Automatic Classification Of Sleep Stage From An Ecg Signal Using A Gated-Recurrent Unit
INTERNATIONAL JOURNAL OF FUZZY LOGIC AND INTELLIGENT SYSTEMS(2020)
摘要
A healthy sleep structure is clinically very important for overall health. The sleep structure can be represented by the percentage of different sleep stages during the total sleep time. In this study, we proposed a method for automatic classification of sleep stages from an electrocardiogram (ECG) signal using a gated-recurrent unit (GRU). The proposed method performed multiclass classification for three-class sleep stages such as awake, light, and deep sleep. A deep structured GRU was used in the proposed method, which is a common recurrent neural network. The proposed deep learning (SleepGRU) model consists of a 5-layer GRU and is optimized by batch-normalization, dropout, and Adam update rules. The ECG signal was recorded during nocturnal polysomnography from 112 subjects, and was normalized and segmented into units of 30-second duration. To train and evaluate the proposed method, the training set consisted of 80,316 segments from 89 subjects, and the test set used 20,079 segments from 23 subjects. We achieved good performances with an overall accuracy of 80.43% and F1-score of 80.07% for the test set. The proposed method can be an alternative and useful tool for sleep monitoring and sleep screening, which have previously been manually evaluated by a sleep technician or sleep expert.
更多查看译文
关键词
Sleep stage classification, Gated-recurrent unit, Deep learning, Electrocardiogram
AI 理解论文
溯源树
样例
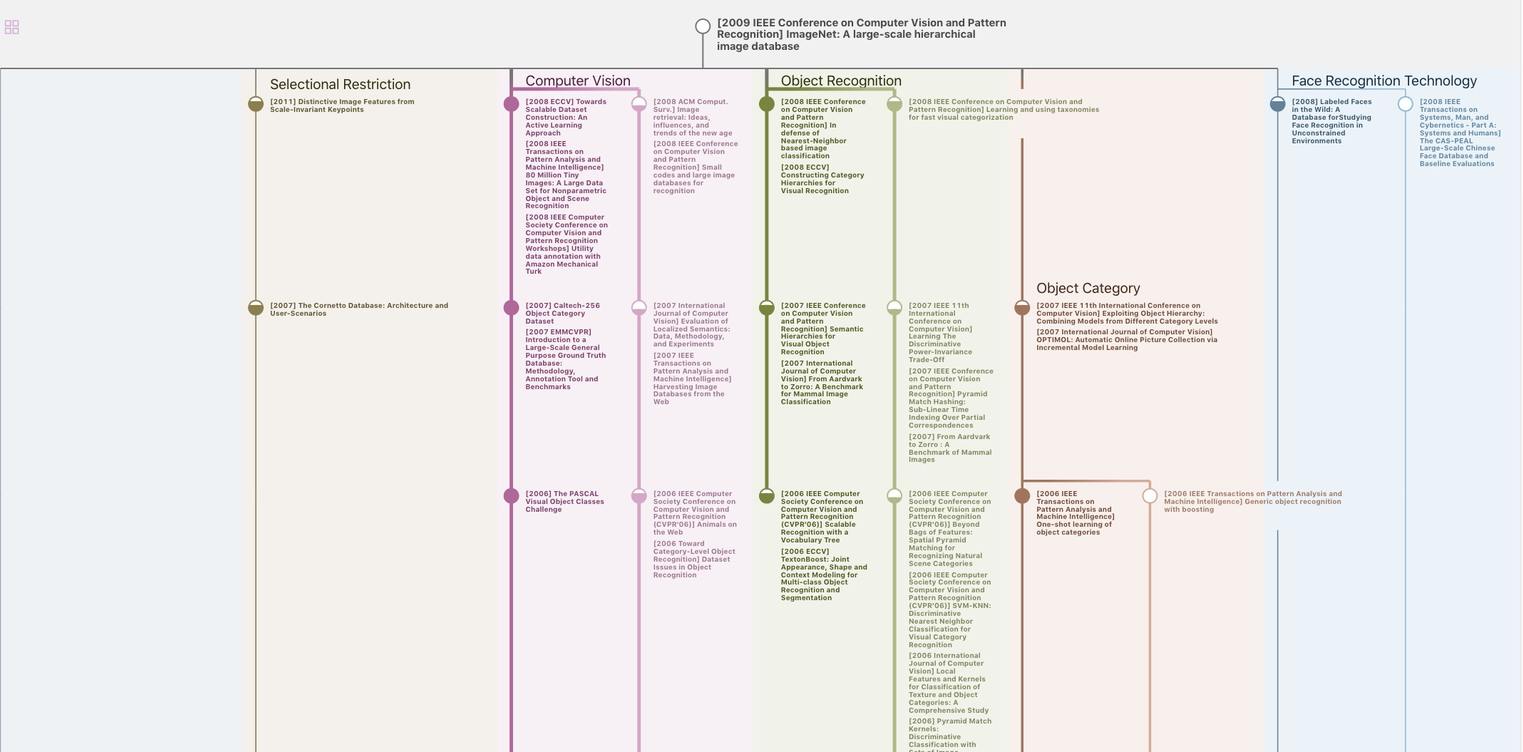
生成溯源树,研究论文发展脉络
Chat Paper
正在生成论文摘要