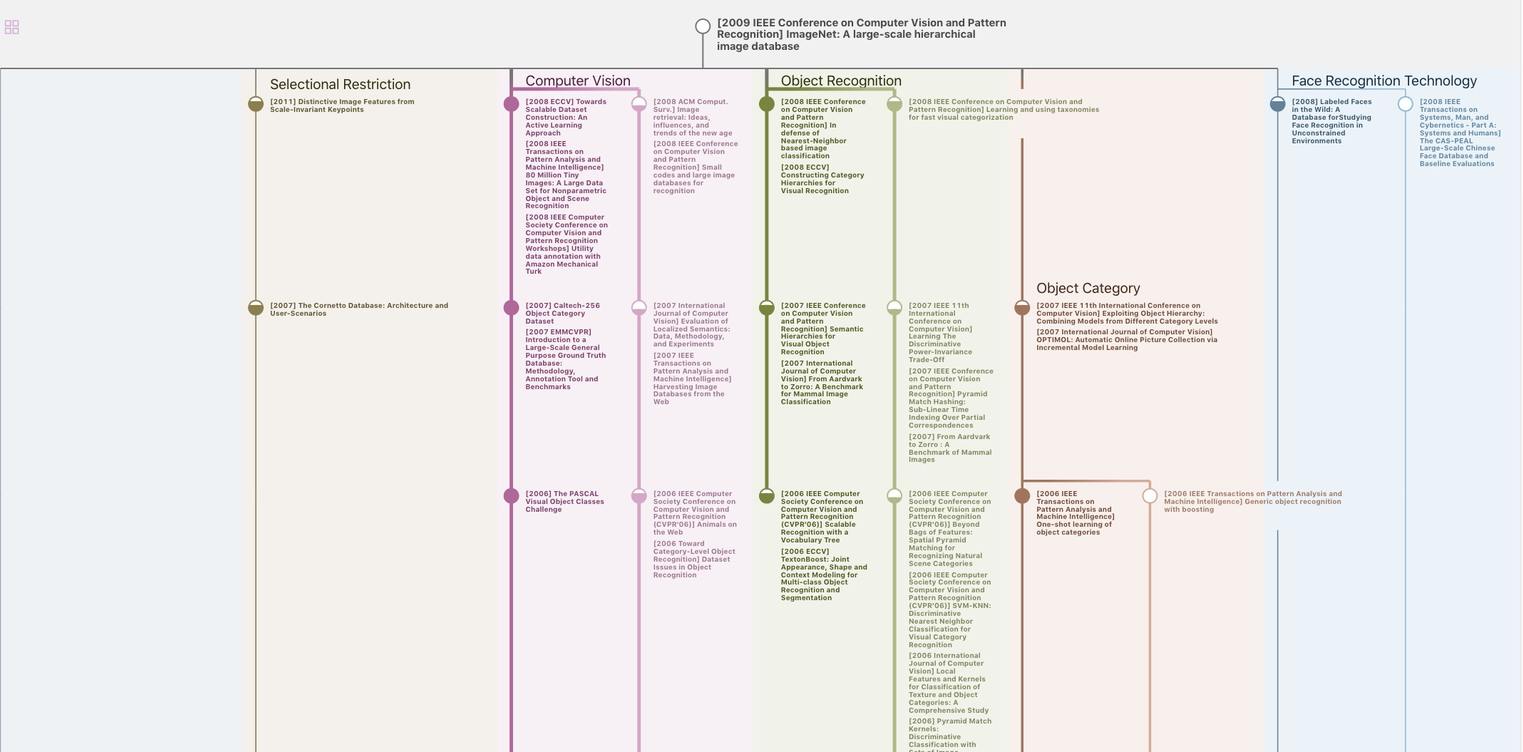
生成溯源树,研究论文发展脉络
Chat Paper
正在生成论文摘要